Artificial Intelligence in Industry and Finance
We would like to welcome you to the «3rd COST Conference on Mathematics for Industry in Switzerland» on Artificial Intelligence in Industry and Finance, hosted by the Institute of Applied Mathematics and Physics and the Institute of Data Analysis and Process Design at the Zurich University of Applied Sciences ZHAW in Winterthur, Switzerland.
Artificial Intelligence in Industry and Finance (3rd European COST Conference on Mathematics for Industry in Switzerland)
September 6, 2018, 9:00-17:30 - ZHAW Winterthur, Technikumstrasse 71, 8401 Winterthur
Information at a glance
- Conference Date: Thursday, September 6, 2018
- Conference Location: ZHAW Winterthur, Technikumstrasse 71, 8401 Winterthur, Eulachpassage (TN)
- Conference Topics: Artificial Intelligence in Industry and Finance
- Conference Flyer(PDF 1,9 MB)
- Related Conferences: "Perspektiven mit Industrie 4.0", "Smart Maintenance"
- Related Journal: "Frontiers in Artificial Intelligence"(PDF 266,5 KB)
Aim of the conference
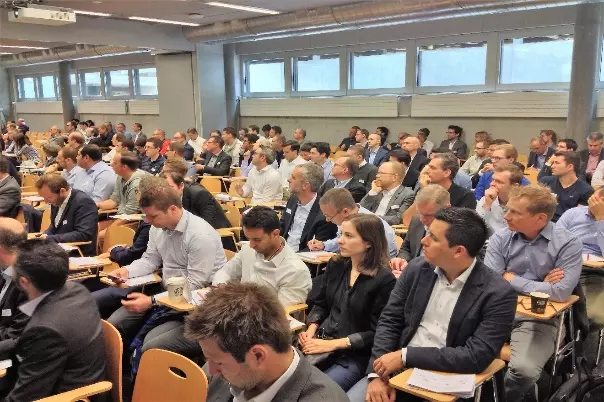
The aim of this conference is to bring together European academics, young researchers, students and industrial practitioners to discuss the application of Artificial Intelligence in Industry and Finance within the COST research network.
This conference is supported by COST Action TD1409, Mathematics for Industry Network (MI-NET) and by the Swiss State Secretariat for Education, Research and Innovation SERI. COST is the longest-running European framework supporting transnational cooperation among researchers, engineers and scholars across Europe. The 1st COST Conference on this topic was held on September 15, 2016, and the 2nd COST Conference was held on September 7, 2017. The 4th Conference on will be held on September 5, 2019.
All lectures are open to the public. Registration is now open.
Scientific topics
- Artificial Intelligence challenges for European companies from the mechanical and electrical industry, but also life sciences.
- Artificial Intelligence and Fintech challenges for the European banking and insurance industry
Speakers
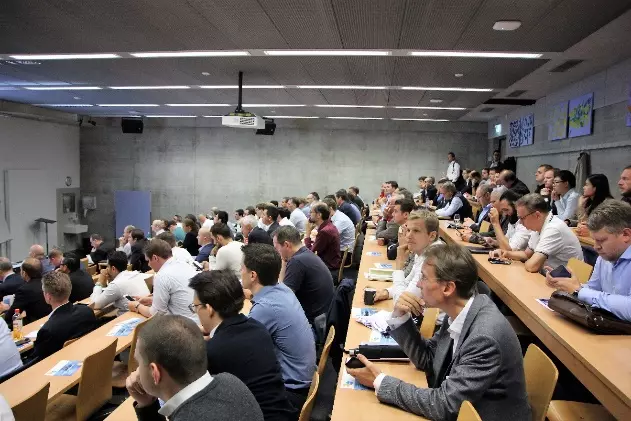
We have invited a large number of speakers both from within Switzerland as well as abroad, working on AI in Finance and Industry.
One track will focus on financial mathematics and its applications of machine learning, whereas the other one will tackle the implications for industry. In addition, we have a focus session on Fintech-Driven Automation in the Financial Industry.
Participants
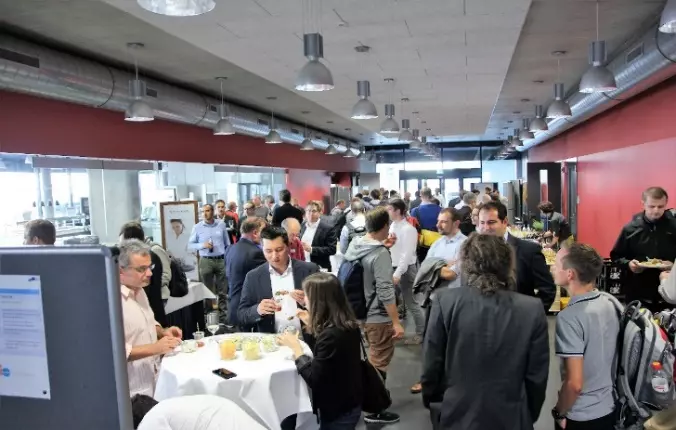
In September 2017, we have had more than 190 participants, both from Academia and Industry. The latest installment of the COST conference also saw a large number of international guests and speakers, travelling to Switzerland from destinations such as the UK, Germany, the United States and Bulgaria.
The largest proportion of participants will come from the industry complemented by a significant number of academic researchers. This mirrors our unique approach of connecting the academic world to their respective fields of application, putting new exciting concepts to work in industrial frameworks, where they can open up new opportunities.
Key Note Presenter
Prof. Dr. Marcello Pelillo, Università Ca'Foscari Venezia/ European Centre for Living Technology: "Opacity, Neutrality, Stupidity: Three Challenges for Artificial Intelligence"(PDF 4,7 MB)
Invited Speakers
Financial Mathematics
- Prof. Dr. Damian Borth, DFKI Kaiserslautern: "Deep Learning & Financial Markets: A Disruption and Opportunity"
- Dr. Daniel Egloff, Flink AI/QuantAlea: "Trade and Manage Wealth with Deep Reinforcement Learning and Memory"(PDF 1,3 MB)
- Prof. Dr. Paolo Giudici, University of Pavia: "Scoring Models for Roboadvisory Platforms: A Network Approach"
- Dr. Jürgen Hakala, Leonteq Securities AG: "Machine Learning applied to SLV Calibration"(PDF 2,3 MB)
- Prof. Dr. Markus Loecher, Berlin School of Economics and Law: "Pitfalls of Variable Importance Measures in Machine Learning"(PDF 2,6 MB)
- Dr. Yannik Misteli, Julius Bär: "Decision trees and ways on removing noisy labels"(PDF 2,9 MB)
- Anna Maria Nowakowska, InCube: "Recommender Systems for Mass Customization of Financial Advice"(PDF 1,2 MB)
- Dr. Alla Petukhina, Humboldt University of Berlin: "Portfolio allocation strategies in the cryptocurrency market"(PDF 9,2 MB)
- Prof. Dr. Peter Schwendner, ZHAW School of Management and Law: "Sentiment in European Sovereign Bonds"(PDF 2,0 MB)
- Prof. Dr. Marc Wildi, ZHAW School of Engineering: "FX-trading: challenging intelligence"(PDF 1,1 MB)
Industrial Mathematics
- Dr. Thomas Büttner, Ernst & Young: "Interpretability of Machine Learning Models"
- Dr. Lukas Hammerschmidt, Meteomatics: "Big Data meets weather: How real-time access to weather data enables a rapid development of business applications"
- Dr. Teodoro Laino, IBM: "Optimizing aluminum alloy's manufacturing using AI"
- Ricardo Pereira, Kühne & Nagel: "Reinventing Freight Logistics with Data Science"(PDF 8,1 MB)
- Dr. Stefan Scheib, Varian: "AI for Oncology at Varian – Potential Applications and Opportunities"(PDF 3,1 MB)
- Dr. Christian Spindler, PWC: "Trust in AI: explainability and compliance"(PDF 1,2 MB)
- Dr. Thilo Stadelmann, ZHAW: "Lessons Learned from Deep Learning in Industry"(PDF 2,4 MB)
- Marc Stampfli, NVIDIA: "Rise of modern AI with Deep Learning in Industry and Robotics"(PDF 4,7 MB)
- Dr. Tash ter Braack, Hilti: "Identifying and Prioritizing AI Applications"(PDF 1,9 MB)
- Dr. Volker Ziebart, ZHAW: "Artificial Intelligence for HVAC Systems"(PDF 3,6 MB)
Focus Session "Fintech-Driven Automation in the Financial Industry"
- Dr. Willi Brammertz, Ariadne Business Analytics : "Smart Contracts: The Basic Building Blocks of Future Digital Banks" (PDF 771,7 KB)
- Prof. Dr. Wolfgang Breymann, ZHAW: "A Prototype Environment for Financial Risk Modeling"(PDF 5,7 MB)
- Francis Gross, European Central Bank: "The economy as a network of contracts connecting a population of parties"(PDF 1,1 MB)
- Prof. Dr. Petros Kavassalis, Aegean University: "Blockchain and Financial Risk Reporting: design principles and formal reasoning"(PDF 599,5 KB)
- The Honorable Allan I. Mendelowitz, ACTUS Financial Research Foundation: "Regulation 2.0: Stress Tests and Oversight of Financial Risk"(PDF 854,1 KB)
- Marc Sel, PwC: "How smart contracts can implement the policy objective of 'report once' "(PDF 3,2 MB)
- Prof. Dr. Kurt Stockinger, ZHAW: "Data-Driven Financial Risk Modeling at Scale with Apache Spark"(PDF 1,1 MB)
- Panel Discussion: Sam Chadwick, Thomson Reuters; Arie Levy-Cohen, Blockhaus; George Williams, King & Spalding LLP; Wolfgang Breymann, ZHAW (Moderator)
Schedule
- Registration, Znüni: 08:30-09:00
- Welcome: 09:00-09:05
- Keynote: 09:05-09:50
- Thematic Sessions 1: 10:00-11:00
- Coffee: 11:00-11:30
- Thematic Sessions 2: 11:30-12:30
- Lunch: 12:30-14:00
- Thematic Sessions 3: 14:00-15:30
- Coffee: 15:30-16:00
- Thematic Sessions 4: 16:00-17:30
- Apéro Riche: 17:30
Detailed Schedule
Keynote (TN E0.46-54) | |||
09:00-09:05 | D. Wilhelm: "Welcome and Introduction" | ||
09:05-09:50 | M. Pelillo: "Opacity, Neutrality, Stupidity: Three Challenges for Artificial Intelligence" | ||
Finance 1 (TS 01.40) | Industry 1 (TN E0.46) | Focus Session 1 (TN E0.54) | |
10:00-10:30 | P. Giudici: "Scoring models for roboadvisory platforms: a network approach" | C. Spindler: "Trust in AI: explainability and compliance" | W. Breymann: "Short Overview" (10:00-10:15) |
10:30-11:00 | D. Borth: "Deep Learning & Finance Markets: A disruption and opportunity" | M. Stampfli: "Rise of modern AI with Deep Learning in Industry and Robotics" | F. Gross: "The economy as a network of contracts connecting a population of parties" (10:15-11:00) |
11:00-11:30 | Coffee (Foyer TN) | ||
Finance 2 (TS 01.40) | Industry 2 (TN E0.46) | Focus Session 2 (TN E0.54) | |
11:30-12:00 | M. Loecher: "Pitfalls of variable importance measures in Machine learning" | T. Laino: "Optimizing aluminum alloy's manufacturing using AI" | W. Brammertz: "Smart Contracts: The Basic Building Blocks of Future Digital Banks " |
12:00-12:30 | D. Egloff: "Trade and Manage Wealth with deep reinforcement learning and memory" | R. Pereira: "Reinventing Freight Logistics with Data Science" | A. Mendelowitz: "Regulation 2.0: Stress Tests and Oversight of Financial Risk" |
12:30-14:00 | Lunch (Foyer TN) | ||
Finance 3 (TS 01.40) | Industry 3 (TN E0.46) | Focus Session 3 (TN E0.54) | |
14:00-14:30 | A. Hoepner: "Embracing AI Opportunities = (Humans*Teamwork)^Machine -1" | L. Hammerschmidt: "Big Data meets weather" | P. Kavassalis: "Blockchain and Financial Risk Reporting: design principles and formal reasoning " |
14:30-15:00 | A. Petukhina: "Portfolio allocation strategies in cryptocurrency markets" | T. Stadelmann: "Lessons Learned from Deep Learning in Industry" | M. Sel: "How smart contracts can implement the policy objective of 'report once' " |
15:00-15:30 | Y. Misteli: "Decision trees in Machine Learning" | V. Ziebart: "Artificial Intelligence for HVAC Systems" | K. Stockinger: "Data-Driven Financial Risk Modeling at Scale with Apache Spark" |
15:30-16:00 | Coffee (Foyer TN) | ||
Finance 4 (TS 01.40) | Industry 4 (TN E0.46) | Focus Session 4 (TN E0.54) | |
16:00-16:30 | A. M. Nowakowska: "Recommender systems for mass customization of finance advice" | S. Scheib: "AI for Oncology at Varian – Potential Applications and Opportunities" | W. Breymann: "A Prototype Environment for Financial Risk Modeling" |
16:30-17:00 | J. Hakala: "Machine Learning applied to SLV Calibration" | T. Büttner: "Interpretability of Machine Learning Models" | Panel Discussion (S. Chadwick, A. Levy-Cohen, G. Williams, W. Breymann (Moderator)): "Next steps for real-world solutions" (16:30-17:30) |
17:00-17:30 | M. Wildi: "FX-trading: challenging intelligence" | T. ter Braack: "Identifying and Prioritizing AI Applications" | |
17:30-19:00 | Apéro riche (Foyer TN) | ||
Prof. Dr. Marcello Pelillo
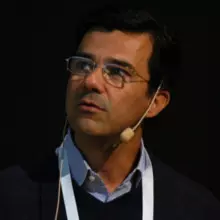
Marcello Pelillo is a Professor of Computer Science at the University of Venice, Italy, where he directs the European Centre for Living Technology and leads the Computer Vision and Pattern Recognition group, which he founded in 1995. He held visiting research positions at Yale University (USA), McGill University (Canada), the University of Vienna (Austria), York University (UK), the University College London (UK), and the National ICT Australia (NICTA) (Australia). He serves (or has served) on the editorial boards of IEEE Transactions on Pattern Analysis and Machine Intelligence, IET Computer Vision, Pattern Recognition, Brain Informatics, and is on the advisory board of the International Journal of Machine Learning and Cybernetics. He has initiated or chaired several conferences series (EMMCVPR, IWCV, SIMBAD, ICCV). He is (or has been) scientific coordinator of several research projects, including SIMBAD, a highly successful EU-FP7 project devoted to similarity-based pattern analysis and recognition. Prof. Pelillo has been elected a Fellow of the IEEE and a Fellow of the IAPR, and has been appointed IEEE Distinguished Lecturer (2016-2017 term).
