6th European COST Conference on Artificial Intelligence in Industry and Finance
We would like to welcome you to the «6th European COST Conference on Artificial Intelligence in Industry and Finance», hosted by the Institute of Applied Mathematics and Physics (IAMP) and the Institute of Data Analysis and Process Design (IDP) at the School of Engineering, and the Institute of Wealth & Asset Management (IWA) at the School of Management and Law of the Zurich University of Applied Sciences (ZHAW) in Winterthur, Switzerland.
Artificial Intelligence in Industry and Finance (6th European COST Conference on AI in Industry and Finance in Switzerland)
September 9, 2021, 12:30-17:15 - Online Conference
Information at a glance
- Conference Date: Thursday, September 9, 2021
- Conference Location: online
- Conference Topics: Artificial Intelligence in Industry and Finance
- Conference Flyer(PDF 303,8 KB)
- Conference Program(PDF 323,1 KB)
- Program booklet(PDF 1,5 MB)
- Related Journal: "Frontiers in Artificial Intelligence"(PDF 266,5 KB)
- Funding: This conference is funded by the Innosuisse (Swiss Innovation Agency) Networking Event Series, and is an event in the Networking Event Series – Artificial Intelligence in Industry and Finance.
Zoom Links
- Introduction and keynote: ID: 965 8228 0075: https://zhaw.zoom.us/j/96582280075
- Networking stage during the breaks: https://www.wonder.me/r?id=9bbe24ca-e619-4d93-a1b7-8517d3012800
- AI in Finance: https://zhaw.zoom.us/j/61074149347
- AI in Industry: https://zhaw.zoom.us/j/61438609327
- Ethical Questions in AI: https://zhaw.zoom.us/j/67914306899
- Use Cases / Best Practice: https://zhaw.zoom.us/j/63385452379
- Closing Panel: ID: 965 8228 0075 https://zhaw.zoom.us/j/96582280075
Aim of the conference
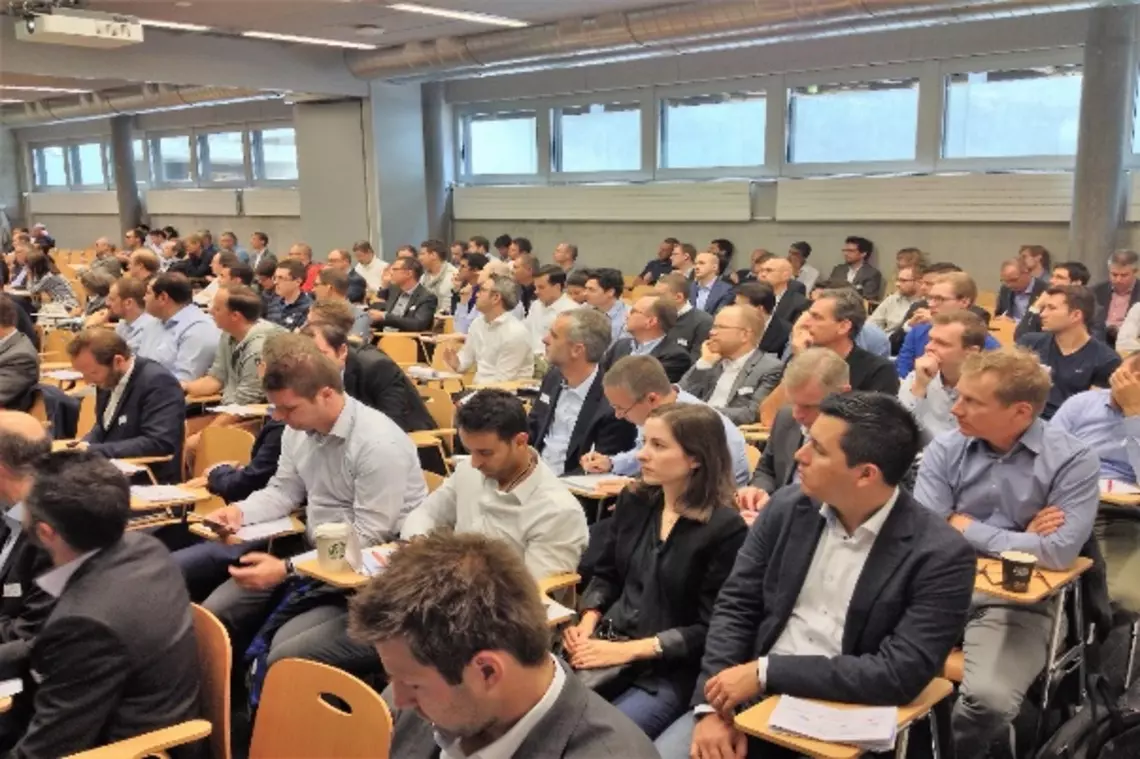
The aim of this conference is to bring together academics, young researchers, students and industrial practitioners to discuss the application of Artificial Intelligence in Industry and Finance.
The 1st COST Conference was held on September 15, 2016, the 2nd COST Conference was held on September 7, 2017, the 3rd COST Conference was held on September 6, 2018, the 4th AI Conference was held on September 5, 2019, and the 5th COST Conference was held on September 3, 2020.
Related Conferences
- Intelligent Maintenance Conference 2021 (September 7/8, 2021, Zürich)
- 4. Konferenz Perspektiven mit Industrie 4.0 (June 6, 2021, Winterthur)
Thematic Sessions
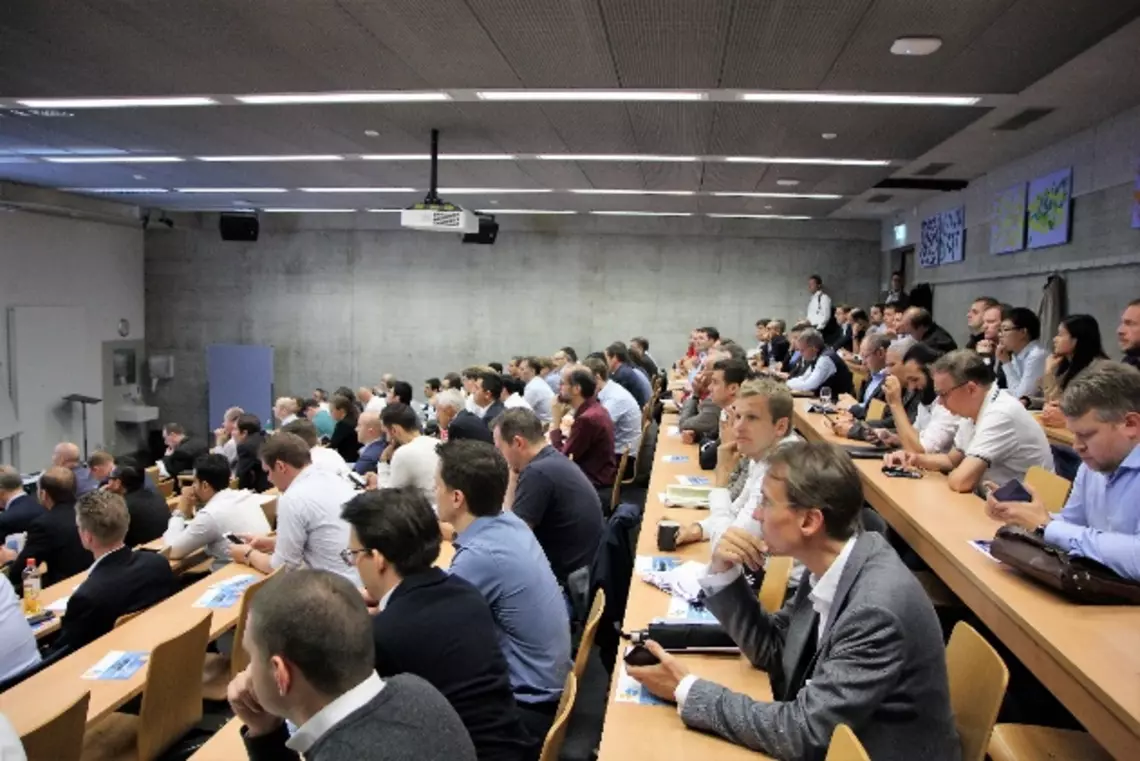
- Artificial Intelligence in Finance: Artificial Intelligence and Fintech challenges for the banking and insurance industry
- Artificial Intelligence in Industry: Artificial Intelligence challenges for companies from the mechanical and electrical industry, but also life sciences.
