Research Group for Neuromorphic Computing
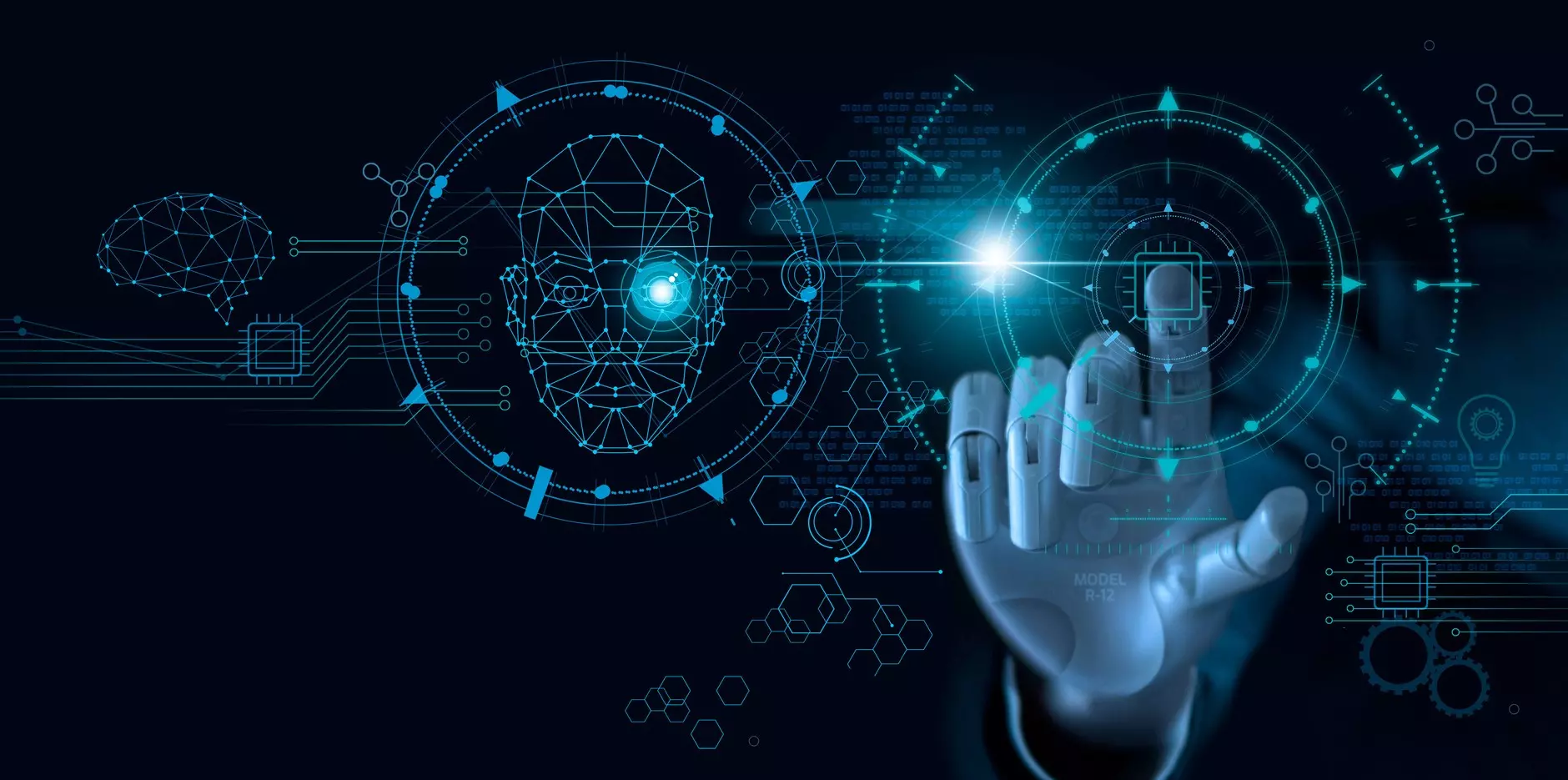
Introduction
The Research Group for Neuromorphic Computing develops advanced neural-network based algorithms, software libraries, and systems with the new generation of computing chips – brain-inspired neuromorphic sensing and computing hardware. We focus on perception, motion planning, and control for robotic actuators with applications in life sciences: healthcare, agriculture, food processing, and smart environments. We follow a human-centered design approach to develop new generation of physical AI systems that are power-efficient, adaptive, and safe.
Expertise
- Neuromorphic computing hardware and algorithms
- Event-based vision
- Robotics: Motion planning, control, SLAM
- Efficient machine learning and AI
- Dynamical systems, cognitive architectures
Areas of application
- Assistive robotics in healthcare, agriculture, food processing, smart environments
- Machine vision in healthcare, agriculture, food processing, smart environments
- Continual learning and adaptive systems
- Robot safety, human-robot interaction
Collaborations and partners
Engagement in teaching
Our research group includes teaching engagements at BSc and MSc level as well as in continuing education.
Our Team
-
ZHAW Life Sciences und Facility Management
FS Cognitive Computing in Life Sciences
Schloss
8820 Wädenswil -
ZHAW Life Sciences und Facility Management
FG Neuromorphic Computing Group
Schloss 1
8820 Wädenswil -
ZHAW Life Sciences und Facility Management
FG Neuromorphic Computing Group
-
ZHAW Life Sciences und Facility Management
FS Cognitive Computing in Life Sciences
-
ZHAW Life Sciences und Facility Management
FG Neuromorphic Computing Group
Current projects
- Vorherige Seite
- Seite 01
- Seite 02
- Seite 03
- Seite 04
- Seite 05
- …
- Seite 25
- Nächste Seite
-
Reinforcement Learning Analysis Framework
Das Ziel dieses Projekts ist es, einen Rahmen zu schaffen, der die Entwicklung von RL-Lösungen für reale Anwendungen erleichtert. Dies ist notwendig, da sich die akademische Literatur in der Regel auf spezifische Algorithmen konzentriert und sich die Ansätze für verschiedene Regionen im hochkomplexen RL-Problemraum ...
-
Optimierung der Pflanzengesundheit im Indoor-Farming mit Hilfe von Verstärkendem Lernen
Die Forschungsfrage, mit der wir uns in diesem Projekt befassen, lautet: "Wie können wir den Wachstumspfad von Indoor-Pflanzen automatisch optimieren?". Um dieses Ziel zu erreichen, entwickeln wir neue Algorithmen zur Messung von Pflanzengesundheit und -wachstum. Anschliessend evaluieren wir auf Verstärkendem Lernen ...