Prof. Dr. Damian Borth
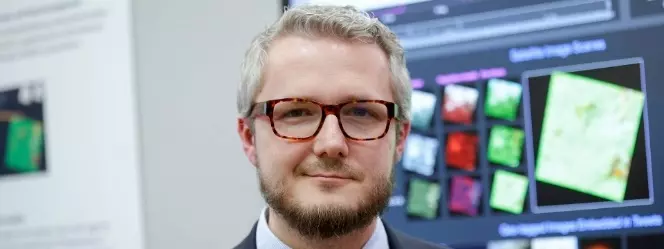
A Brief Biography
Dr. Damian Borth is a computer scientist and head of the centre of competence for deep learning at the German research center for artificial intelligence in Kaiserslautern (DFKI). He was awarded his PhD in Computer Science at the TU Kaiserslautern and the center of competence for multimedia analysis and data mining (MADM). For his achievements Borth and his team received various prizes, such as the McKinsey business technology award and the google research award.
Deep Learning & Financial Markets: A Disruption and Opportunity
Learning to detect fraud or accounting irregularities from low-level transactional data e.g. general ledger journal entries is one of the long-standing challenges in financial audits or forensic investigations. To overcome this challenge we propose the utilization of deep autoencoder or replicator neural networks. We demonstrate that the latent space representations learned by such networks can be utilized to conduct an anomaly assessment of individual journal entries. The representations are learned end- to-end eliminating the need for handcrafted features or large volumes of labelled data. Empirical studies on two accounting dataset support our hypothesis. We evaluated the methodology utilizing two anonymized and large scaled datasets of journal entries extracted from Enterprise Resource Planning (ERP) systems.
Dr. Daniel Egloff
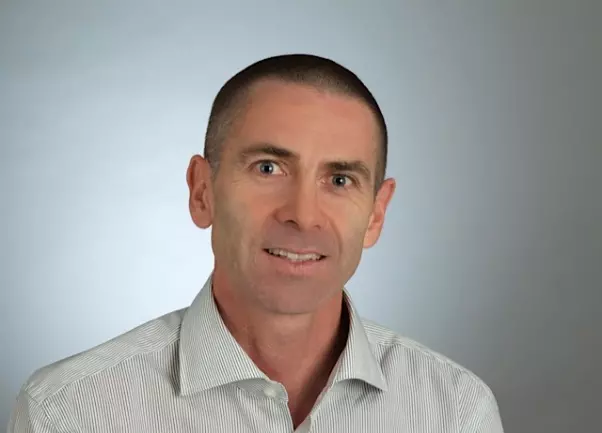
A Brief Biography
Dr. Daniel Egloff is the founder of Flink AI and QuantAlea. Flink AI is developing new AI solutions using Reinforcement Learning and is advising banks, hedge funds and eCommerce companies on practical applications of AI and Deep Learning. QuantAlea is a Swiss based software engineering company specialized in GPU software development and high performance numerical computing. He studied mathematics, theoretical physics and computer science and worked for more than 15 years as a quant in the financial service industry before he started his entrepreneurial career in 2007.
Trade and Manage Wealth with deep Reinforcement Learning and Memory
In this session we present how Deep Reinforcement Learning (DRL) and memory extended networks can be used to train agents, which optimize asset allocations or propose trading actions. The memory component is crucial for improved mini-batch parallelization and helps to mitigate catastrophic forgetting. We also address how concepts from risk sensitive and safe reinforcement learning apply to improve the robustness of the learned policies. The DRL approach has several advantages over the industry standard approach, which is still based on the Mean Variance portfolio optimization. The most significant benefit is that the information bottleneck between the statistical return model and the portfolio optimizer is removed and that the available market data and trade history is used much more efficiently
Prof. Dr. Paolo Giudici
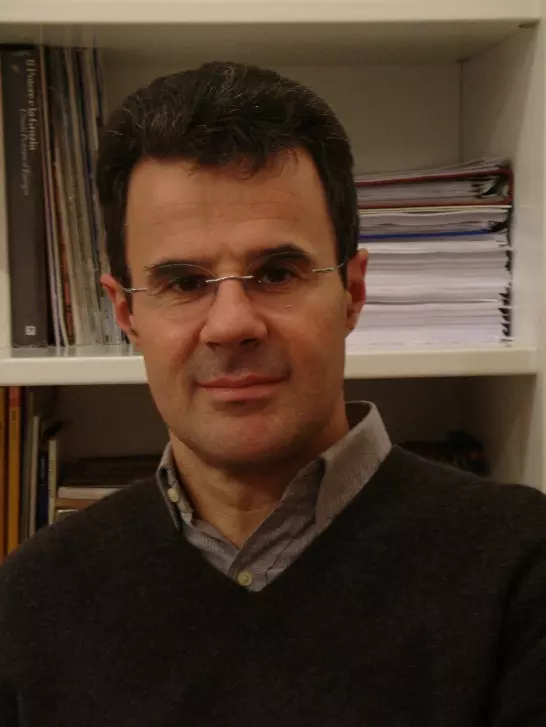
A Brief Biography
Professor of Statistics and Data Science at the Department of Economics and Management of the University of Pavia. His current research interests are: Financial networks, Financial risk management, Systemic risk, and their application to Cryptocurrencies and Fintech platforms. Director of the University of Pavia Financial Technology laboratory (formerly Data Mining laboratory) which, since 2001, carries out research and consulting projects, for leading financial institutions.
Scoring Models for Roboadvisory Platforms: A Network Approach
Due to technological advancement, robo-advice platforms have allowed significant cost reduction in asset management. However, this improved allocation may come at the price of a biased risk estimation. To verify this, we empirically investigate allocation models employed by robo-advice platforms. Our findings show that the platforms do not accurately assess risks and, therefore, the corresponding allocation models should be improved, incorporating further information, through clustering and network analysis.
Dr. Jürgen Hakala
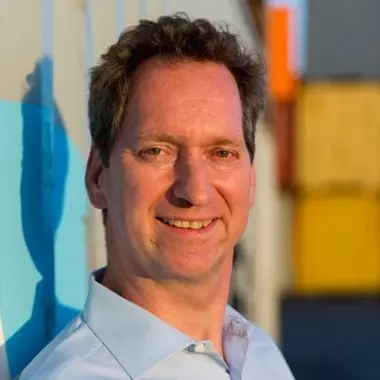
A Brief Biography
Jürgen works for Leonteq Securities AG, where he is involved in modelling and financial engineering for all asset classes. His interests are numerical methods in mathematical finance, in particular multi-asset and hybrid modelling, as well as the impact of regulation onto markets and models. Backed by his PhD was on Neural Network he recently rekindled his interest in machine learning methods, now applied to problems in financial engineering. Initially he worked on foreign exchange, where he is co-editor of a textbook about FX derivatives.
Machine Learning applied to SLV Calibration
We calibrate local stochastic volatility using the particle method developed by [Guyon, Henry-Labordere]. A critical step in this method is an estimation of the conditional expectation of the stochastic volatility process, given the realized spot. We reformulate this estimation as a non-linear regression at each time-step of the discretized process, which allows us to apply machine-learning (ML) techniques. We review appropriate ML techniques, compare results and Performance.
Prof. Dr. Andreas Hoepner
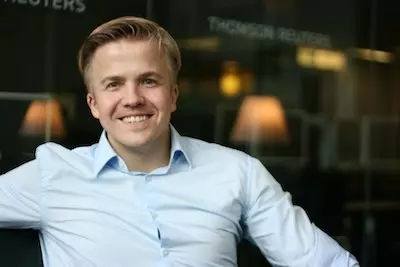
Prof. Dr. Andreas Hoepner
Professor Andreas G. F. Hoepner, Ph.D., is a Financial Data Scientist working towards the vision of a conflict-free capitalism. Formally, Dr. Hoepner is Full Professor of Operational Risk, Banking & Finance at the Michael Smurfit Graduate Business School and the Lochlann Quinn School of Business of University College Dublin (UCD).
Andreas is also heading the ‘Practical Tools’ research group of the Mistra Financial Systems (MFS) research consortium which aims to support Scandinavian and global asset owners with evidence-based tools for investment decision making.
Furthermore, he is currently a visiting Professor in Financial Data Science at the University of Hamburg, as well as at the ICMA Centre of Henley Business School, were he was an associate Professor of Finance.
Embracing AI Opportunity = (Humans*Teamwork)^Machine -1
Prof. Hoepner argues that AI and augmented Intelligence (AugmI) can both have huge potential, with the use case suggesting which one to deploy, and how to organize ones team. He cites Gary Kasparov's observation that the Freestyle Chess Championships were won neither by the best grand master nor the best machine but by the best human-machine team: two amateur chess players using three machines simultaneously. Based on an immediacy, confirmability, population size and time-series attributes of use cases, he argues that AI is superior for real-time repeated recognitions of static objects, while AugmI is likely to remain preferred choice for quite a while in regular but not real-time predictions of reactive processes. AugmI also demands a strong focus on creating an excellent teamwork between among all involved humans and them and their machine(s).
Lastly, Prof. Hoepner connects both AI abbreviations with the pressing need of climate change mitigation based on the use case of corporate GHG emissions reporting.
Prof. Dr. Markus Loecher
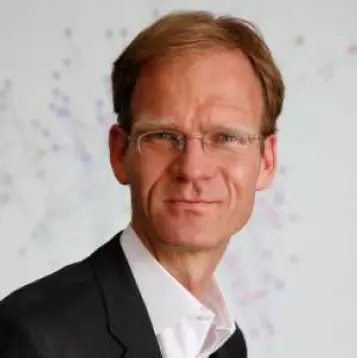
A Brief Biography
Prof. Dr. Markus Loecher has been a professor of mathematics and statistics at the Berlin School of Economics and Law (HWR Berlin) since 2011. His research interests include machine learning, spatial statistics, data visualization and sequential learning. Prior to joining HWR Berlin he worked as principal and lead scientist at various data analytics companies in the United States. In 2005, he founded a consulting firm, DataInsight, which focused on applying novel statistical learning algorithms to massive data sets. Prior to DataInsight, he worked at Siemens Corporate Research (SCR) in Princeton, NJ for 5 years, where he focused on failure prediction. Markus Loecher completed his postdoctoral research in physics at the Georgia Tech University in which he studied the spatiotemporal chaos. He holds a PhD in physics and a master degree in statistics.
Pitfalls of Variable Importance Measures in Machine Learning
Random forests and boosting algorithms are becoming increasingly popular in many scientific fields because they can cope with "small n large p" problems, complex interactions and even highly correlated predictor variables. The predictive power of covariates is derived from the permutation based variable importance score in random forests. It has been proven that these variable importance measures show a bias towards correlated predictor variables. We demonstrate the fundamental dilemma of variable importance measures as well as their appeal and wide spread use in practical data science applications. We address recent criticism of the reliability of these scores by residualizing and deriving analogous procedures to the F-test.