- Ethical Questions in Artificial Intelligence: Issues arising in AI applications such as trust, explainability, neutrality, responsibility, moral consequences of algorithmic decisions.
- Academia-Industry Best Practices: General discussion of issues arising in academia-industry cooperations, and the role of Innosuisse in supporting these cooperations
Invited Speakers
Artificial Intelligence in Finance
- Prof. Dr. Dirk Eddelbuettel, TileDB: "Using R for Machine Learning in a Multi-Lingual World"
- Prof. Dr. Josef Teichmann, ETHZ: "Model free Deep Hedging"
- Dr. Miquel Noguer i Alonso, AI Finance Institute: "A Meta-Learning approach to Model Uncertainty in Financial Time Series"
- Dr. Jochen Spuck & Dr. Alex Posth, Ecosight / ZHAW: "Thematic Investing in GreenTech: Patent-data-driven Identification of Green Technology Trends and Sustainable Investment Opportunities" (recording)
- Prof. Dr. Natalie Packham, HWR Berlin: "Correlation scenarios and correlation stress testing" (slides(PDF 1,6 MB) / recording)
- Prof. Dr. Peter Schwendner, ZHAW: "Interpretable Machine Learning for Diversified Portfolio Construction" (slides(PDF 2,8 MB) / recording)
Artificial Intelligence in Industry
- Dr. Pascal Paysan, Varian Medical Systems: "Applications for AI in Image Formation for Radiation Therapy at Varian"
- Dr. Iason Kastanis, CSEM: "Deep Learning for quality inspection of injection molding parts"
- Dr. Andreas Fitze, Swisscognitive: "Data explosion, but all hidden in your data centre"
- Michael Berns, PWC: "AI Superpowers for Sustainability - how do we leverage AI-driven insights to speed up our climate change strategy?"
- Dr. Stefan Pauli, VTU Engineering: "Machine learning in the GMP regulated industries"
Ethical Questions in Artificial Intelligence
- Dr. Teresa Scantamburlo, Univ. Venezia: "Surveying the Opinion of European Citizens on AI"
- Joachim Baumann & Dr. Christoph Heitz, ZHAW: "Fairness of insurance premiums: May personalized risk models lead to discrimination and social injustice?"
- Dr. Michael Karner, Virtual Vehicle Research GmbH: "Bringing Internet of Things and Artificial Intelligence together – but is it trustworthy?"
- Dr. Gabriele Bolek-Fügl, Compliance 2b GmbH: "Definition of Wrong"
- Dr. Branka Hadji Misheva, ZHAW: "Explainable AI in Finance"
Best practice / Use cases
- Prof. Dr. Dirk Wilhelm, ZHAW: Overview
- Prof. Dr. Galena Pisoni, University of Côte d’Azur: "Innovative Big Data and AI-Empowered Solutions for Financial Companies"
- Dr. Gabriele Schwarz, Innosuisse: "Get support for your innovation project: Innosuisse mentoring"
- Prof. Dr. Patricia Deflorin, FHGR: Open Innovation for Industry 4.0
- Prof. Dr. Jörg Osterrieder, ZHAW: "European Cooperation in Fintech and Artificial Intelligence in Finance"
- Jürgen Büscher, QCAM: "Currency overlay trading strategies"
Closing panel: Automation of Journalism
- Prof. Dr. Guido Keel, Director Institute of Applied Media Studies, ZHAW (Moderation)
- Stefan Trachsel, "Data & Automation Specialist" at CH Media
- Johanna Wild, Open Source Investigator at Bellingcat
- Dr. Jessica Kunert, Wissenschaftliche Mitarbeiterin, Journalistik und Kommunikationswissenschaft, Universität Hamburg
Participants
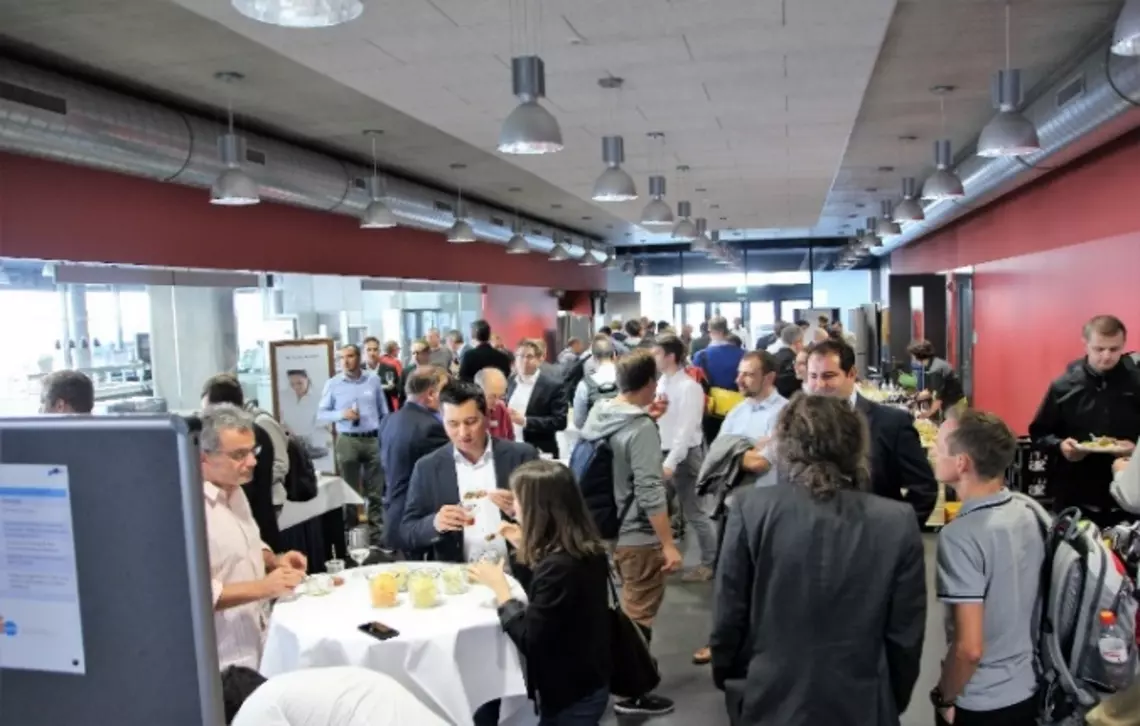
In 2017, 2018, and 2019, we had around 200 participants, both from Academia and Industry. The 2019 instalment of the AI conference also saw a large number of international guests and speakers, travelling to Switzerland from destinations such as the UK, Germany, the United States and Bulgaria. In 2020, we had around 350 online participants and speakers from all around the world.
The largest proportion of participants will come from the industry complemented by a significant number of academic researchers. This mirrors our unique approach of connecting the academic world to their respective fields of application, putting new exciting concepts to work in industrial frameworks, where they can open up new opportunities.
