Intelligent Vision Systems Group
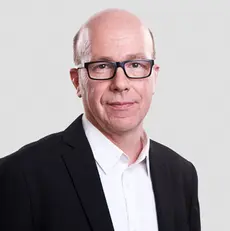
"We aim at advancing the state of the art in AI, Deep Learning and Machine Learning research, while at the same time developing tailored solutions to real-world challenging problems which help to advance technology and benefit humanity."
Fields of expertise
- Computer Vision
- Machine Learning Systems (MLOps)
- Trustworthy and certifiable AI
We conduct research primarily in the domain of computer vision using 2-,3- or 4-D image or video data as input and performing classification, object detection or other visual tasks, for which we develop state of the art deep neural network architectures. We are particularly interested in recent developments including vision transformers and gauge equivariant neural networks. Domains of applications include, but are not limited to, industrial quality control, medical imaging and diagnosis (computed tomography), as well as earth (satellites) and sky (radio-astronomy) observation data. We are also interested in hybrid approaches to AI as well as geometric deep learning. Our second main area of interest concerns MLOps, which describes best practices for building complete, production-ready and scalable Machine Learning systems. Finally, we are interested in methods to create safe, trustworthy and certifiable AI systems, which comply with current and future legislation.
Services
- Insight: keynotes, trainings
- AI consultancy: workshops, expert support, advise, technology assessment
- Research and development: small to large-scale research projects, third party-funded research, student projects, commercially applicable prototypes
Team
Head of Research Group
Projects
Unfortunately, no list of projects can be displayed here at the moment. Until the list is available again, the project search on the ZHAW homepage can be used.
Publications
-
Bonaldi, A.; Hartley, P.; Braun, R.; Purser, S.; Acharya, A.; Ahn, K.; Resco, M. Aparicio; Bait, O.; Bianco, M.; Chakraborty, A.; Chapman, E.; Chatterjee, S.; Chege, K.; Chen, H.; Chen, X.; Chen, Z.; Conaboy, L.; Cruz, M.; Darriba, L.; De Santis, M.; Denzel, P.; Diao, K.; Feron, J.; Finlay, C.; Gehlot, B.; Ghosh, S.; Giri, S. K.; Grumitt, R.; Hong, S. E.; Ito, T.; Jiang, M.; Jordan, C.; Kim, S.; Kim, M.; Kim, J.; Krishna, S. P.; Kulkarni, A.; López-Caniego, M.; Labadie-García, I.; Lee, H.; Lee, D.; Lee, N.; Line, J.; Liu, Y.; Mao, Y.; Mazumder, A.; Mertens, F. G.; Munshi, S.; Nasirudin, A.; Ni, S.; Nistane, V.; Norregaard, C.; Null, D.; Offringa, A.; Oh, M.; Oh, S. -H.; Parkinson, D.; Pritchard, J.; Ruiz-Granda, M.; López, V. Salvador; Shan, H.; Sharma, R.; Trott, C.; Yoshiura, S.; Zhang, L.; Zhang, X.; Zheng, Q.; Zhu, Z.; Zuo, S.; Akahori, T.; Alberto, P.; Allys, E.; An, T.; Anstey, D.; Baek, J.; Basavraj; Brackenhoff, S.; Browne, P.; Ceccotti, E.; Chen, H.; Chen, T.; Choudhuri, S.; Choudhury, M.; Coles, J.; Cook, J.; Cornu, D.; Cunnington, S.; Das, S.; Acedo, E. De Lera; Delou