Dr. Yannik Misteli
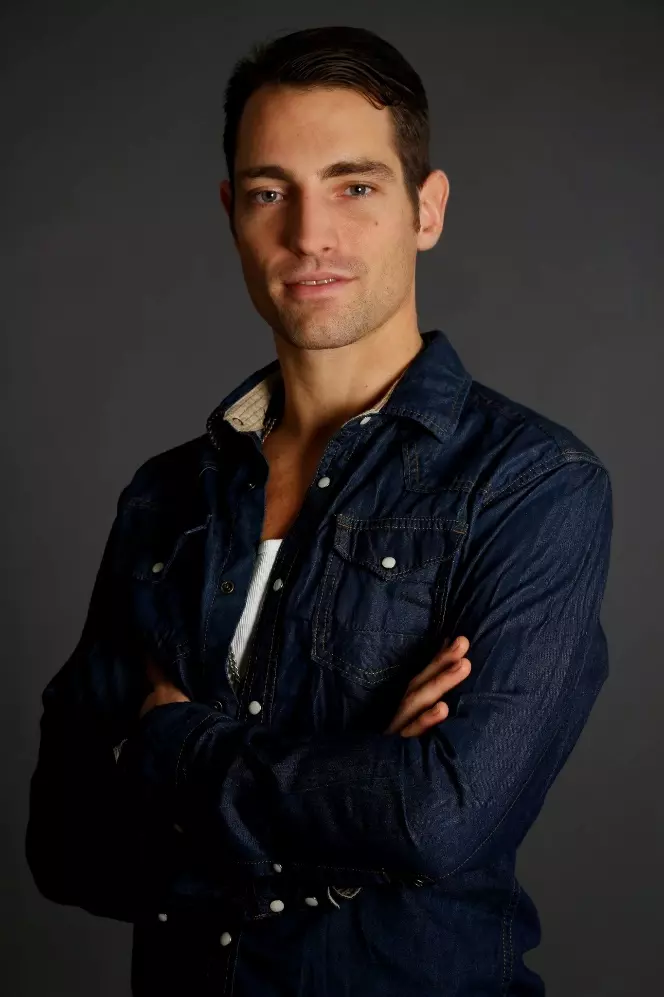
A Brief Biography
Dr. Yannick Misteli leads the Advisory Analytics Team at Julius Bär in Zürich. The team develops new data based approaches with the aim of better supporting the relationship managers who serve the clients. During his PhD he gained experience in numerical high-performance computing, multi-objective optimisation algorithms as well as statistical mechanics. He was always keen to transfer his insights gained from academic research over to industry and is now enjoying working to shape the future of the financial sector.
Decision trees and ways on removing noisy labels
Decision trees neither are the most sophisticated classifier nor are they accurate predictors. However, they are a powerful instrument to establish machine-learning techniques within a company as they are easily and intuitively interpreted. They can be used to gradually build up understanding and confidence in using machine learning techniques amongst (senior) management, introducing more sophisticated methods once this confidence has been achieved. We demonstrate the use of decision trees in the context of identifying clients that should be moved to a different service model. A simple partition tree classifier is used to model the different customers and hence the leaf nodes are investigated for misclassified clients.
Anna Maria Nowakowska
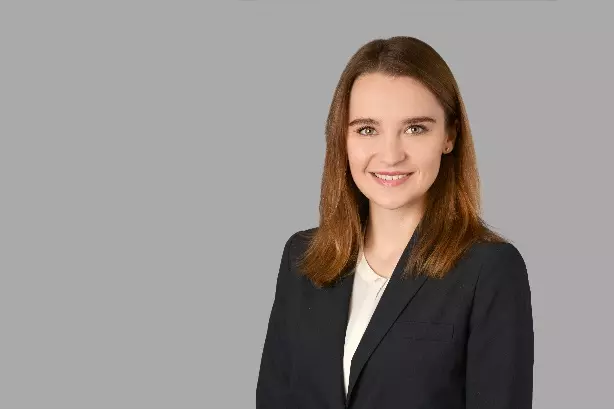
A Brief Biography
Anna Maria Nowakowska leads the Data Analytics team at InCube, which focuses on delivering data consulting services to clients within the Swiss financial sector. She holds a Master of Engineering degree in Electronics and Electrical Engineering from the University of Edinburgh, as well as the Chartered Financial Analyst® designation from the CFA Institute. She has over 7 years of experience in the software and financial services industries and has worked in the UK, US and Switzerland.
Recommender Systems for Mass Customization of financial Advice
Recommender systems have been widely adopted in areas such as online shopping and movie streaming. They automatically suggest new items to users based on their characteristics and previous behaviour. Despite the support that recommender systems can bring to decision making in finance, their application to banking data is an underexplored field, and our research is focused on filling this gap. We build recommenders for private and retail banking use cases, following the growing push for digitization and mass customization of financial advice. The vision is to enhance the quality of personal financial advice and to make it accessible to a wider client base, by automating a large part of the process.
Dr. Alla Petukhina
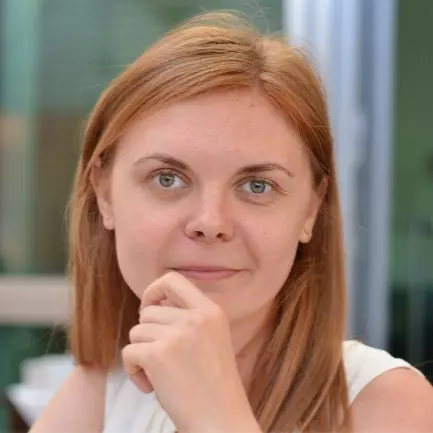
A Brief Biography
Alla Petukhina holds a M.Sc. in economics from the Ural state university, Russia. Since 2014 she has joined the Ladislaus von Bortkiewicz chair of statistics at the Humboldt-University in Berlin as a Ph.D. candidate. Her research interests are focused on asset allocation strategies and risk modelling for high-dimensional portfolios, investment strategies in crypto-currencies market.
Portfolio allocation strategies in the cryptocurrency market
Current study aims to identify pro and con arguments of crypto-currencies as a new asset class in portfolio management. We investigate characteristics of the most popular portfolio-construction rules such as Mean-variance model (MV), Risk-parity (ERC) and Maximization diversification (MD) strategies applied to the universe of cryptocurrencies and traditional assets. We evaluate the out-of-sample portfolio performance as well as we explore diversification effects of incorporation of crypto-currencies into the investment universe. Taking into account a low liquidity of crypto-currency market we also analyze portfolios under liquidity constraints. The empirical results show crypto-currencies improve the risk-return profile of portfolios. We observe that crypto-currencies are more applicable to target return portfolio strategies than minimum risk models. We also found that the MD strategy in this market outperforms other optimization rules in many aspects.
Prof. Dr. Marc Wildi
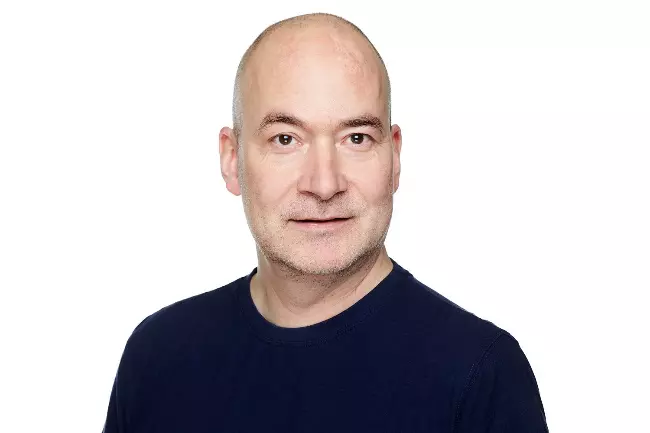
A Brief Biography
Marc Wildi holds an M.Sc. in Mathematics from the Swiss Federal Institute of Technology (ETH) in Zurich; he obtained his PhD from the University of St-Gallen, Switzerland. After being lecturer in Statistics at the Universities of Fribourg and of St-Gallen, he began his current position as a Professor in Econometrics in 2002 at the Zurich University of Applied Sciences. His novel forecast and signal extraction methodology, the so-called Multivariate Direct Filter Approach (MDFA), won two international forecast competitions in a row. His current research interests involve applications of the MDFA to mixed-frequency (daily) macro-economic indicators and to algorithmic trading.
FX-trading: challenging intelligence
FX-trading is widely recognized as one of the most challenging forecast applications with the range of methodological complexity reaching from appalling simplicity to frightening complexity. We here sweep through this methodological range by proposing a series of novel and less novel, linear and non-linear, intelligent and less so approaches, either in isolation or in combination. Empirical results are benchmarked against plain-vanilla approaches, based on the most liquid and therefore most challenging (FX-)pairs. R-users will be pleased to replicate results.
Thomas Büttner
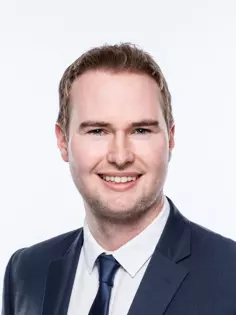
A Brief Biography
Thomas Büttner is working as a Consultant at EY in the Quant and Analytics Advisory team of the EMEIA Financial Services Risk practice in Zurich. At EY, he is using Machine Learning for various applications in the financial sector such as transaction monitoring, credit scoring and data quality remediation. Before joined EY in 2016, he completed his experimental and theoretical PhD research in the field of nonlinear integrated photonics at the University of Sydney in Australia.
Interpretability of Machine Learning Models
For many data science applications it is imperative to understand why models make certain predictions. Interpretable models allow for the required trust into model predictions and provide insight into how the model may be improved. With the increasing size of datasets and the increasing importance of unstructured data, however, the best accuracy is often achieved by using complex models that are difficult to interpret. This creates a trade-off between model performance and model interpretability. In this presentation, I will discuss the interpretability of different Machine Learning algorithms as well as different approaches to explaining predictions of complex models.
Dr. Lukas Hammerschmidt
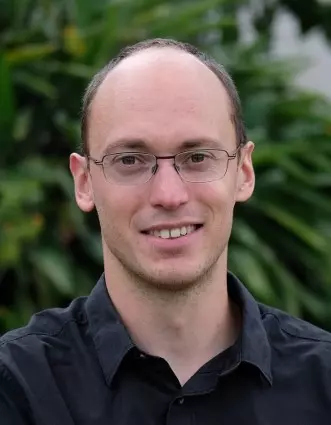
A Brief Biography
Lukas Hammerschmidt studied chemistry at FU Berlin and wrote a PhD thesis on theories of nanostructured thermoelectric materials. Thereafter, he was postdoc at the University of Wellington and Auckland. The core of his work was in particular the implementation of the previously developed scientific methods on high-performance computers.About a year ago, he joined Meteomatics and works on our big data solution "MeteoCache" and hydrologic applications thereof.
Big Data meets weather: How real-time access to weather data enables a rapid development of business applications
The availability of quality weather data has improved dramatically over the past decade. At the same time the number of big data analytics businesses delivering sector-specific solutions and business insights has also grown dramatically. However timely access to quality weather data, as cut outs that are suited to specific business requirements, delivered in formats that users can simply apply to new and existing in-house systems and models has remained a challenge. Meteomatics is a commercial weather data provider that is working collaboratively with National Met Services (NMSs), Academia and Scientific communities. We bring together historical, nowcast and forecast weather data from global models such as the ECMWF model, satellite operations and station data. By applying in-house modelling and downscaling capabilities, Meteomatics is able to deliver weather data for any lat / long and time series to use in 3rd party models via an industrial scale robust Weather API. Weather data enabled insights are relevant to many sectors in industry. For instance, aviation, automotive, energy, logistics, insurances and others, both public and private: In the field of agriculture, weather risk management solutions are already protecting the crops of farmers across Africa from drought and innovative start-ups around the globe are applying weather data to a variety of models to meet precision farming challenges. Energy companies, both in the traditional and renewable sectors, are extensively using these solutions to forecast demand, power output, inform energy trading, protect themselves against unfavourable seasons and safeguard revenues. Meanwhile, wind farm operators seek protection against low or excessively strong wind to secure cash flow and underpin their financing. Marine insurers are combining vessel tracks and crew behaviours in differing weather conditions to influence their view of risk, and Lloyd’s of London are using historical weather data and ship tracks to identify fraudulent marine claims. Water utilities are enhancing demand and leakage models, better managing system capacity and ensuring regulatory compliance through weather-enabled automation of alarms, catchment modelling and enhanced workforce management. So, in summary, simple API access to quality weather data is extending the understanding of weather risk for a broad range of sectors. The speed of development of new products and services underpinned by quality weather data, indices, benchmarks and parametric triggers is growing rapidly.