Schedule
- Warm-up: 12:30-13:00
- Welcome: 13:00-13:10
- Keynote: 13:10-13:40
- Break: 13:40-14:00
- Thematic Sessions Part 1: 14:00-15:00. AI in Finance, AI in Industry, Ethical Questions, Best practice/use cases
- Break: 15:00-15:30
- Thematic Sessions Part 2: 15:30-16:30. AI in Finance, AI in Industry, Ethical Questions, Best practice/use cases
- Break: 16:30-16:45
- Final panel: 16:45-17:15
Detailed Schedule
12:30-13:00 | Warm-up | |||
13:00-13:10 | Intro: D. Wilhelm: "Welcome and Introduction" | |||
13:10-13:40 | Keynote: A. Curioni: "What's Next in AI" | |||
13:40-14:00 | Break | |||
AI in Finance 1 | AI in Industry 1 | Ethical Questions 1 | Best practice 1 | |
14:00-14:20 | D. Eddelbuettel: "Using R for Machine Learning in a Multi-Lingual World" | P. Paysan: "Applications for AI in Image Formation for Radiation Therapy at Varian" | T. Scantamburlo: "Surveying the Opinion of European Citizens on AI" | D. Wilhelm: "Overview" |
14:20-14:40 | J. Teichmann: "Model free Deep Hedging" | I. Kastanis: "Deep Learning for quality inspection of injection molding parts" | J. Baumann & C. Heitz: "Fairness of insurance premiums: May personalized risk models lead to discrimination and social injustice?" | G. Pisoni: "Innovative Big Data and AI-Empowered Solutions for Financial Companies" |
14:40-15:00 | M. Noguer: "A Meta-Learning approach to Model Uncertainty in Financial Time Series" | A. Fitze: "Data explosion, but all hidden in your data centre" | M. Karner: "Bringing Internet of Things and Artificial Intelligence together – but is it trustworthy?" | G. Schwarz: "Get support for your innovation project: Innosuisse mentoring" |
15:00-15:30 | Break | |||
AI in Finance 2 | AI in Industry 2 | Ethical Questions 2 | Best practice 2 | |
15:30-15:50 | J. Spuck & A. Posth: "Thematic Investing in GreenTech: Patent-data-driven Identification of Green Technology Trends and Sustainable Investment Opportunities" | M. Berns: "AI Superpowers for Sustainability - how do we leverage AI-driven insights to speed up our climate change strategy?" | G. Bolek-Fügl: "Definition of Wrong" | P. Deflorin: "Open Innovation for Industry 4.0" |
15:50-16:10 | N. Packham: "Correlation scenarios and correlation stress testing" | S. Pauli: "Machine learning in the GMP regulated industries" | B. Hadji Misheva: "Explainable AI in Finance" | J. Osterrieder: "European Cooperation in Fintech and Artificial Intelligence in Finance" |
16:10-16:30 | P. Schwendner: "Interpretable Machine Learning for Diversified Portfolio Construction" | J. Büscher: "Currency overlay trading strategies" | ||
16:30-16:45 | Break | |||
16:45-17:15 | Closing Panel | |||
Dr. Alessandro Curioni
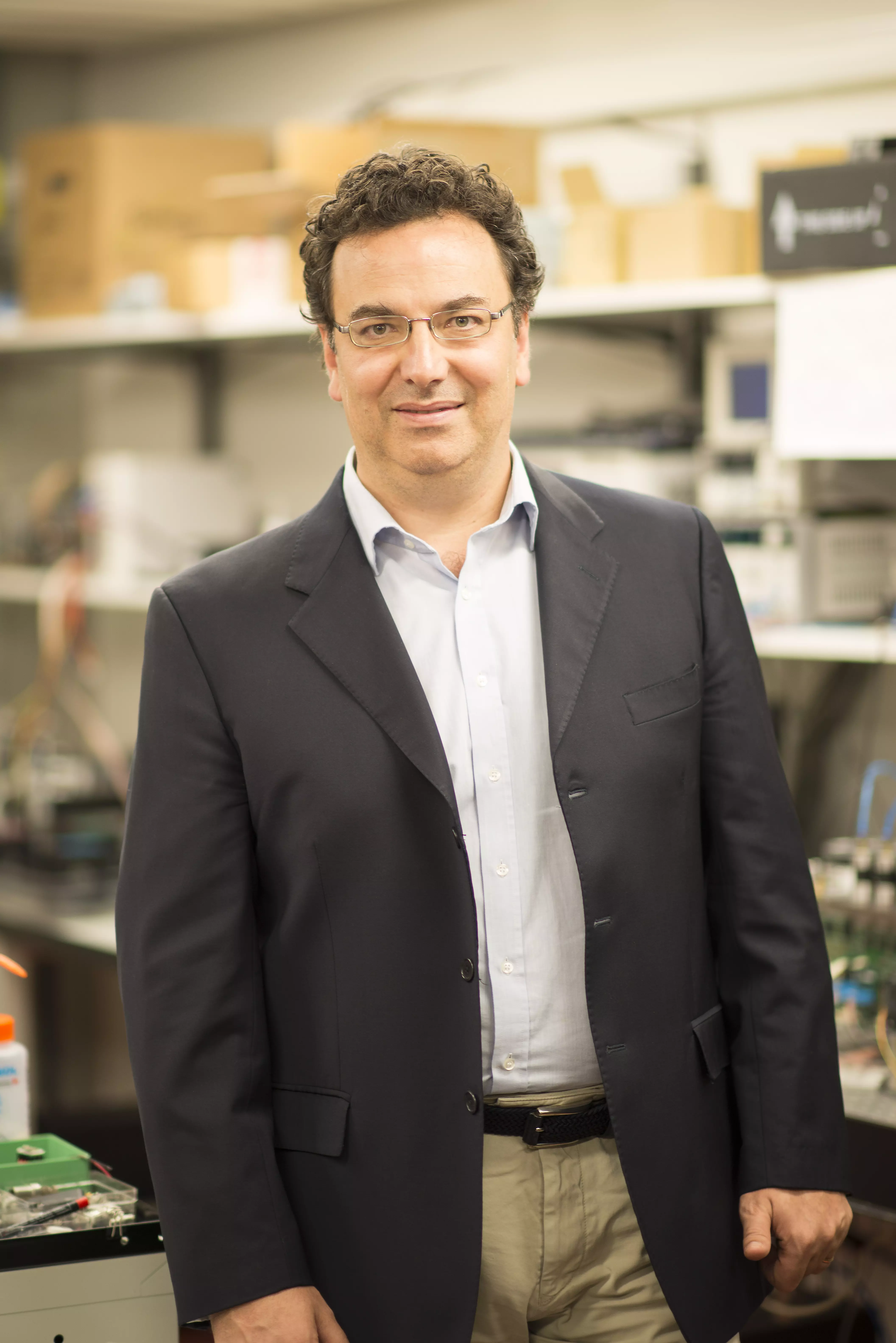
A Brief Biography
Dr. Alessandro Curioni is responsible for IBM corporate research in Europe and IBM's global research agenda in Future of Computing and Security. Dr. Curioni is an internationally recognized leader in the area of high-performance computing and computational science, where his innovative thinking and seminal contributions have helped solve some of the most complex scientific and technological problems in healthcare, aerospace, consumer goods and electronics. He was a member of the winning team recognized with the prestigious Gordon Bell Prize in 2013 and 2015. His research interests include AI and novel compute paradigms, such as quantum and neuromorphic computing, and the convergence of computing technologies to accelerate discovery.
What's Next in AI
What's Next in AI: Today's AI is narrow. While many AI models deliver value in specific, well-defined situations, applying those same models to new challenges requires an immense amount of new data and training. Enterprises need AI that is fluid and adaptable, capable of applying knowledge acquired for one purpose to new domains and challenges. They need AI that can combine different forms of knowledge, unpack causal relationships, and learn new things on its own. In short, enterprises need AI with fluid intelligence. To achieve this vision, we'll have to make significant strides in algorithm development while improving our AI engineering tools and hardware and to make sure it's trusted and secure.
Prof. Dr. Dirk Eddelbuettel
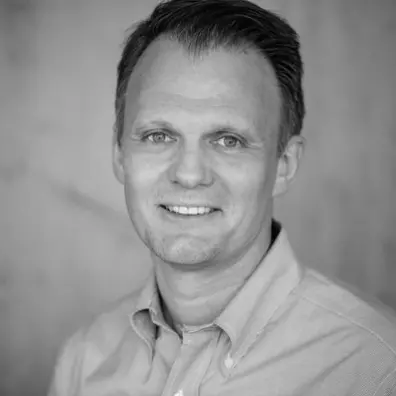
A Brief Biography
Dirk Eddelbuettel is the author / coauthor of several dozen R packages on CRAN including Rcpp; co-creator of the Rocker Project for R use on Docker; an editor at the Journal of Statistical Software; the Debian/Ubuntu maintainer for R, numerous CRAN packages and other quantitative software; an elected board member of the R Foundation; an adjunct Clinical Professor at the University of Illinois at Urbana-Champaign where he teaches a class on Data Science Programming Methods, and a principal software engineer at TileDB. He holds a MA and PhD in Mathematical Economics from EHESS in France, and a MSc in Industrial Engineering from KIT in Germany.