is, J. -M.; Deng, F.; Ding, J.; Elahi, K. M. A.; Fernandez, P.; Fernández, C.; Alcázar, A. Fernández; Galluzzi, V.; Gao, L. -Y.; Garain, U.; Garrido, J.; Gendron-Marsolais, M. -L.; Gessey-Jones, T.; Ghorbel, H.; Gong, Y.; Guo, S.; Hasegawa, K.; Hayashi, T.; Herranz, D.; Holanda, V.; Holloway, A. J.; Hothi, I.; Höfer, C.; Jelić, V.; Jiang, Y.; Jiang, X.; Kang, H.; Kim, J. -Y.; Koopmans, L. V.; Lacroix, R.; Lee, E.; Leeney, S.; Levrier, F.; Li, Y.; Liu, Y.; Ma, Q.; Meriot, R.; Mesinger, A.; Mevius, M.; Minoda, T.; Miville-Deschenes, M. -A.; Moldon, J.; Mondal, R.; Murmu, C.; Murray, S.; SR, Nirmala; Niu, Q .; Nunhokee, C.; O'Hara, O.; Pal, S. K.; Pal, S.; Park, J.; Parra, M.; tra, N. N. Pa; Pindor, B.; Remazeilles, M.; Rey, P.; Rubino-Martin, J. A.; Saha, S.; Selvaraj, A.; Semelin, B.; Shah, R.; Shao, Y.; Shaw, A. K.; Shi, F.; Shimabukuro, H.; Singh, G.; Sohn, B. W.; Stagni, M.; Starck, J. -L.; Sui, C.; Swinbank, J. D.; Sánchez, J.; Sánchez-Expósito, S.; Takahashi, K.; Takeuchi, T.; Tripathi, A.; Verdes-Montenegro, L.; Vielva, P.; Vitello, F. R.; Wang, G. -J.; Wang, Q.; Wang, X.; Wang, Y.; Wang, Y. -X.; Wiegert, T.; Wild, A.; Williams, W. L.; Wolz, L.; Wu, X.; Wu, P.; Xia, J. -Q.; Xu, Y.; Yan, R.; Yan, Y. -P.,
2025.
Square Kilometre Array Science Data Challenge 3a : foreground removal for an EoR experiment.
arXiv.
Available from: https://doi.org/10.48550/arxiv.2503.11740
-
Frischknecht-Gruber, Carmen; Denzel, Philipp; Forster, Oliver; Billeter, Yann; Iranfar, Arman; Repetto, Marco; Reif, Monika Ulrike; Schilling, Frank-Peter; Weng, Joanna; Chavarriaga, Ricardo,
2025.
In:
AI Days @ HES-SO, Geneva and Lausanne, Switzerland, 27–29 January 2025.
ZHAW Zürcher Hochschule für Angewandte Wissenschaften.
Available from: https://doi.org/10.21256/zhaw-32422
-
Frischknecht-Gruber, Carmen; Denzel, Philipp; Reif, Monika; Billeter, Yann; Brunner, Stefan; Forster, Oliver; Schilling, Frank-Peter; Weng, Joanna; Chavarriaga, Ricardo; et al.,
2025.
AI assessment in practice : implementing a certification scheme for AI trustworthiness[paper].
In:
Görge, Rebekka; Haedecke, Elena; Poretschkin, Maximilian; Schmitz, Anna, eds.,
Symposium on Scaling AI Assessments (SAIA 2024).
Symposium on Scaling AI Assessments (SAIA 2024), Cologne, Germany, 30 September - 1 October 2024.
Schloss Dagstuhl – Leibniz-Zentrum für Informatik.
pp. 15:1-15:18.
Open Access Series in Informatics (OASIcs) ; 126.
Available from: https://doi.org/10.4230/OASIcs.SAIA.2024.15
-
Billeter, Yann; Denzel, Philipp; Chavarriaga, Ricardo; Forster, Oliver; Schilling, Frank-Peter; Brunner, Stefan; Frischknecht-Gruber, Carmen; Reif, Monika Ulrike; Weng, Joanna,
2024.
MLOps as enabler of trustworthy AI[paper].
In:
2024 11th IEEE Swiss Conference on Data Science (SDS).
11th IEEE Swiss Conference on Data Science (SDS), Zurich, Switzerland, 30-31 May 2024.
IEEE.
pp. 37-40.
Available from: https://doi.org/10.1109/SDS60720.2024.00013
-
Denzel, Philipp; Brunner, Stefan; Billeter, Yann; Forster, Oliver; Frischknecht-Gruber, Carmen; Reif, Monika Ulrike; Schilling, Frank-Peter; Weng, Joanna; Chavarriaga, Ricardo; Amini, Amin; Repetto, Marco; Iranfar, Arman,
2024.
Towards the certification of AI-based systems[paper].
In:
2024 11th IEEE Swiss Conference on Data Science (SDS).
11th IEEE Swiss Conference on Data Science (SDS), Zurich, Switzerland, 30-31 May 2024.
IEEE.
pp. 84-91.
Available from: https://doi.org/10.1109/SDS60720.2024.00020