Dr. Teodoro Laino
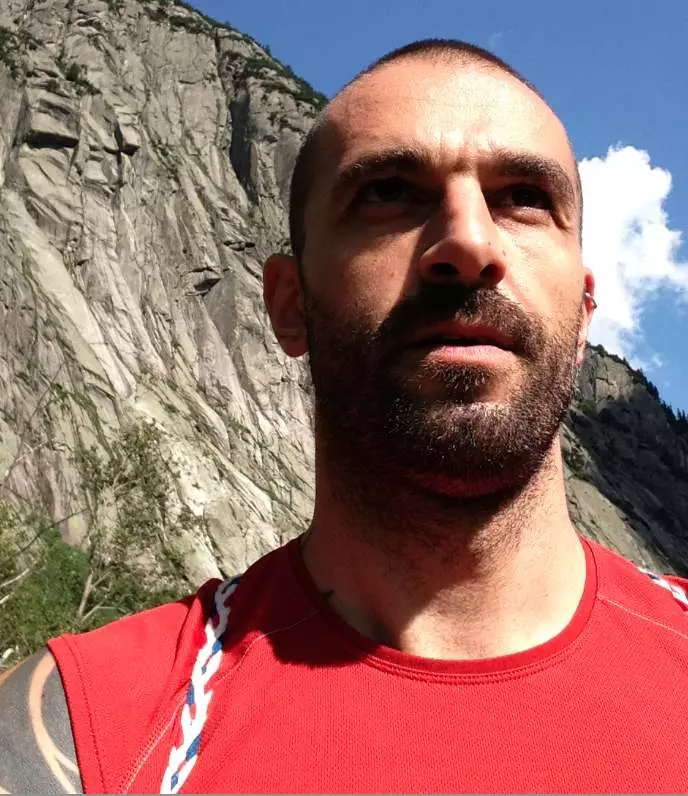
A Brief Biography
Teodoro Laino received his degree in theoretical chemistry in 2001 (University of Pisa and Scuola Normale Superiore di Pisa) and the doctorate in 2006 in computational chemistry at the Scuola Normale Superiore di Pisa, Italy. His doctoral thesis, entitled "Multi-Grid QM/ MM Approaches in ab initio Molecular Dynamics" was supervised by Prof. Dr. Michele Parrinello. From 2006 to 2008, he worked as a post-doctoral researcher in the research group of Prof. Dr. Jürg Hutter at the University of Zurich, where he developed algorithms for ab initio and classical molecular dynamics simulations. Since 2008, he has been working in the department of Cognitive Computing and Industry Solutions at the IBM Research - Zurich Laboratory (ZRL). In 2015, he was appointed technical leader for molecular simulations at ZRL.
The focus of his research is on complex molecular dynamics simulations for industrial-related problems (energy storage, life sciences and nano-electronics) and on the application of machine learning/artificial intelligence technologies to chemistry and materials science problems.
Optimizing aluminum alloy's manufacturing using AI
In this talk, we will present an AI strategy to optimize the composition and process for the production of alloys with tailored mechanical properties, relevant to metallurgical industries. The work makes use of a variational autoencoder (VAE) to transform discrete data into a continuous representation. Subsequently, optimisation algorithms search for an alloy's optimal properties in the previously obtained continuous representation. The entire framework will be presented and discussed.
Ricardo Pereira
A Brief Biography
Today at Logindex (an affiliate of Kuehne+Nagel) he is responsible to bring to life new business solutions, that are on the border of logistics and finance, with a focus on science- and data-driven products.
Between 2006 and 2015, he worked as quant analyst in Investment Banking and Wealth Management in Zürich, in areas of risk engineering and sales analytics respectively.
Before moving to Switzerland he held a few development consultant roles in financial and telecommunication institutions.
Graduated in 1994 from Instituto Superior Tecnico of Lisbon, Portugal as Computer Engineer and hold a MBA from INSEAD since 2006.
Reinventing Freight Logistics with Data Science
By either sea, air or road, the business of freight transportation hasn't seen major quantum leaps, except for the introduction of the container in the 50s.
But today the "perfect storm" approaches this industry: with public AIS vessel tracking, weather forecast data, IoT sensors, NLP applied to documentation or even blockchain, we are working on reinventing the business, with the help of data science.
In this session we'll present some of our work while forecasting Trade Balance for financial agents by monitoring in realtime the flow physical goods, estimating vessel capacity utilisation, forecasting the ETA of containerships or benchmarking the performance of carriers, ports and terminal handlers.
Dr. Stefan Scheib
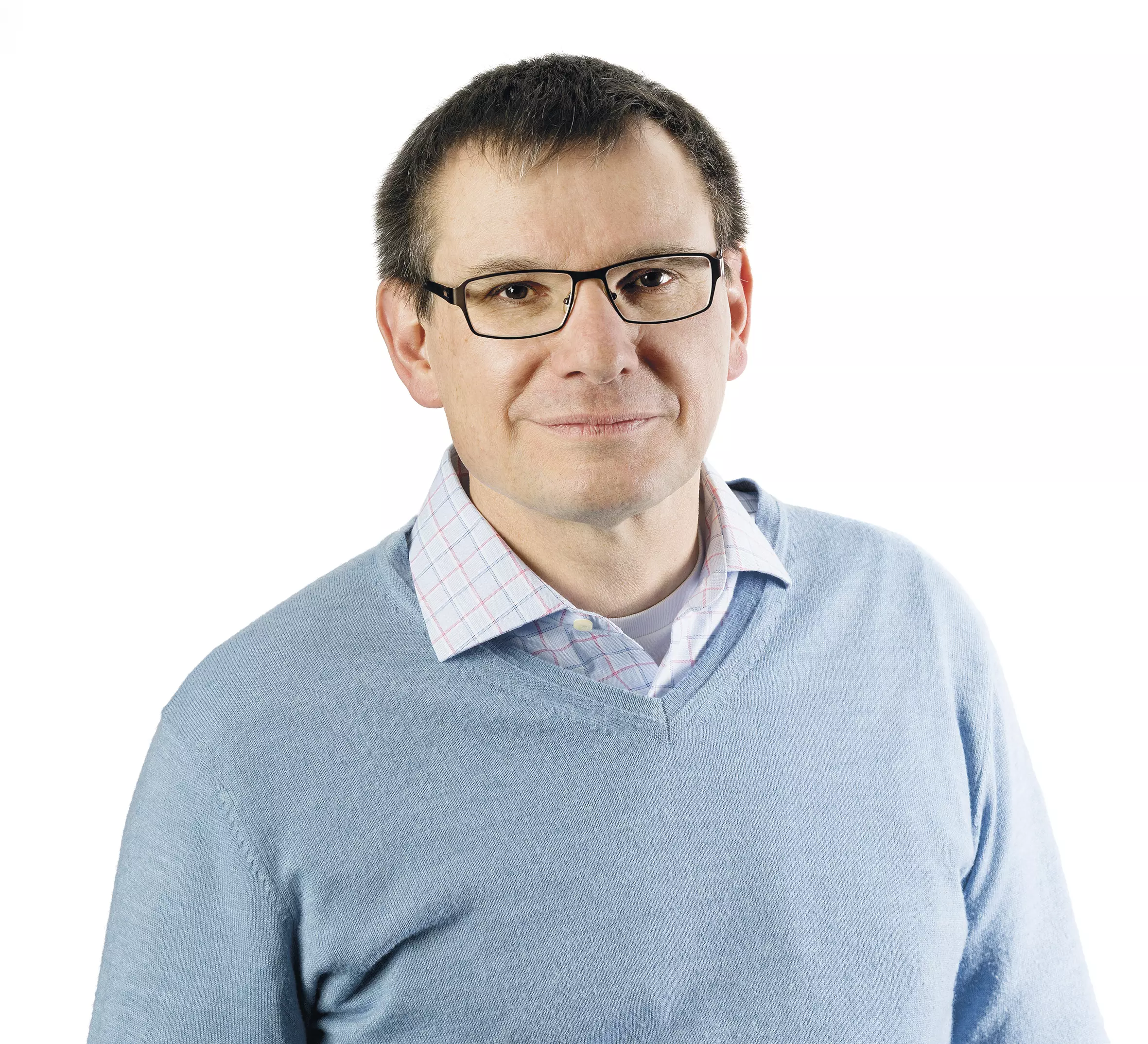
A Brief Biography
Stefan Scheib received his degree in Physics in 1989 from the University of Karlsruhe and his PhD from ETHZ in Physics in 1994. During his PhD studies he worked at the Paul Scherrer Institute in Villigen, Switzerland, pioneering proton radiation therapy. Staying in this field he was engaged in introducing stereotactic radiosurgery in Switzerland within the Private Clinic Group Hirslanden and did his Master of Advanced Studies in Medical Physics at ETHZ leading to the national certification in medical radiation physics according to the Swiss Radiation Protection Ordinance in 1996. He also studied teaching for secondary schools at the University Zurich and became a teacher at the radiation technician school in Zurich and is currently lecturing at the University of Applied Sciences in Buchs, SG. In 2007 he joined the Imaging Laboratory of Varian Medical Systems as Scientific Advisor and since 2016 he is acting as Senior Manager Applied Research at this R&D site of Varian, specialized in medical imaging. His applied research activities are dedicated to novel imaging and treatment devices including hard- and software solutions.
AI for Oncology at Varian – Potential Applications and Opportunities
Varian Medical Systems, the market leader in radiation oncology hard- and software solutions has a long track record in transforming technical innovation into successful customer facing products, touching cancer patients throughout the world. Artificial Intelligence (AI), Machine Learning (ML) and Deep Learning (DL) technologies made their way into every day’s applications and are currently of huge interest in various domains.
Looking closer into this technology, we realized the potential in various areas throughout our product portfolio. Currently several applied research projects around automated workflows, image processing and fully automated organ segmentation have been initiated to explore the value of this technology in detail within our domain. Early results are promising and soon we will open our AI environment to interested research customers to explore the practical clinical value of these solutions prior a product development decision will be made.
In this presentation promising early results, implementation strategies and product opportunities will be discussed to demonstrate the potential added value of AI technology in a clinical radiation oncology environment.
Dr. Christian Spindler
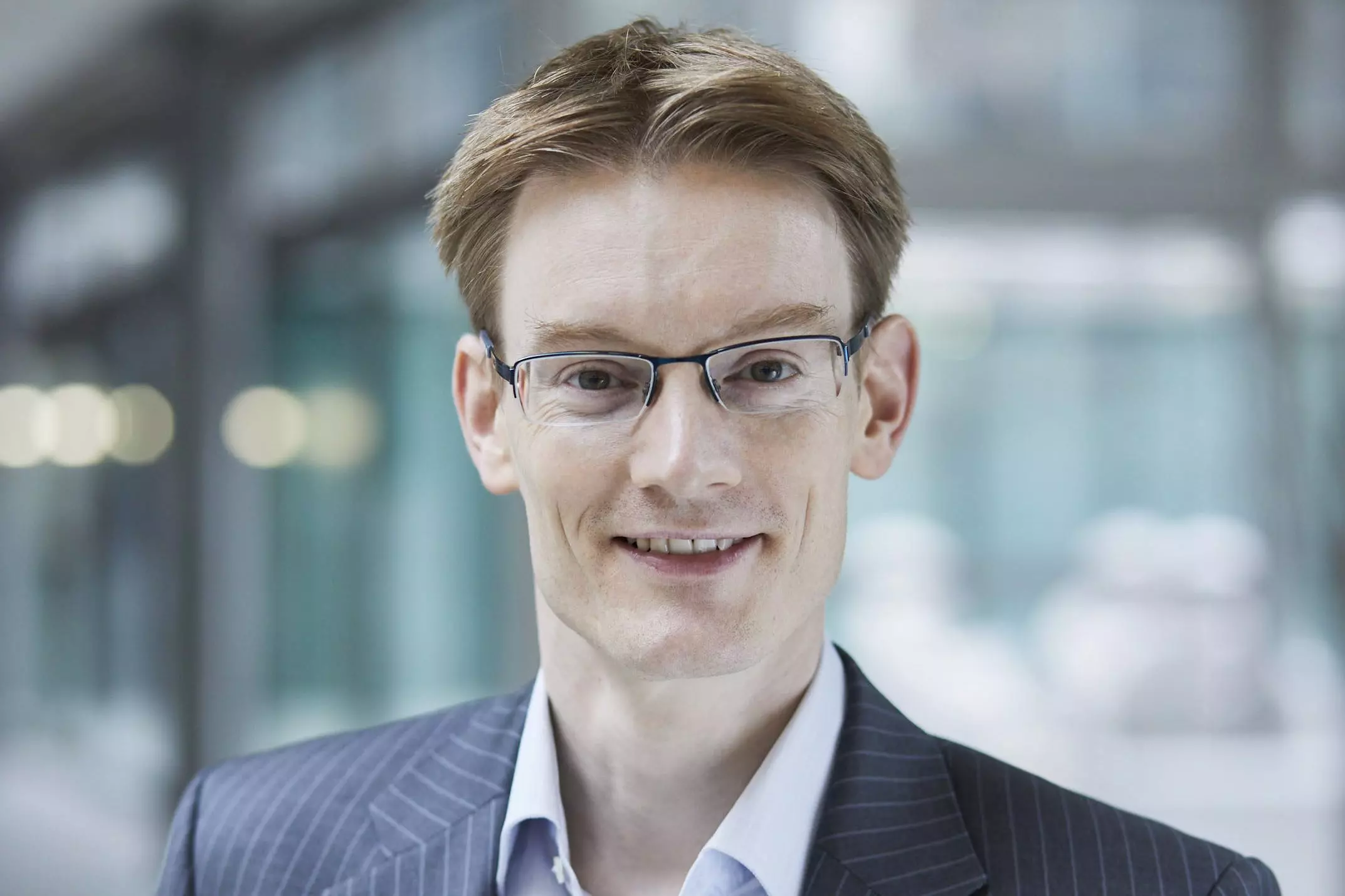
A Brief Biography
Christian Spindler is Data Scientist at PwC’s Artificial Intelligence Center of Excellence in Zürich. Educated in physics, Christian Spindler accumulates 10+ years of experience with machine learning and deep learning modelling for various applications in financial services, insurance, manufacturing and robotics.