Using R for Machine Learning in a Multi-Lingual World
John Chambers, the key driver behind the S language underlying the R language, environment, and project, stated in his most recent book (Extending R, CRC, 2016) that _Interfaces to other software are part of R_. We illustrate this with use cases from widely deploayed interface packages. As a concrete example, we discuss 'mlpack' by Curtin et al which brings a performant and very complete Machine Learning library to R (using C++ bindings). But connecting to 'code' in other languages is only a first step, connecting to data flow is a portable cross-language manner is another. We highlight some recent work in language agonostic data representations.
Prof. Dr. Josef Teichmann
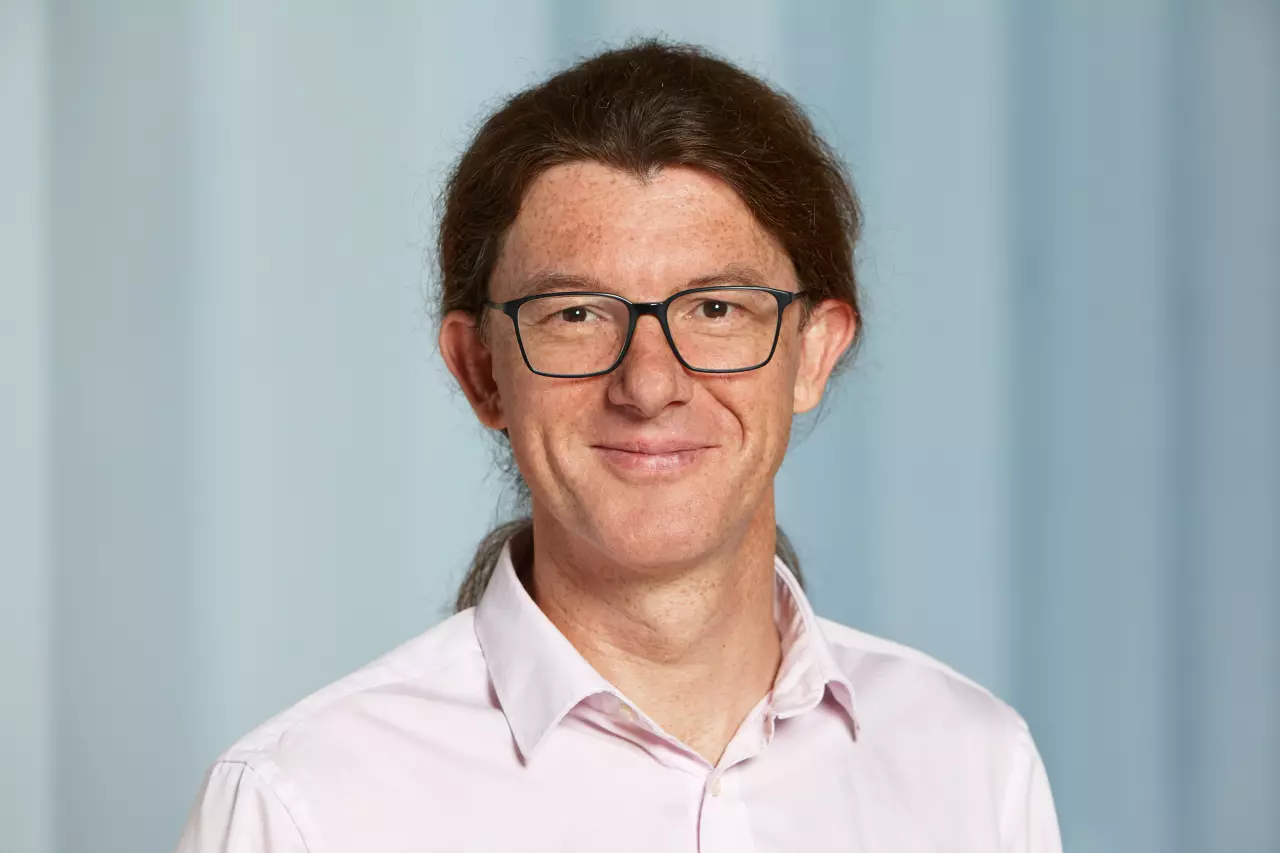
A Brief Biography
Josef Teichmann is a professor at ETH Zürich with particular interest in Mathematical Finance, Stochastic Analysis and Machine Learning in Finance. Recent work focuses on several fundamental problems in Finance such as Pricing, Hedging, Prediction or Calibration from a Machine Learning perspective, and in turn applies methods from Stochastic Finance to deepen the understanding of those new technologies.
Model free Deep Hedging
We combine techniques from Bayesian model selection (Duembgen, Rogers 2014) and Deep Hedging (Buehler, Gonon, Teichmann, Wood 2019) to obtain a purely data driven artifical risk manager. In contrast to Deep Hedging no particular scenario generating process has to be chosen (joint work with Thorsten Schmidt).
Dr. Miguel Noguer i Alonso
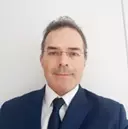
A Brief Biography
Miquel Noguer i Alonso is a financial markets practitioner with more than 20 years of experience in asset management, he is the Founder of Artificial Intelligence Finance Institute. Head of Development at Global AI and co-Editor of the Journal of Machine Learning in Finance.
He worked for UBS AG (Switzerland) as Executive Director. He is member of European Investment Committee for the last 10 years. He worked as a Chief Investment Office and CIO for Andbank from 2000 to 2006. He started his career at KPMG.
He is Visiting Professor at NYU Courant Institute of Mathematical Sciences and the CQF institute. He has been Adjunct Professor at Columbia University teaching Asset Allocation, Big Data in Finance and Fintech. He is also Professor at ESADE teaching Hedge Fund, Big Data in Finance and Fintech. He taught the first Fintech and Big Data course at the London Business School in 2017.
He received an MBA and a Degree in business administration and economics in ESADE in 1993. In 2010 he earned a PhD in quantitative finance with a Summa Cum Laude distinction (UNED – Madrid Spain). He completed a Postdoc in Columbia Business School in 2012. He collaborated with the Mathematics department of Fribourg during his PhD. He also holds the Certified European Financial Analyst (CEFA) 2000. He also holds the ARPM certificate.
His research interests range from asset allocation, big data, machine learning to algorithmic trading and Fintech. His academic collaborations include a visiting scholarship in Columbia University in 2013 in the Finance and Economics Department, in Fribourg University in 2010 in the mathematics department, and giving presentations in Indiana University, ESADE and CAIA and several industry seminars like the Quant Summit USA 2019 and 2010.
A Meta-Learning approach to Model Uncertainty in Financial Time Series
Financial markets have experienced several negative sigma events in recent years; these events occur with much more regularity than current risk models can predict. There is no guarantee that the training set's data generating process will be the same in the test set in finance. Mathematical models are designed to operate with unlimited and changing data, and yet, actual events keep making life hard for most models. The assumption of independent and identically distributedrandom variables and a stationary time series do not hold in reality. Over-reliance on historical data and backtesting of models is not a sufficient approach to overcome these challenges.Reinforcement-learning faces similar challenges when applied to financial time series.Out-of-distribution generalization is a problem that cannot be solved without assumptions onthe data generating process. If the test data is arbitrary or unrelated to the training data, then generalization is not possible. Finding these particular principles could potentially help us buildAI and financial modeling systems. N-Beats, Oreshkin et al. [2020], is a deep neural architecture with backward and forward residual links and a deep stack of fully-connected layers. N-Beats can be considered as a meta-learning model for time series prediction. Meta-Learning is a machine learning approach that intends to design models that can learn new skills or adapt to new environments rapidly with few training examples. We explore the performance of N-Beats and compare its performance with other deep learning models. The results are not conclusive in establishing N-Beats as a better model than the other models tested in this study. We show in this study that other neural network-based models offer similar performance.
Dr. Jan-Alexander Posth & Dr. Jochen Spuck
A Brief Biography
Dr. Jan-Alexander Posth is a senior lecturer at the Institute for Wealth and Asset Management at the ZHAW School of Management and Law, with a research focus GreenTech and AI in finance. He has more than 12 years’ of professional track record in the financial industry, where he gained extensive expertise as a risk manager, quant and portfolio manager. Starting at Deutsche Postbank as a credit risk manager, Alex moved on to Landesbank Baden-Württemberg where he led the fund derivatives trading desk. Joining STOXX Ltd. in 2012, he was responsible for the development of smart-beta equity indices before becoming Head of Research and Portfolio Management at a start-up hedge fund in 2015. Alex changed to ZHAW in 2017; he holds a PhD in theoretical physics.
