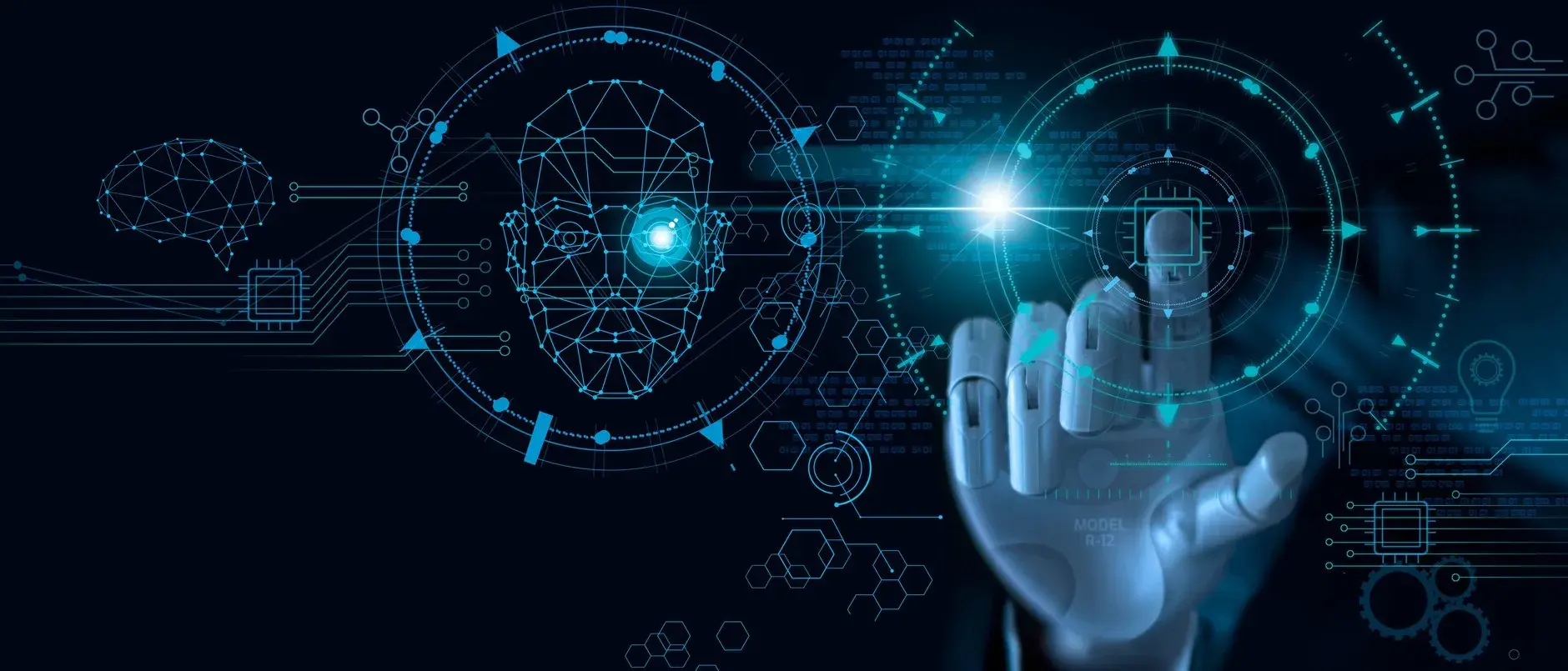
Introduction
The Research Group for Neuromorphic Computing develops advanced neural-network based algorithms, software libraries, and systems with the new generation of computing chips – brain-inspired neuromorphic sensing and computing hardware. We focus on perception, motion planning, and control for robotic actuators with applications in life sciences: healthcare, agriculture, food processing, and smart environments. We follow a human-centered design approach to develop new generation of physical AI systems that are power-efficient, adaptive, and safe.
Expertise
- Neuromorphic computing hardware and algorithms
- Event-based vision
- Robotics: Motion planning, control, SLAM
- Efficient machine learning and AI
- Dynamical systems, cognitive architectures
Areas of application
- Assistive robotics in healthcare, agriculture, food processing, smart environments
- Machine vision in healthcare, agriculture, food processing, smart environments
- Continual learning and adaptive systems
- Robot safety, human-robot interaction
Collaborations and partners
Engagement in teaching
Our research group includes teaching engagements at BSc and MSc level as well as in continuing education.
Our Team
-
ZHAW School of Life Sciences and Facility Management
Schloss
8820 Wädenswil -
ZHAW School of Life Sciences and Facility Management
Schloss 1
8820 Wädenswil -
ZHAW School of Life Sciences and Facility Management
-
ZHAW School of Life Sciences and Facility Management
Schloss
8820 Wädenswil -
ZHAW School of Life Sciences and Facility Management
Schloss
8820 Wädenswil -
ZHAW School of Life Sciences and Facility Management
Schloss
8820 Wädenswil
Current projects
Unfortunately, no list of projects can be displayed here at the moment. Until the list is available again, the project search on the ZHAW homepage can be used.