Explainable and responsible AI for financial Services and Insurance
Decisions taken by machine learning / artificial intelligence systems cannot be assessed using traditional source code analysis. The final behavior of such algorithms is defined during training, not during programming. PwC develops services based on LIME, QII and other assessment techniques for black-box systems to build trust in AI. We analyze the influence of individual input factors and search for bias, possibly leading to overreactions on specific inputs. We determine whether algorithms are fully trained and identify potential risks of developing an algorithm into production.
We demonstrate a case study for responsible AI in Financial Services, particularly the insurance of autonomous, driverless vehicles. We cover various aspects of trustful AI systems and show how to integrate them in daily business operation.
Dr. Thilo Stadelmann
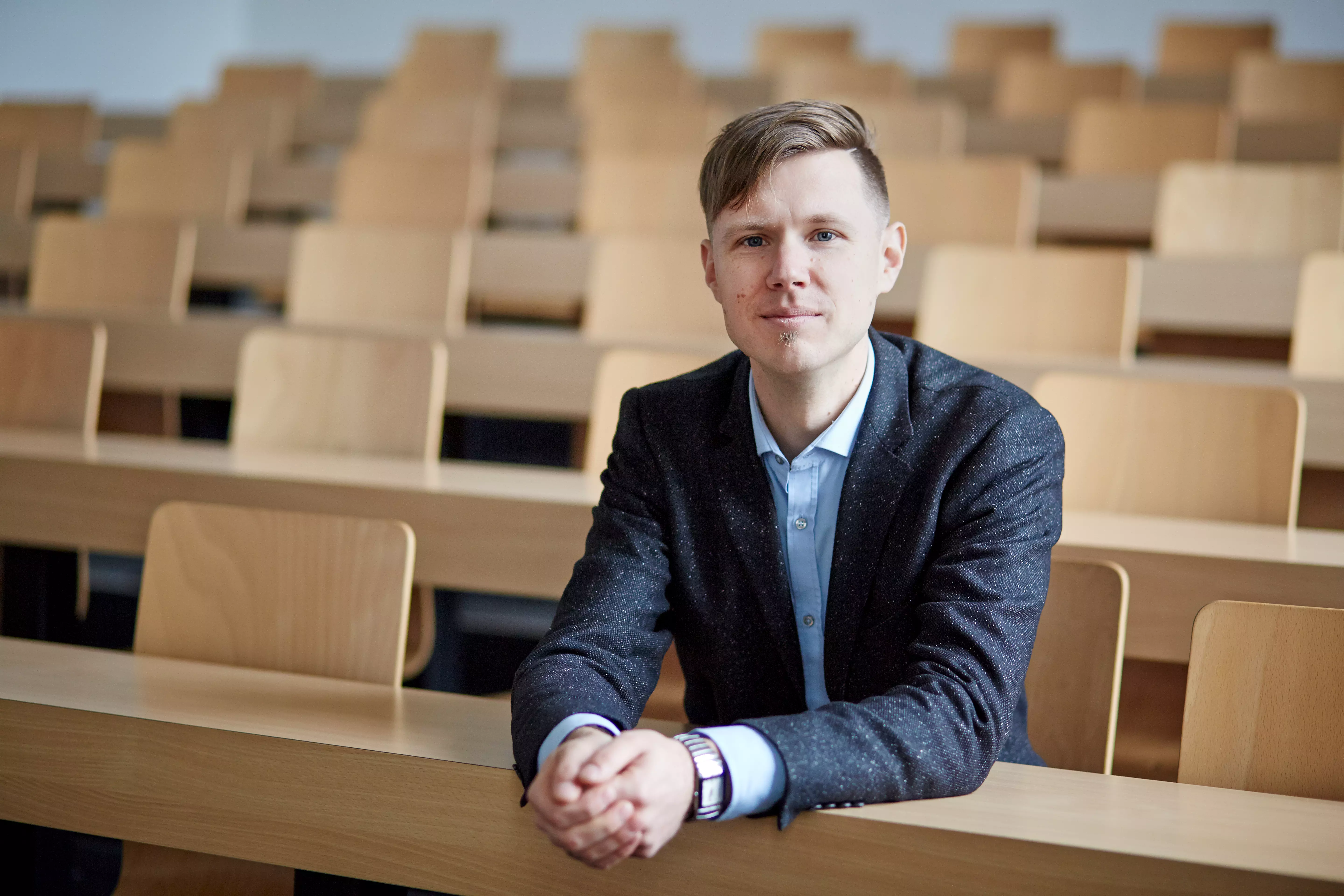
A Brief Biography
Thilo Stadelmann is senior lecturer of computer science at ZHAW School of Engineering in Winterthur. He received his doctor of science degree from Marburg University in 2010, where he worked on multimedia analysis and voice recognition. Thilo joined the automotive industry for 3 years prior to switching back to academia. His current research focuses on applications of machine learning, especially deep learning, to pattern recognition problems on diverse kinds of data. He is head of the ZHAW Datalab and vice president of SGAICO, the Swiss Group for Artificial Intelligence and Cognitive Sciences.
Lessons Learned from Deep Learning in Industry
Deep learning with neural networks is applied by an increasing number of people outside of classic research environments, due to the vast success of the methodology on a wide range of machine perception tasks. While this interest is fueled by beautiful success stories, practical work in deep learning on novel tasks without existing baselines remains challenging. In this talk, we explore the specific challenges arising in the realm of real world tasks, based on case studies from research & development in conjunction with industry, and extract lessons learned from them. This fills a gap between the publication of latest algorithmic and methodical developments, and the usually omitted nitty-gritty of how to make them work. For example, we give insight into deep learning projects on face matching, industrial quality control or music scanning, thereby providing best practices for deep learning in practice.
Marc Stampfli
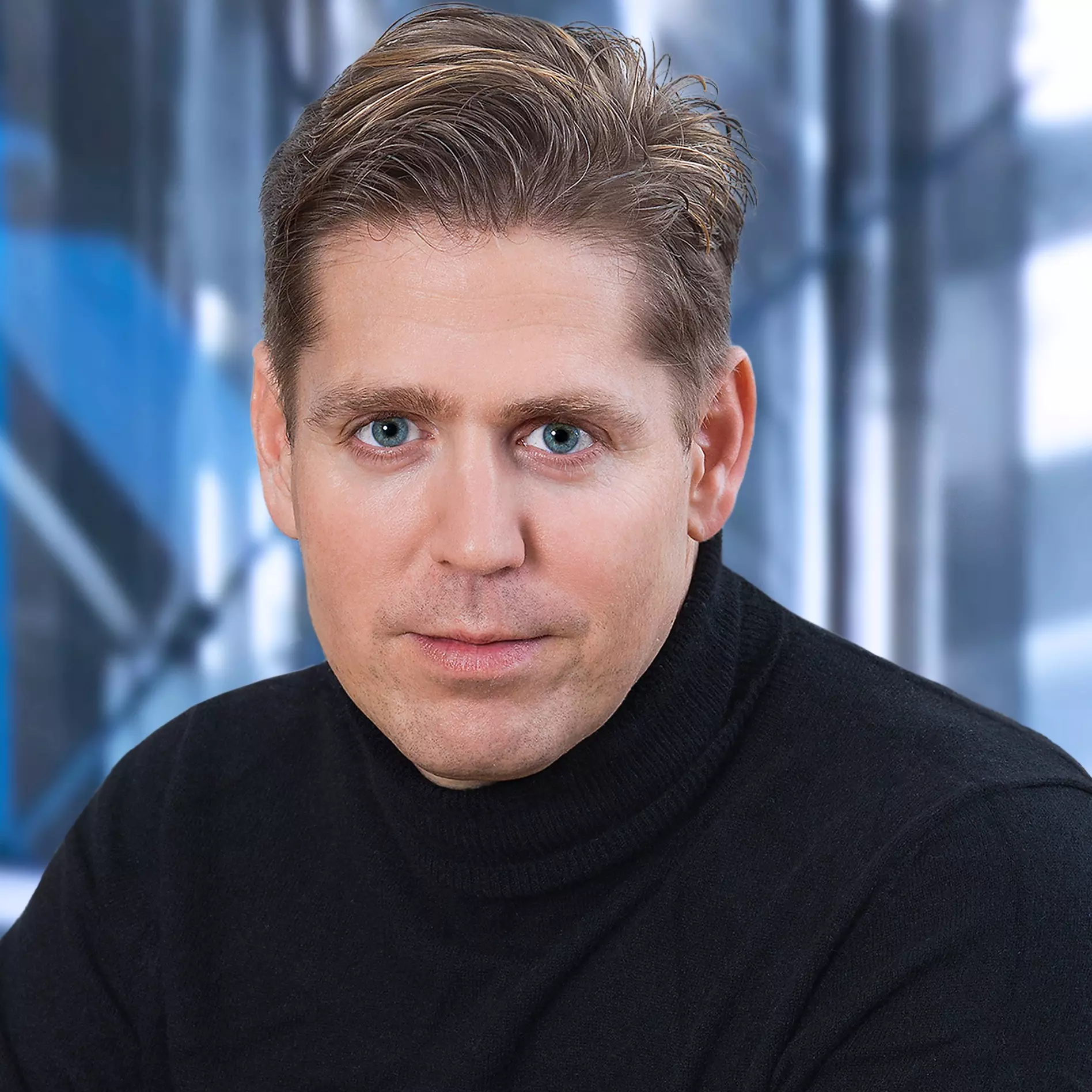
A Brief Biography
Marc Stampfli is computer scientist and responsible to build the market for NVIDIA in Switzerland focussing on modern artificial intelligence with deep learning. He has a master in computer science from University of Zurich and has more than 18 years of experience in developing new technology market segments for enterprise companies. Before NVIDIA, he was working in leading positions for major technology companies from Silicon Valley such as IBM, Oracle, but as well traditional companies such as Colt Technology Services and PricewaterhouseCoopers. He is an enthusiastic speaker that is able to explain difficult technological topics in simple words so that everybody can follow and understand.
Rise of modern AI with Deep Learning in Industry and Robotics
Internet, mobile computing, inexpensive sensors (IoT) collecting terabytes of real world data. The rise of deep learning that can use that data in real-time will fundamentally change the way that industries will innovate. Smart Machines, autonomous Vehicles, Robots and Drones will change our everyday life as we know it today.
In this session we will explain the rise of technology and show case real examples from customers of NVIDIA which already use successful deep learning today in smart machines, autonomous cars, drones or robots. The cases range from different appliances like preventive maintenance, to autonomous vehicles for logistics, to robots that train their neuronal network in virtual reality to be used in real world.