Dr. Jochen Spuck, Chief Technology Officer EconSight studied organic and inorganic chemistry at the University of Fribourg in Switzerland and graduated as Dr. rer. nat in inorganic chemistry (combined studies on inorganic polymer chemistry ("Smart Polymers") and computational chemistry (factor analysis (multivariate statistics) and other methods). Between 1999 and 2008, he worked as project manager and later head of R&D in two SME’s in Switzerland developing and producing digital print media, often in cooperation with larger companies as well as Head of International Customer Support at Mettler Toledo’s Sensor Branch. He joined the Institute of Intellectual Property (IGE, the “Swiss Patent Office”) in Bern in 2008 and worked from 2008 and 2018 as a researcher and examiner and most recently as head of product developments of ip-search, the international service branch of the IGE. He was the responsible head of the Artificial Intelligence group for patent searches as well as the patent searchers' digital toolbox. In addition, as Head of Business Development, he was responsible for digital business methods in the field of patents and IP as well as for relations with corporate clients. He achieved QPIP certified patent searcher and analyst status and was educated as patent search trainer by the EPO. He also developed the foundation of the technology field project, which evolved later to be the heart of the company EconSight, Gmbh, which he co-founded in 2019 together with Kai Gramke. He is now responsible for the technological content of the company’s products, analysis and studies. As such he contributed largely to technology analysis for and in cooperation with EPO, ESA, Bertelsmann Foundation, Handelsblatt, Forbes Digital or VbW Zukunftsrat Bavaria as well as many other (industry & finance) corporate patent and technology projects meanwhile.
Thematic Investing in GreenTech: Patent-data-driven Identification of Green Technology Trends and Sustainable Investment Opportunities
GreenTech is the single-most important enabler to unlock the technological transformation and innovation potential necessary for the change towards a more sustainable economy and society. By now, it has been recognized that ESG compliance and more sustainability will not be realized by economization only; rather, ESG-compliant investing and policy design is needed to creatively reshape entire industries and proactively advance this transformation.
We will discuss how AI-supported analysis of patent data helps to identify up-and-coming trends in green technologies, how maps of GreenTech can provide guidance for policy makers and regulators, and how essential technology trends can be mapped to thematic investment themes.
Prof. Dr. Natalie Packham
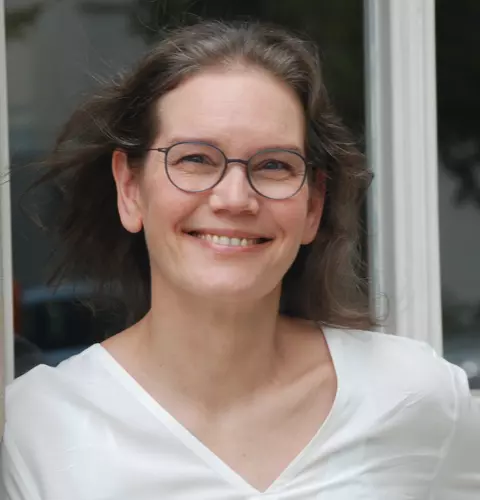
A Brief Biography
Natalie Packham is Professor of Mathematics and Statistics at Berlin School of Economics and Law and Principal Researcher within the International Research Training Group “High Dimensional Nonstationary Time Series” (IRTG 1792) at Humboldt University Berlin. Natalie has several years of industry experience as a front office software engineer at an investment bank. Her research expertise includes Mathematical Finance, Financial Risk Management and Computational Finance, and her academic work has been published in Mathematical Finance, Finance & Stochastics, Quantitative Finance, Journal of Applied Probability and many other academic journals. Natalie holds an M.Sc. in Computer Science from the University of Bonn, a Master’s degree in Banking & Finance from Frankfurt School, and a Ph.D. in Quantitative Finance from Frankfurt School.
Correlation scenarios and correlation stress testing
We develop a general approach for stress testing correlations in stock and credit portfolios. Using Bayesian variable selection methods, we build a sparse factor structure, linking individual names or stocks with country and industry factors. We specify a parametric form of the correlation matrix, where correlations of stock returns are represented as a function of the country and industry factors. Regular calibration yields a distribution of economically meaningful stress scenarios on the factors, which can then be translated into stressed correlations. The method also lends itself as a reverse tress testing framework: using e.g. the Mahalanobis distance on the joint risk factor distribution, allows to infer worst-case correlation scenarios. We give examples of stress tests on a large portfolio of European and North American stocks.
Prof. Dr. Peter Schwendner
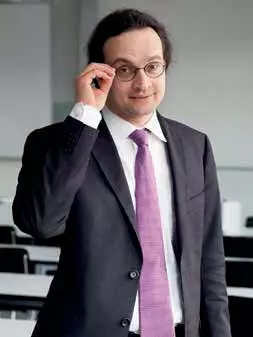
A Brief Biography
Peter Schwendner leads the Institute of Wealth & Asset Management at Zurich University of Applied Sciences, School of Management and Law. He collected 15 years of work experience in the financial industry as a head of quantitative research at Sal. Oppenheim and as a partner at Fortinbras Asset Management after he completed a doctorate in physics. He has been developing analytics for primary and secondary markets and quantitative risk premia strategies with financial industry partners.
Interpretable Machine Learning for Diversified Portfolio Construction
The use case benchmarks hierarchical risk parity (HRP) relative to equal risk contribution (ERC) as examples of diversification strategies allocating to liquid multi-asset futures markets with dynamic leverage (volatility target). The authors use interpretable machine learning concepts (explainable AI) to compare the robustness of the strategies and to back out implicit rules for decision-making.
Dr. Pascal Paysan
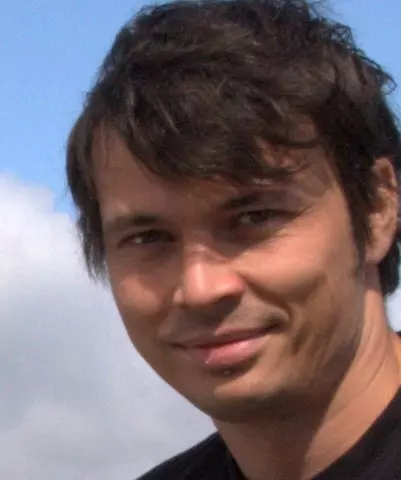
A Brief Biography
Pascal is working as Sr. Research Scientist in the medical imaging domain for Varian, a Siemens Healthineers Company, since more than 10 Years. Beginning his carrier at Varian he worked in product development and lead the development of Iterative Cone Beam CT (iCBCT) reconstruction algorithms. Since 2019, he became part of Applied Research exploring future technologies for improved image guidance in radiation therapy. In this context he is guiding several projects leveraging deep learning techniques to improve image quality and model patient motion. In his current role he is in charge of research collaborations with leading scientists in the medical imaging field such as DKFZ and UCLA.
Pascal graduated at University of Basel and attained his PhD in computer science 2010 for his work on statistical modeling of facial aging. During his PhD research he applied machine learning, generative 3D shape models, computer graphics, and computer vision methods. At University of Applied Sciences Esslingen he obtained his Dipl. Ing. degree 2004. During his studies he worked for Daimler AG in the computer vision for autonomous driving field. He carried out his diploma thesis, about detection of oncoming cars using stereo vision and machine learning, at MIT in Boston.
Applications for AI in Image Formation for Radiation Therapy at Varian
Varian, a Siemens Healthineers company is the market leader in radiation oncology hard- and software solutions and has a long track record in transforming technical innovation into successful customer facing products, touching cancer patients throughout the world. Artificial Intelligence (AI), Machine Learning (ML) and Deep Learning (DL) technologies made their way into every day’s applications and are currently of huge interest in various domains.
In recent research it became evident that AI can be successfully applied in various medical imaging domains. In our studies we are focusing on X-ray based imaging and computed tomography. Ongoing studies applying data driven methods in this domain are showing promising results and are subject to explore the practical clinical value prior a product development decision will be made.
In this presentation promising early results, implementation strategies and product opportunities will be discussed to demonstrate the potential added value of AI technology in clinical Image-Guided Radiation Therapy (IGRT).