Dr. Tash Ter Braack
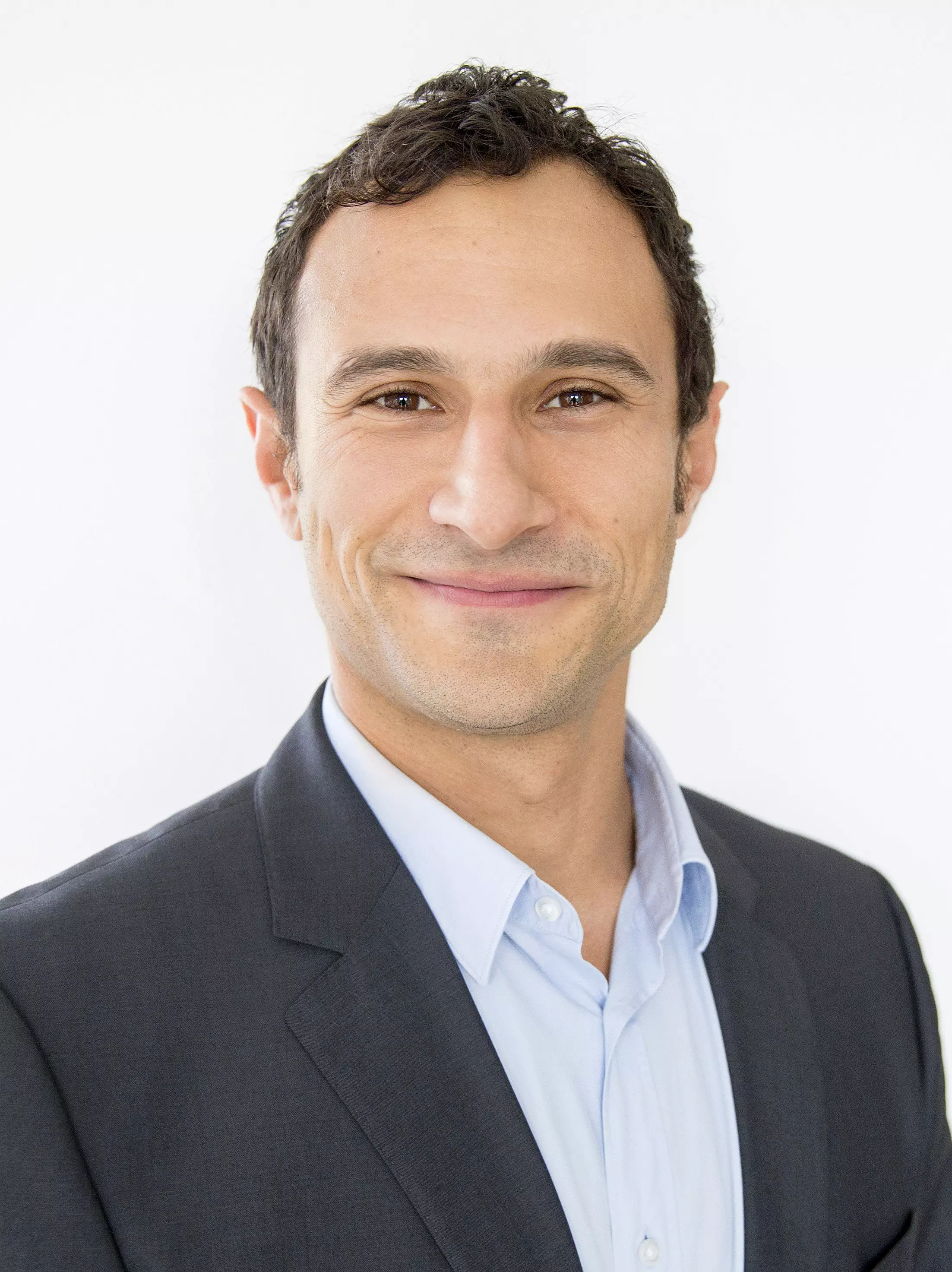
A Brief Biography
Dr. T. Ter Braack is Project Manager Mechantronics and Artificial Intelligence at the Corporate Research and Technology center at Hilti AG in Liechtenstein. His projects focus on creating smart tools and prescriptive maintenance with a focus on innovation and machine learning. He co-founded the Hilti Start-up incubator for interns. Previously Dr. Ter Braack worked in robotics (ROS) creating innovative solutions for small to medium businesses. He holds a Ph.D. in Mechanical Engineering from the University of California, Davis.
Identifying and Prioritizing AI Applications
Artificial Intelligence is a popular topic for companies these days. Many companies have the perception that they can leverage data for financial profits. Usually the first steps are taken with external consultants yielding disappointing results. This talk will give a brief summary of the lessons learned from the initial implementation of Artificial Intelligence at Hilti AG. The results of numerous workshops are aggregated to help other companies on the journey into effectively and efficiently absorbing this technology. Methods for identifying and prioritizing applications will be proposed and discussed.
Dr. Volker Ziebart
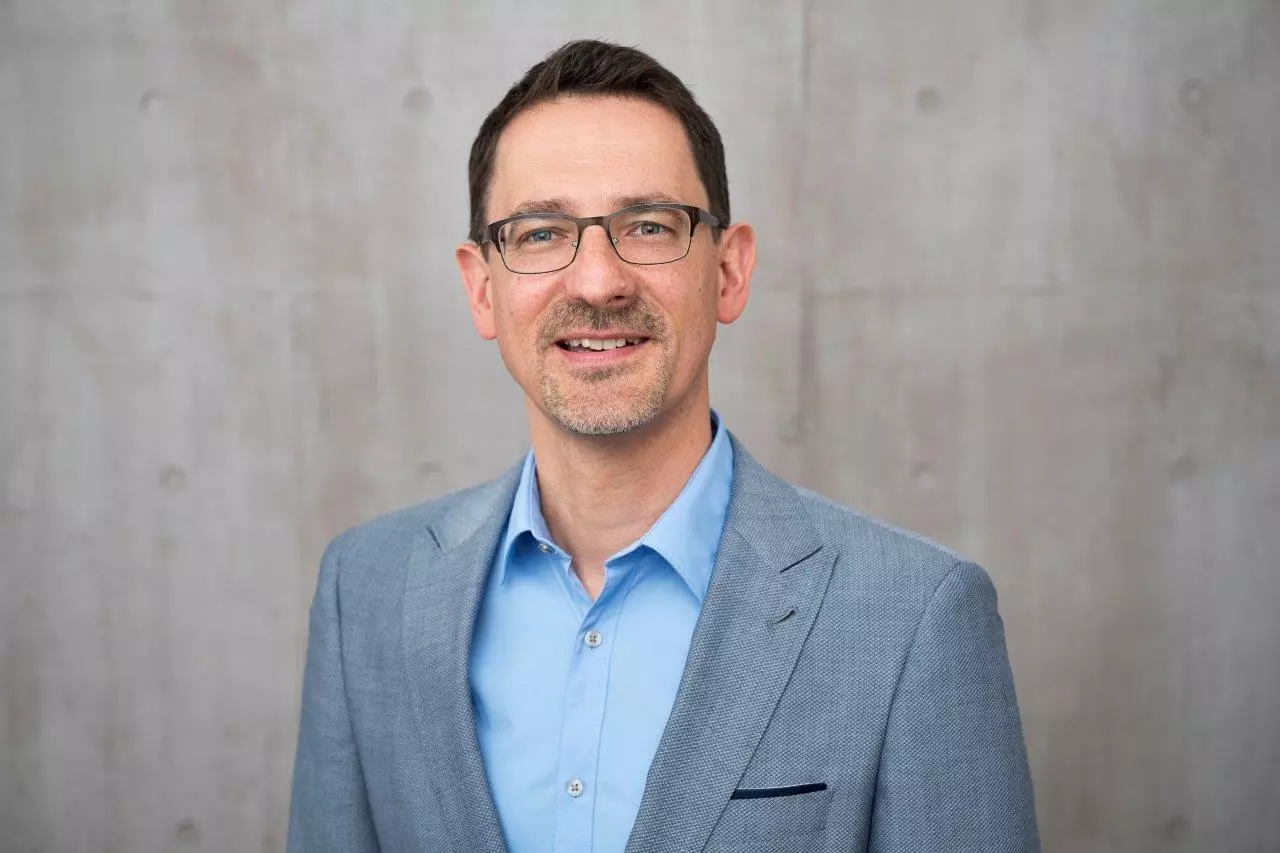
A Brief Biography
Volker Ziebart studied Physics in Cologne, Germany, and holds a PhD of the Swiss Federal Institute of Technology (ETH). He worked in different positions for Phonak und Mettler-Toledo where he especially focused on multi-physics modelling and ultra-high precision measurement techniques. Since 2017 he is with the Applied Complex System Science Group of the Institute of Applied Mathematics and Physics, ZHAW. His current research interest is optimization of building HVAC systems and reinforcement learning control.
Artificial Intelligence for HVAC Systems
Intelligent control systems can achieve significant reductions of energy consumption for heating, ventilation, and air condition (HVAC) of buildings while maintaining the thermal comfort. During the last ten years, Model Predictive Control was studied as optimal control method for HVAC-systems. Although its energy efficiency was demonstrated in many studies, it is currently economically not competitive because of the large effort needed to formulate adequate thermodynamic models for both building and HVAC-system.
We investigate reinforcement learning (RL) as a data driven approach to optimal control for HVAC -systems. This talk presents an approach how to deal with the problem of data inefficiency of RL as well as results and insights from a study in which different RL algorithms were used to control a simulated heating system.
Dr. Willi Brammertz
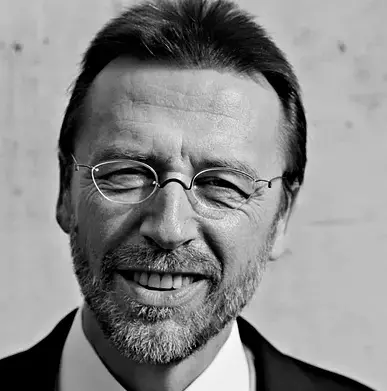
A Brief Biography
Dr. Brammertz obtained his PhD in Economics from the University of Zurich. His doctoral thesis discussed the elementary parts of finance which form the basis of any financial analysis. While maintaining the foundation laid in his thesis, the concepts were continually refined and developed. He co-founded early 1996 Iris ag in Zurich and applied these insights in the analytic platform for banks – riskpro™ – which has been sold to date to more than 300 banks in about forty countries worldwide. The success of riskpro™ derived from its simple core – the idea of Contract Types – which led to an unparalleled clarity of design, consistency and completeness on the analytic level – risk management, finance and regulatory reporting. Iris was sold in 2008. Brammertz published widely in the field and is a speaker at conferences.
Currently Brammertz works on the next logical step which is the Open Source implementation of the standard Contract Types. In this capacity he is chairman of the ACTUS User Association. Parallel to this, he is managing director of ARIADNE, a company specialized on financial analytics especially financial planning.
He also teaches on this subject in a regular masters and PhD course at the University of Zurich/ETH Zürich.
Smart Contracts: The Basic Building Blocks of Future Digital Banks
The basic building block of the banks of the future is the standardized smart financial contract. Such a smart financial contract will support the entire life cycle of financial transaction, from its inception, its processing of the payments to the analytic use case. We will explain the basic principles of the smart financial contract, how it is centrally positioned within the architecture and what will come out of it in terms of processing and analytics such as valuation, risk management, accounting and regulatory reporting.
Prof. Dr. Wolfgang Breymann
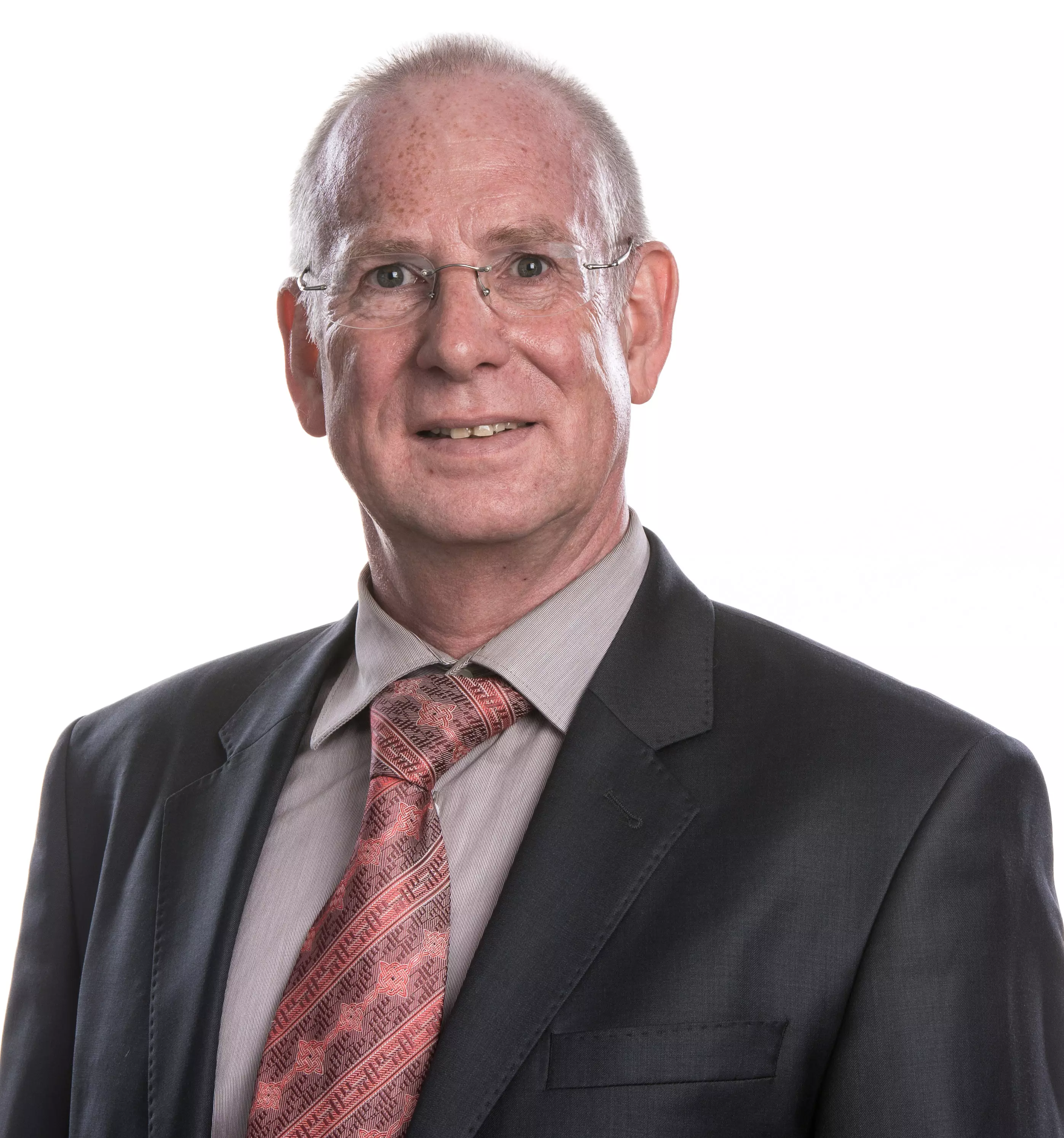
A Brief Biography
Prof. Dr. Wolfgang Breymann is head of the group Finance, Risk Management and Econometrics at Zurich University of Applied Sciences, Institute of Data Analysis and Process Design, which he shaped by developing the research activities in financial markets and risk. After a career in theoretical physics, he turned to finance in 1996 as one of the early contributors to the then burgeoning field of Econophysics and joined ZHAW in 2004. He is one of the originators of project ACTUS for standardizing financial contract modelling and member of the board of directors of the ACTUS Financial Research Foundation as well as founding member of Ariadne Software AG and Ariadne Business Analytics AG. His current R&D interests are focused on the automation of risk assessment to improve the transparency and resilience of the financial system.