Dr. Iason Kastanis
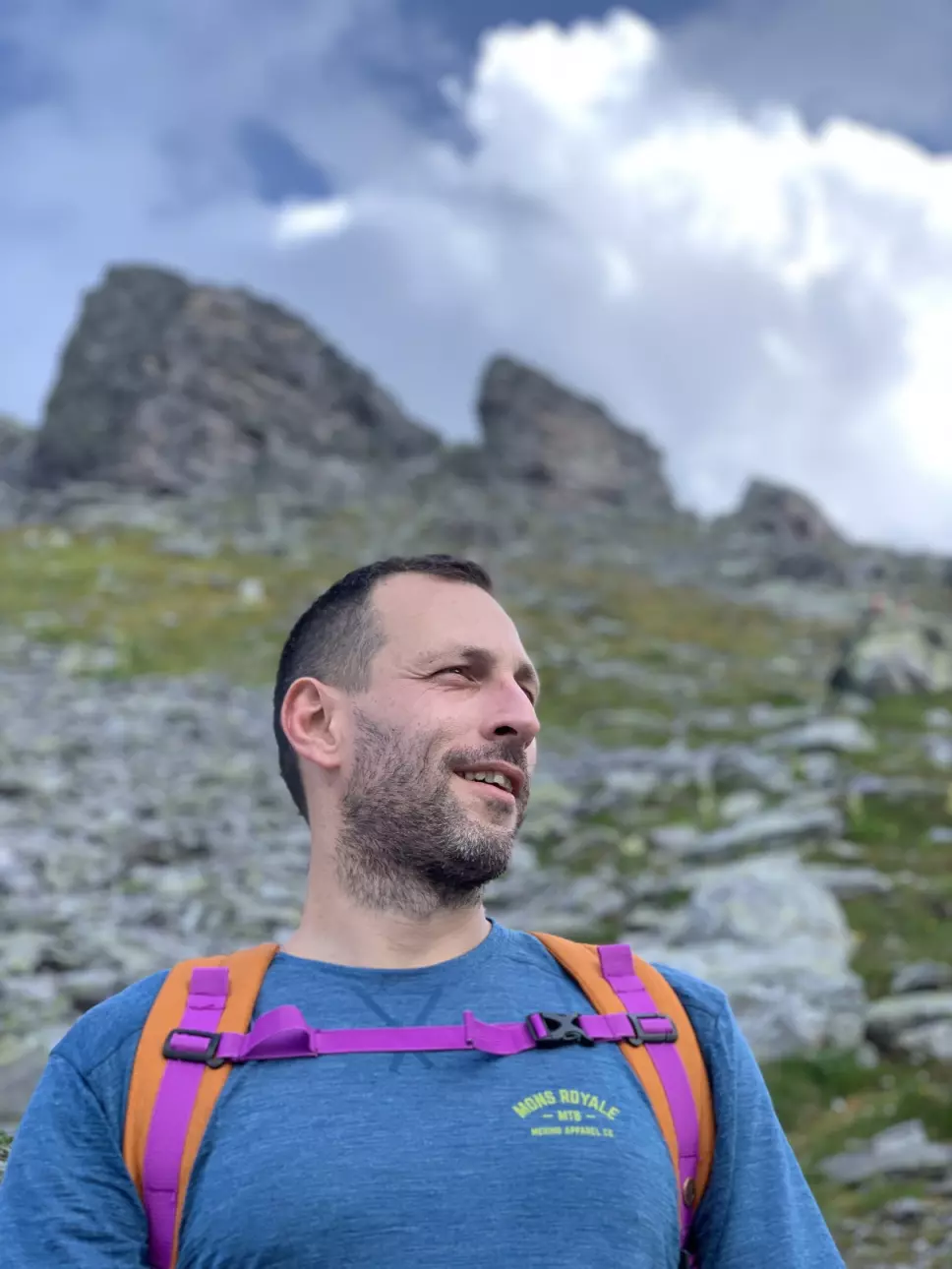
A Brief Biography
Iason Kastanis obtained his MSc (2002) and PhD (2007) in computer science from University College London. He focused on signal processing, computer vision and optimization methods. He continued his career as a developer of image reconstruction software in the UK integrating his PhD research in a medical product. He worked as Post-Doc in the University of Barcelona in the area of Virtual Reality. His passion for applying new technology in the real-world has led him to CSEM in 2013. He is currently an Expert in Machine Learning and Vision leading multiple projects in the fields of predictive maintenance and quality inspection.
Deep Learning for quality inspection of injection molding parts
Camera based systems for quality inspection are nowadays available as Commercial Off The Shelves (COTS) hardware and software bundles. While COTS have come a long way in the last 20 years, there are still a variety of problems were customized solutions are required. On the contrary open source tools are offering a wide selection of methods, but that is typically too complex to be used by non-expert users. In this talk we will present the complete design and development of an optical quality control system for KNF (https://www.knf-flodos.ch) pump parts. An often neglected and underestimated stage is that of data preparation. Data preparation includes not the only the acquisition of images, but also the annotation and processing in order to make the data set analysis ready for the algorithms. We will present how we can simplify this process with effective tools and the use of AI. Often in academic studies data sets are fixed, and the evolution of real-world systems is not accounted for. In this sense many works present excellent result on static benchmark problems. It is very common in industrial applications that the problem is dynamic and changes over time. The presented system is capable of evolving through user input without requiring the intervention of a data scientist. The models are adapting to changing conditions according to the direction given by the operator in the form of labelling. It is exactly this limitation of many available solutions that restricts their readiness for real world application, our system is designed to be flexible at the hands of the customer.
Dr. Stefan Pauli
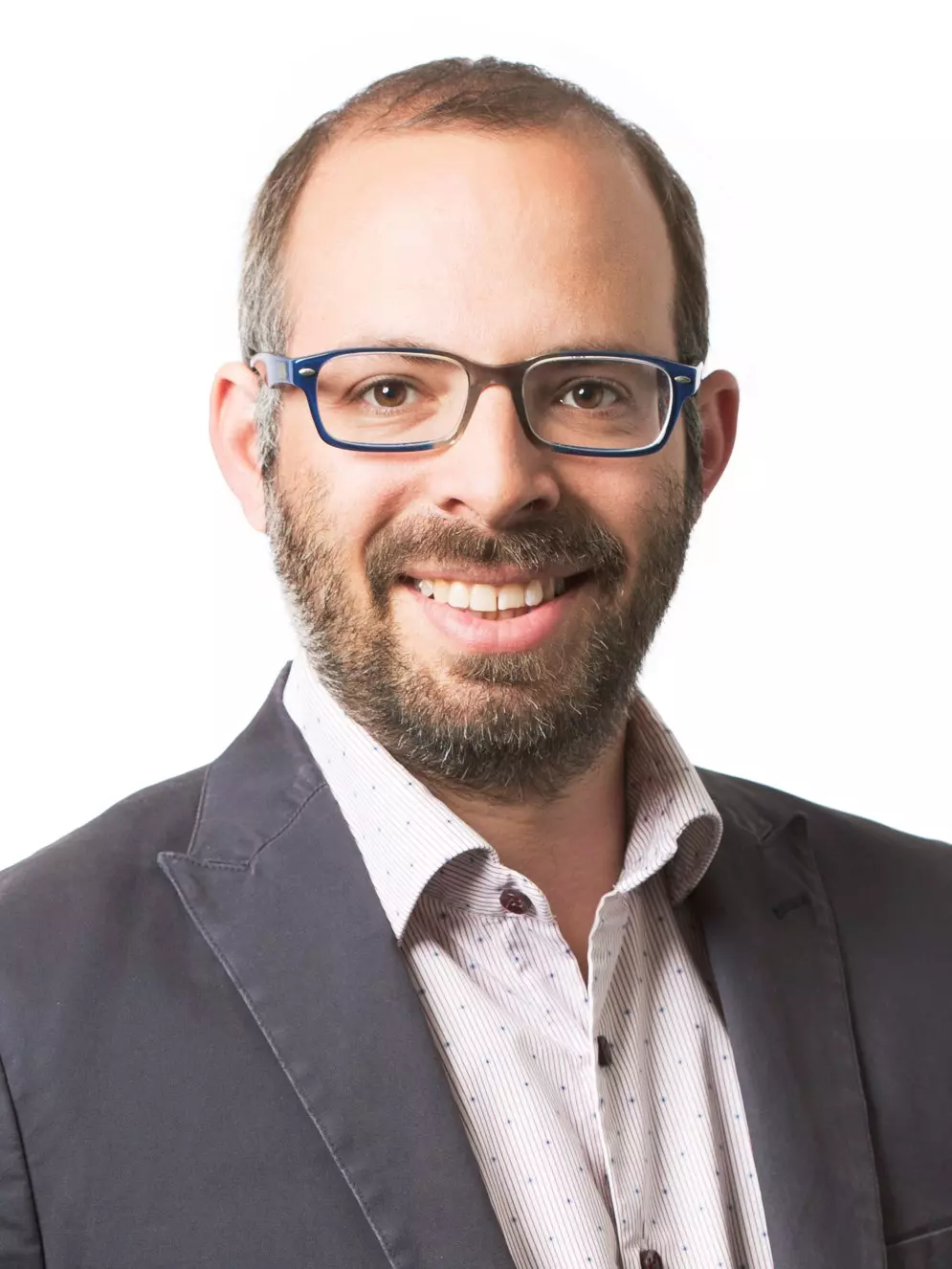
A Brief Biography
Stefan Pauli did his PhD related to computer science and mathematics at ETH Zürich. He gained additional experience in algorithm development in several industrial companies and startup’s. His background, including an industrial apprenticeship, and his experience helps him to link the world of algorithm with the industrial practice. Today he is a Senior Data Scientist at VTU Engineering, where he implements data analysis projects mainly in the chemical and pharmaceutical production, according to the motto: start small and grow with the success.