Wolfgang Breymann has managed large national and international projects. He authored or co-authored over 40 refereed papers and co-authored the book “Unified Financial Analysis”. He has given many invited talks at universities and conferences all over the world.
A Prototype Environment for Financial Risk Modeling
ACTUS Standard Contract Types have been developed in view of increasing consistency, efficiency and transparency of risk assessment at the level of individual institutions as well as the level of the whole financial system. While real-world applications are quite large and require considerable computational resources, we present here a prototype-like demonstrator that illustrates how these financial contract types can be used for portfolio/balance sheet analysis, stress testing and systemic analysis. The demonstrator uses the ACTUS contract library through an R interface (rACTUS) and the R shiny package for the construction of the GUI.
Individual institutions are represented by simple balance sheets each of which contains only a handful of contracts. The network is represented in form of a graph whose nodes represent key performance indicators of the banks and whose links represent the exposure by means of interbank contracts. The demonstrator provides the possibility to value the positions of the individual institutions, to perform simple stress tests and to investigate cascading effect in a toy financial system. The application is highly configurable. It can be run on a server so that it is accessible through the internet and is in principle scalable if sufficient computing power is available.
Francis Gross
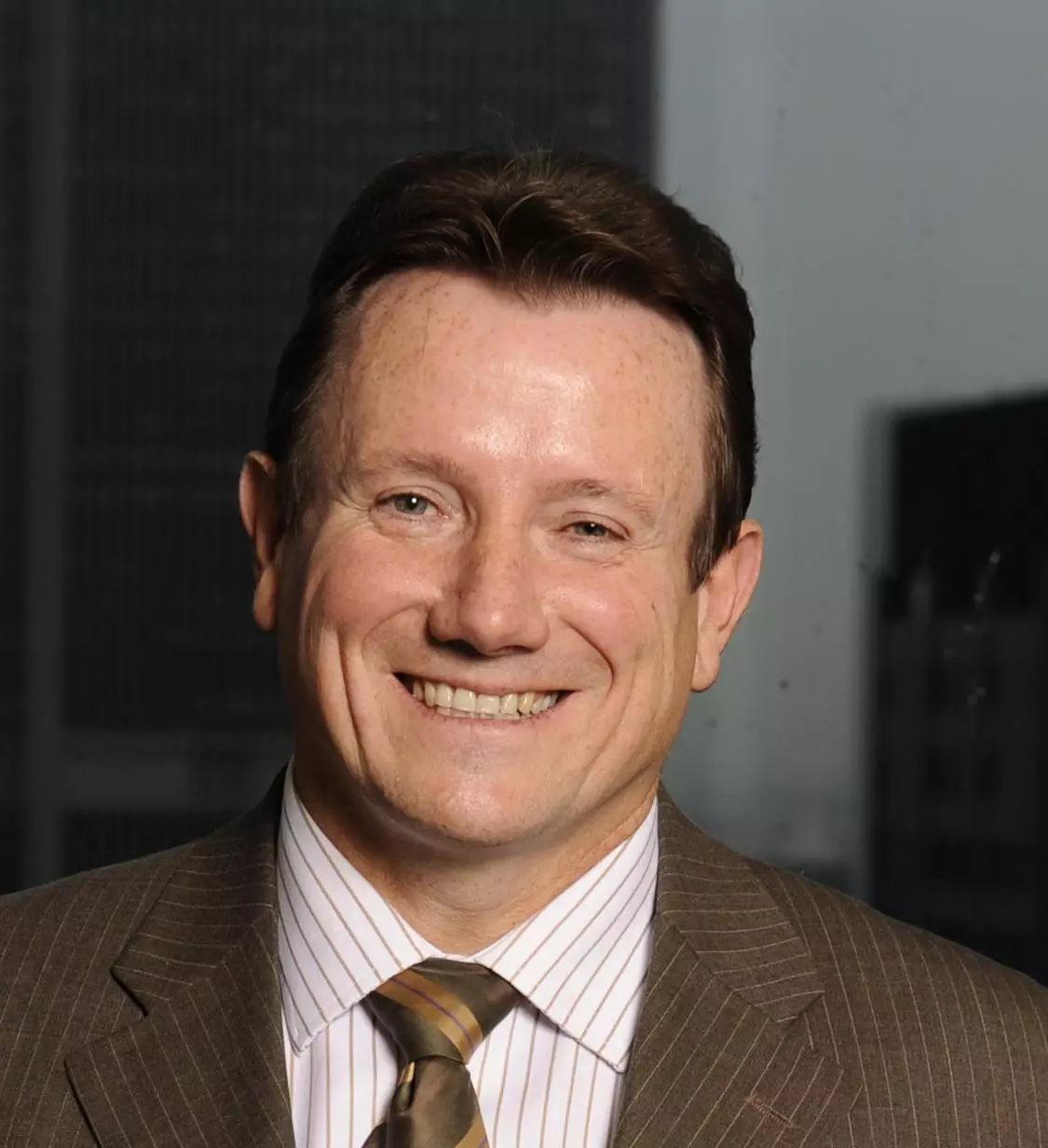
A Brief Biography
Francis Gross is Senior Adviser in the Directorate General Statistics of the European Central Bank. His interests include developing vision and strategy for overcoming the dual disruption of rapid globalization and digitization, as well as designing and driving the implementation of concrete, feasible measures with transformational power to ultimately deliver measurement tools effective at the scale and speed of today’s finance, especially in a crisis. His immediate focus is on the “real world - data world” interface, primarily object identification. He serves on the Regulatory Oversight Committee of the Global LEI System (GLEIS) and has been instrumental in the emergence and development of the GLEIS. Prior to the ECB, Francis spent fifteen years in the automotive industry, eight of which at Mercedes, working mainly on globalisation, strategic alliances and business development. He holds an engineering degree from École Centrale des Arts et Manufactures, Paris, and an MBA from Henley Management College, UK
The economy as a network of contracts connecting a population of parties
Matter is a complex substance, yet the periodic table provided a powerful, simple representation of matter that enabled the development of modern chemistry. All the same, the economy is a complex substance and requires a representation that enables a use of technology that supports efficient and safe operations as well as measurement at the speed and scale of the events the use of technology now enables. The author proposes a vision of the economy as a network of contracts connecting a global population of parties, with each element anchored in the real world’s geographical, legal, political and cultural spaces. He then shows how each contract could be represented as a standardized smart contract on a generalized ACTUS basis, with an algorithm linking parties, assets and events, themselves represented in a suite of distributed ledgers. Finally it is shown how in a digitized world such an infrastructure could be used to support efficient and safe operations in the economy, as well as providing measurement at the speed and scale of the system while strongly reducing the need for reporting. It also shows how economic and financial analysis would evolve in such an environment.
Prof. Dr. Petros Kavassalis
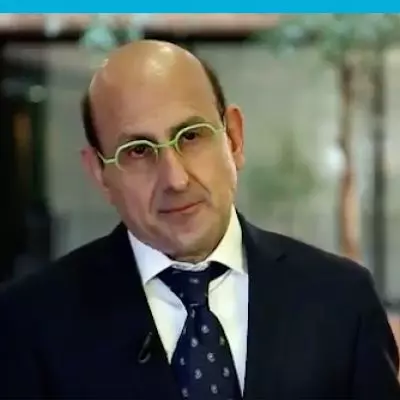
A Brief Biography
Petros Kavassalis is Associate Professor with the University of the Aegean (Dpt of Financial and Management Engineering – Information Management Lab, i4M Lab) and Senior Researcher at CTIP (Computer Technology Institute & Press - Research Unit 9, RU 9), Greece. Petros Kavassalis holds a degree in Civil Engineering from the National Technical University of Athens (NTUA) and a Ph.D. from Dauphine University in Paris (Economics and Management). In the past, he worked as a Researcher at the Ecole Polytechnique, Paris (Centre de Recherche en Gestion), at MIT (Research Program on Communication Policy – now part of SSRC), where he has contributed to the foundation of the MIT Internet Telecommunications Convergence Consortium MIT-ITC, and at ICS-Forth, Greece. His interests focus on the fields of Information Management, Identity and Privacy Management in Federated Environments, Business Process Modeling and Automation, Document Engineering, Communications Policy, Organization of the Digital Economies, Economics and Policy of Industrial and Technical Change. In the last years, he served as: a) Principal Investigator for LGAF Project, implementing “BPM over SOA” solutions for e-government purposes, b) Member of the Core Technical Team of STORK 2.0 EU Project and, c) Information architect for a number of recent projects on privacy management and Distributed Ledger Technologies, including a SmartRegTech draft project promoting an ultra-distributed system built for the needs of the financial risk reporting and monitoring.
Blockchain and Financial Risk Reporting: design principles and formal reasoning
How one can use ultra distributed technologies, such as the Distributed Ledger Technologies (DLTs) / aka blockchain, to improve the efficiency and transparency of financial reporting processes, of current processes as well as of those prescribed by MiFID II/MiFIR standards? Where DLTs meet ACTUS standards? How their combination can improve the financial reporting quality and create a powerful tool early appreciation of financial risks, and for increased transparency through the participation of the "society at large" in the supervision of the financial system? What is the experience of using DLTs in other related domains of economic activity? How stable and mature are these technologies and what are the lessons learned from the use of permission blockchains in relatively similar processes of distributed information capturing and sharing? These the questions, the proposed paper addresses in a "frame the debate" and concepts validation context.
The Honorable Allan I. Mendelowitz
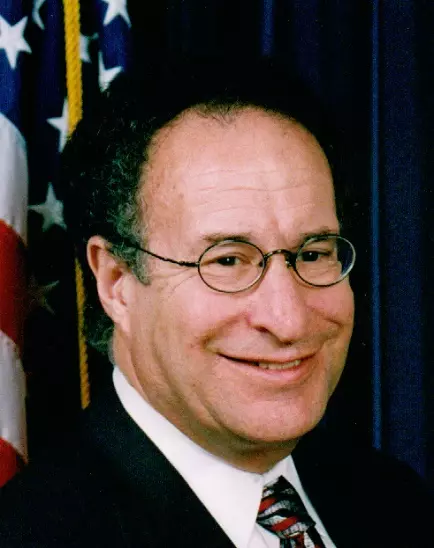
A Brief Biography
Allan Mendelowitz is the President of the ACTUS Financial Research Foundation, a not-for-profit corporation creating an open-source algorithmic data standard for financial instrument. After the 2008 financial crisis he was a founding member and the co-leader of the Committee to Establish the National Institute of Finance. In this capacity he took the lead in formulating the committee’s legislative strategy and drafting the legislation that created the Office of Financial Research. Previously he served on the Board of Directors of the Federal Housing Finance Board from 2000 to 2009, and he was as the board's chairman from 2000 to 2001. His other positions have included: strategic advisor, Deloitte Consulting; executive director of the U.S. Trade Deficit Review Commission (a congressionally appointed bipartisan panel); and executive vice president of the Export-Import Bank of the United States. From 1981 to 1995, Dr. Mendelowitz was the managing director for international trade, finance, and economic competitiveness at the U.S. GAO. In 1980 he was the senior economist on the Chrysler Corporation Loan Guarantee Board where he was responsible for a broad array of analysis, negotiating key provisions of the transaction, and was personally and directly responsible for the inclusion of warrants in the transaction which netted the taxpayers a return of $310 million. He was formerly an economic policy fellow at the Brookings Institution and on the faculty of Rutgers University, where he taught courses in international finance and trade and urban and regional economics. His articles have appeared in the Journal of Risk Finance, Risk Professional, the Journal of Business, the National Tax Journal, the Journal of Policy Analysis and Management, the Financial Times, Waters, the American Banker, and other publications and proceedings volumes. He has also testified as an expert witness before committees of the U.S. Congress 150 times. His education includes economics degrees from Columbia University (A.B.) and Northwestern University (M.A., Ph.D.).
Regulation 2.0: Stress Tests and Oversight of Financial Risk
The 2008 financial crisis revealed how little regulators understood about the condition of financial institutions and the risks of a systemic crisis. Since that crisis improvements in oversight have been adopted. Principle among them has been the institution of regulator-mandated stress tests. Nevertheless, there remains room for significant improvement. Current stress tests are very costly, they take place only annually, they do not enable comparisons between different banks, and they do not make it possible to see the risks of cascading failures. The collection of granular transaction and position data in a data standard that supports financial analysis would make it possible to reduce the costs of stress tests and improve the value of the analytical results by addressing the current weaknesses. This paper will discuss how the ACTUS standard would make these improvement possible.
Marc Sel
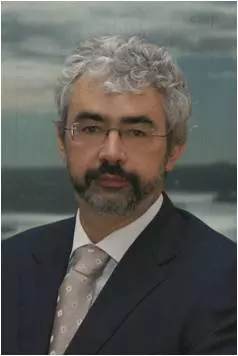
A Brief Biography
Marc Sel is working for PricewaterhouseCoopers 'Advisory Services' in Belgium as a Director, specialised in ICT Security and Performance. He joined the firm in January 1989 as a Consultant. Over time, he specialised in the field of security, both from the technical and from the organisational/management perspective. He performed specialised in-depth reviews, assisted clients with the selection of solutions, and performed implementations. Areas he worked in include authorisations and access control, biometric recognition, network security, PKI, smartcards, as well as information security organisation legislation and policies, standards and guidelines. For details please visit www.marcsel.eu.
Prior to PwC he was with Esso, where he worked for two years as a Systems Programmer on IBM mainframes, and two years as a Technical Analyst in the Breda Headquarters. He moved to Esso after a stay of approximately 4 years with Bell Telephone Manufacturing Company, where he developed and delivered training courses on all aspects of digital telephony. Initially after my first graduation he joined Texas Instruments as Internal Sales Engineer in their Brussels semiconductor department for 18 months.
How smart contracts can implement the policy objective of 'report once'
The presentation will discuss a demonstrator built for DG FISMA and show how smart contracts can implement 'digital doppelgängers' of OTC derivatives contracts that are traded. The technology is based on an Ethereum private blockchain, with smart contracts written in Solidity. The demonstrator is one of the outcomes of a study performed by PwC and Claryon that explored possibilities to address the problems of cost of regulatory burdens for banks and financial institutions and inadequate monitoring of risk attached to financial contracts.
The study investigated the feasibility of Distributed Ledger Technologies (DLTs) as the new way of financial institutions to meet their reporting obligations as laid down in EU financial
sector legislation. The novelty is that the resulting demonstrator illustrates the policy objective of implementing a "report once" approach in financial reporting (EMIR, MIFIR, COREP) in the EU using smart contracts.
The demonstrator addresses three stories, performed by actors that all participate in a blockchain. Alice, Bob and Eve are contracting parties, Romeo is a regulator, and the Narrator tells the stories.
Story 1: Bond, where Alice lends money to Bob and later merges with Eve to form AliceEve
Story 2: Interest Rate Swap, where Bob creates contract towards AliceEve and creates a new Interest Rate Swap contract with her
Story 3: AliceEve defaults (stops paying)on the swap contract
Prof. Dr. Kurt Stockinger
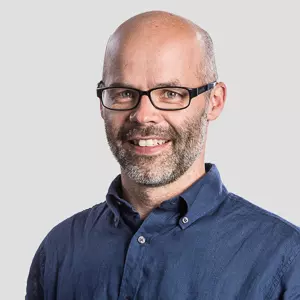
A Brief Biography
Prof. Dr. Kurt Stockinger is Professor of Computer Science and Director of Studies, Data Science at Zurich University of Applied Sciences. His research focuses on Data Science with emphasis on Big Data, data warehousing, business intelligences and advanced analytics. He is also on the Advisory Board of Callista Group AG. Previously Kurt Stockinger worked at Credit Suisse, at Lawrence Berkeley National Laboratory in Berkeley, at California Institue of Technology, as well as at CERN. He holds a Ph.D. in computer science from CERN / University of Vienna.
Data-Driven Financial Risk Modeling at Scale with Apache Spark
Highly volatile financial markets and heterogeneous data sets within and across banks as well as financial services institutions world-wide make near real-time financial analytics and risk assessment very challenging such that cutting edge financial algorithms are needed. Moreover, large financial organizations have hundreds of millions of financial contracts (financial instruments) on their balance sheet, turning these financial calculations into a true big data problem. However, due to the lack of standards, current financial algorithms for calculating aggregate risk exposures are typically inconsistent and non-scalable. Addressing these limitations, Ariadne Business Analytics AG offers a financial analytics platform which builds on the ACTUS financial instruments standard
for data and algorithms. In this talk we will present a data-driven, distributed financial simulation engine built with Apache Spark that has been developed in a joint research project between Ariadne and Zurich University of Applied Sciences. In particular, we will discuss early results of large-scale simulation experiments with real financial contract data and provide detailed insights into how to build such a scalable big data system with state-of-the-art Apache Spark technology. We round up our talk with best practices and pitfalls of using big data technology at massive scale.
Participants in the Panel Discussion "Next Steps for Real-World Solutions"
Sam Chadwick, Director of Strategy and Innovation, Thomson Reuters
Sam is responsible for several fintech innovation initiatives within the Financial & Risk business of Thomson Reuters. He has worked for the company in product strategy and product management roles for 15 years in New York, Hong Kong and Switzerland. Sam has a Masters in Financial Strategy from the University of Oxford and a Masters in Engineering from the University of Bristol. Sam currently lives in Zug where he is focused on alternative finance, cryptocurrencies, blockchain technology and artificial intelligence. Sam is also a founding board member of the Crypto Valley Association.
Arie Levy-Cohen
Mr. Levy-Cohen is a thought leader in the field of generational wealth preservation and tail risk management, a follower of capital flow analysis and economic cycles, with a keen eye for emerging trends and technologies.
For more than 8 years Arie worked at Morgan Stanley as International Client Advisor and Private Banker running both The Levy-Hafen and The WMO Groups. As a dual licensed Financial Advisor with both Morgan Stanley Wealth Management and Morgan Stanley Private Bank N.A., Arie serviced institutional and ultra-high net worth clients. He also served as an investment advisor to think tanks, technology incubators and Blockchain companies and single-family offices. Since 2010, Arie has been actively involved with various distributed ledger initiatives, advising start-ups and speaking at multiple FinTech conferences. He also co-moderated the Morgan Stanley Bitcoin Forum.
Currently Arie is Founder & CEO of Blockhaus Investment, AG, and Co-Founder & President of SingularDTV, GmbH, and VP Strategy to Monetary Metals. He is also deeply involved in FinTech serving as an Invited Expert with the World Wide Web Consortium (W3C) and advisor to select Blockchain companies. Special focus on Identity, cryptographic security, risk and compliance (KYC\AML) and Tokenized Ecosystems.
Arie is primarily focused in the Blockchain (FinTech) industry whilst maintaining his roots as a fully licensed wealth management advisor, international private banker and in precious metals.
Dr. George Williams
George M. Williams jr is a financial regulatory attorney at the New York office of King & Spalding LLP, which advises the ACTUS Financial Research Foundation and the ACTUS Users Association. In addition to activities relating to the licensing and compliance structure of institutions, money and asset transmission, the treatment of commodity interests, and lending, his interests include the way systemic risk in the financial system is treated analytically and legally and the effects of technology on the financial and legal systems. In addition to his law degree, he has a PhD in Linguistics from MIT.
Registration
Please find here the form to register for the «3rd European COST Conference on Artificial Intelligence in Industry and Finance».
Location
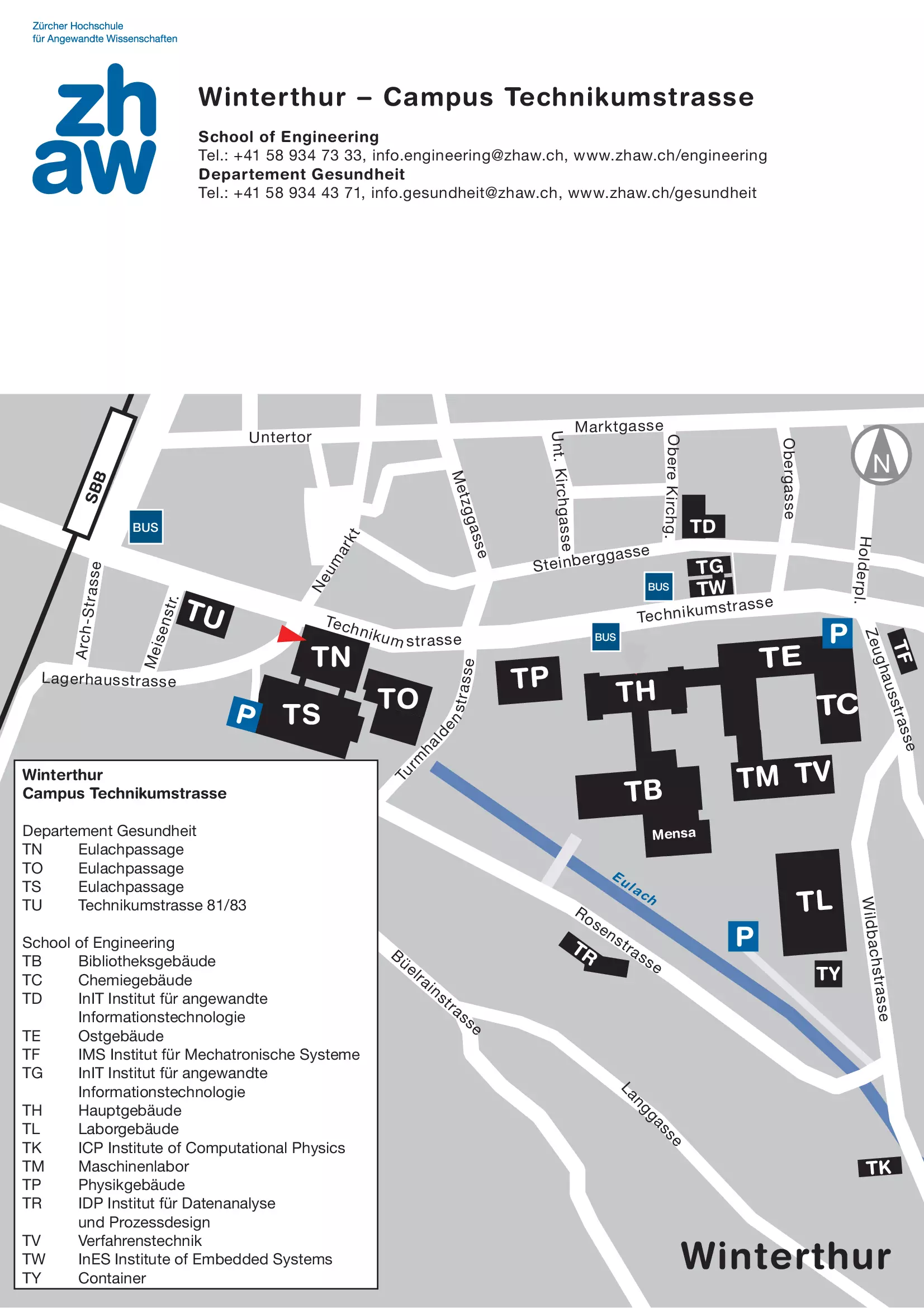
The conference venue is situated at a walking distance from the station.
Travel to ZHAW School of Engineering, Winterthur
From the Zürich airport local transport brings you to the centre of Winterthur in just 20 minutes.
The conference takes place in a short walking distance to the train station. Numerous hotels in the immediate vicinity will ensure a pleasant stay in Winterthur
By train
The trains run as often as every 15 to 20 minutes from Zürich Airport and Zürich City and take about 15 minutes to arrive in Winterthur.
From the main railway-station, the conference location can be reached within a walking distance of less than 5 minutes.
By Car
Location
Organizing Committee
- Prof. Dr. Jörg Osterrieder
- Prof. Dr. Dirk Wilhelm
- Prof. Dr. Rudolf Füchslin
- Dr. Andreas Henrici
- Prof. Dr. Peter Schwendner
Program Comittee
- Prof. Dr. Jörg Osterrieder
- Prof. Dr. Dirk Wilhelm
- Prof. Dr. Wolfgang Breymann
- Prof. Dr. Ruedi Füchslin
- Dr. Andreas Henrici
- Prof. Dr. Peter Schwendner
- Prof. Dr. Marc Wildi
- Dr. Thilo Stadelmann