Machine learning in the GMP regulated industries
In industry, the increased amount and availability of process, machine and quality control data has opened up profitable opportunities by analyzing this data with advanced methods such as machine learning. In this context, machine learning is already successfully used for production optimization to guarantee e.g. high and consistent quality as well as increased throughput. However, in GMP (Good Manufacturing Practices) environments such as pharmaceutical production, the high regulatory requirements must be met at all times. This places high demands on the quality and documentation of machine learning-driven data analyses.
Michael Berns
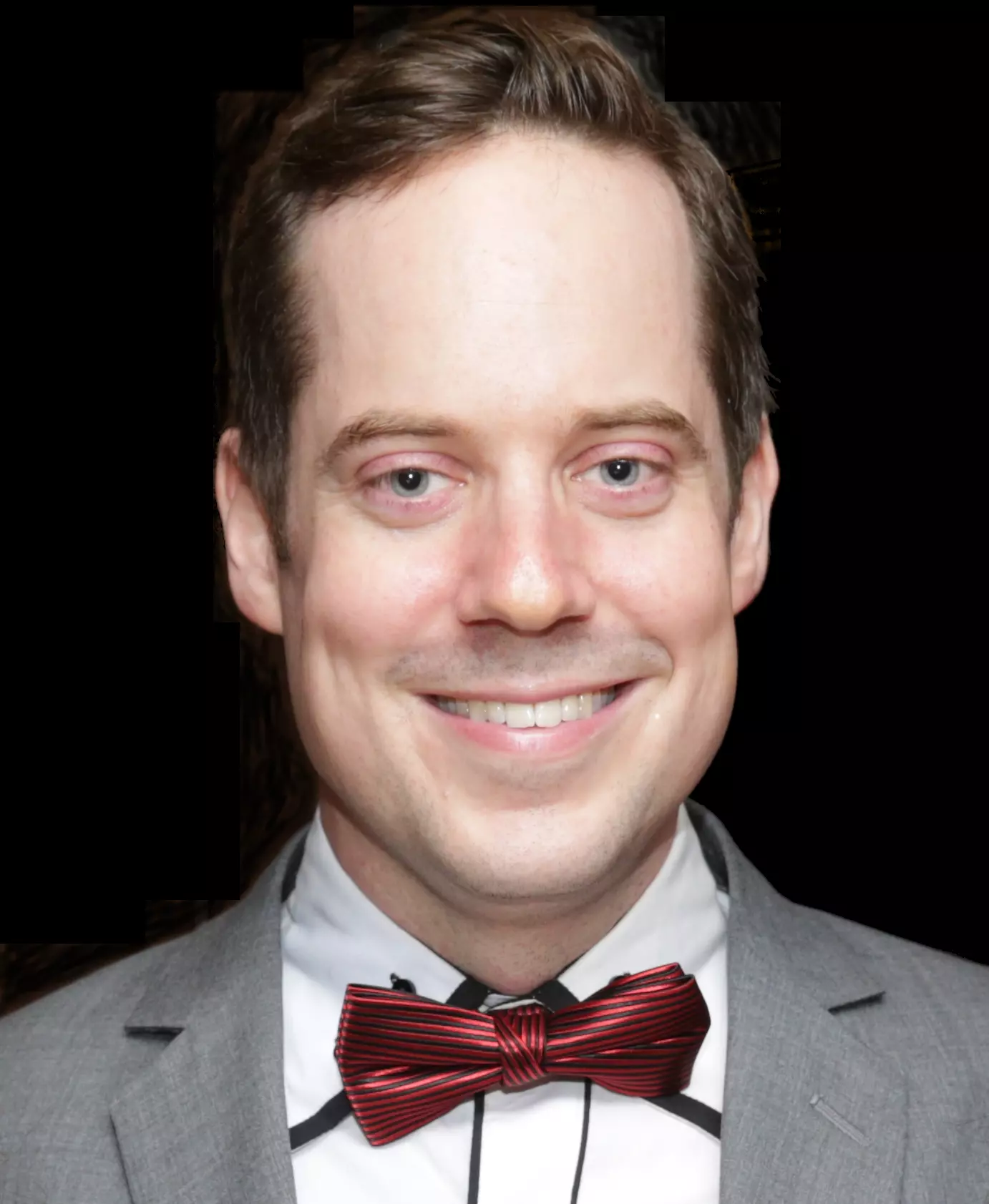
A Brief Biography
Michael's talk is about how to use AI for sustainability, not only to inspire and educate but as a call to action! Michael is Director for AI & FinTech at PwC. Michael has a broad background across blue chip names such as Morgan Stanley & Moody's as well as a range of smaller innovative AI Firms.
Aside from his day job he also takes a keen interest in understanding the latest in innovation by helping AI firms to scale and has been a Mentor and Judge for organizations like Startup Bootcamp, Virgin Money Startup, Cocoon Network, Level 39, MIT IIC and the United Nations World Food Program for the last 10 years.
As a well-known Expert in his field, Michael acts as keynote speaker at international conferences and guest lecturer at London Business School and Mannheim Business School.
The “AI Book” to which he contributed as Co-Author was released last year, together with the PwC "AI in Financial Services" study (based on 150 experts).
Many of Michael's other articles, past speaking engagements and contributions to AI are available via his LinkedIn profile. This talk was given at a TEDx event using the TED conference format but independently organized by a local community. Learn more at https://www.ted.com/tedx
AI und Sustainability / ESG
AI Superpowers for Sustainability - how do we leverage AI-driven insights to speed up our climate change strategy? In this short overview Michael will summarize his TEDx talk and latest findings from international AI projects to improve sustainability.
Dr. Andreas Fitze
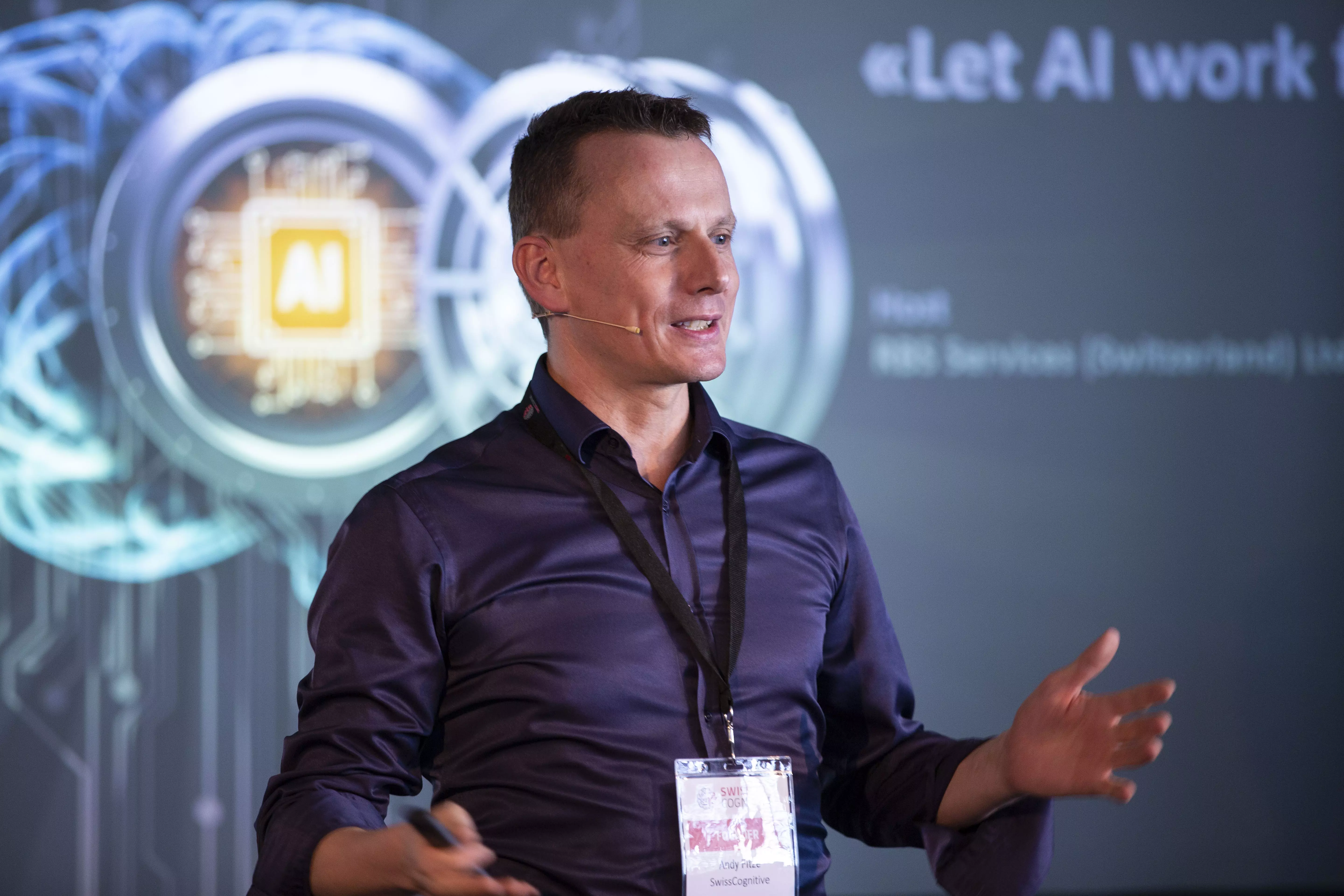
A Brief Biography
Andy Fitze is a digital cognitive strategist, top global AI and digital transformation advisor for start-ups and enterprise boards, tactical leader, and an AI influencer. With Dalith Steiger, Andy is the co-founder of the award-winning start-up SwissCognitive, and the CognitiveValley Foundation. He is president of the Swiss IT Leadership Forum, a member of the Board of Directors of SwissICT, and a Chairman & Member of various boards of Directors. Andy is a lecturer and member of the Strategic Advisory Board at Bern University of Applied Sciences and a lecturer at the ETH Zürich. To share his 30 years of extensive knowledge and experience, he is often seen on global stages. He is also a passionate skipper on the oceans – providing him with an excellent balance for head and soul.
Data explosion, but all hidden in your data centre
In my keynote, I focus on the interrelation between data quality, quantity, accessibility, infrastructure, and literacy and how these components can be advanced in harmony with human cognition to translate into efficiency and productivity for society and business.
Data on its own is nothing. So is technology. There are many ingredients in play. Still, even top-notch data and technology would not line up without human intervention. No component plays a more critical role than the other. Human cognition can advance the interrelation between data and AI in harmony for the benefit of all aspects of our lives.
Dr. Teresa Scantamburlo
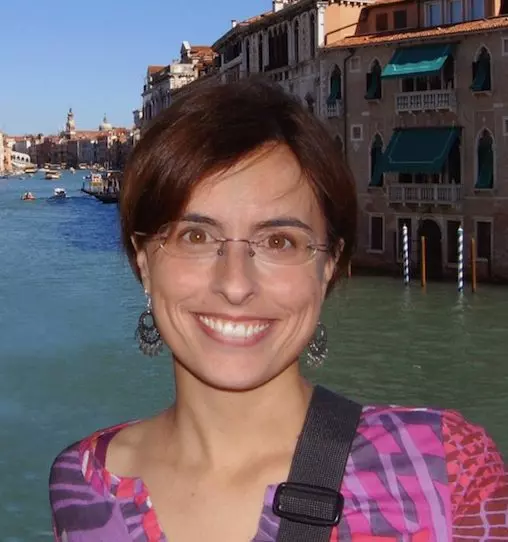
A Brief Biography
I’m a post-doc at the European Centre for Living Technology (ECLT), Ca’ Foscari University (Italy), working on the AI4EU project. Before now, I worked on the ThinkBIG project at the University of Bristol (UK).
My research interests lie at the intersection of Philosophy and Artificial Intelligence. Currently I’m interested in the social and ethical impacts of AI, in particular, on human decision-making and social regulation.
I received my PhD in Computer Science from Ca’ Foscari University (Venice, Italy) under the supervision of professor Marcello Pelillo. My PhD thesis explored the philosophical foundation of machine learning and pattern recognition. I completed a B.Sc. in Computer Science and a M.A. in Digital Humanities at the same University.
Surveying the Opinion of European Citizens on AI
In this talk I will outline a collaborative research conducted in the context of AI4EU, a Horizon 2020 project connecting AI stakeholders and AI resources in a dedicated platform. The goal of this effort was to understand the view of European citizens on AI. In particular, our research aimed to explore: 1) to what extent EU citizens are aware of AI and its impact; 2) citizens' attitude towards AI; 3) citizens' trust in AI. To investigate these topics we launched a survey involving a sample of 4000 citizens spread across 8 EU countries in May 2021. In this presentation I will briefly introduce the methodology we used and some meaningful results that we collected.
Joachim Baumann & Dr. Christoph Heitz
A Brief Biography
Joachim Baumann is a PhD candidate at the Zurich University of Applied Sciences and the University of Zurich. Prior to starting the joint PhD program in 2021, he graduated from the University of Zurich with a master’s degree in information systems and data science. He worked as an application developer and later on in project management consulting. In his research, he focuses on the intersection of algorithms and ethics. In particular, he is interested in questions of fairness when applying machine learning for automated decision making. He investigates these topics on a theoretical and a practical level in various fields such as insurance, recruitment, and criminal justice.
Christoph Heitz is professor for Operations Management at the Institute of Data Analysis and Process Design (IDP) at Zurich University of Applied Sciences. His research areas include data-based decision making in business processes, data-based service innovation, and ethics in Machine Learning.
After a PhD in Theoretical Physics (University of Freiburg, German), he worked in software industry and in industrial research before joining ZHAW in 2000.
In 2017, he co-founded the Swiss Alliance for Data-Intensive Services and serves as its president since then. Data+service is a Swiss national innovation network consisting of more than 40 companies and more than 20 research institutions, involving more than 300 researchers and professionals. Its goal is to foster innovation in the field of data-based services in Switzerland, by establishing cooperation within its interdisciplinary expert network of innovative companies and universities, combining knowledge from different fields into marketable products and services.
Fairness of insurance premiums: May personalized risk models lead to discrimination and social injustice
More and more, personalized risk models are used for determining insurance premiums. In this context, issues of fairness, discrimination, and social injustice might arise: Algorithms for estimating the risk based on personal data may be biased towards specific social groups, leading to systematic disadvantages for those groups. Personalized premiums may thus lead to discrimination and social injustice. It is well known from many application fields that such biases occur frequently and naturally when prediction models are applied to people unless special efforts are made to avoid them. Insurances are no exception.
In our paper, we provide a thorough analysis of algorithmic fairness for the case of insurance premiums. We ask what "fairness" might mean in this context and how the fairness of a premium system can be measured. For this, we apply the established fairness frameworks of the Fair Machine Learning literature to the case of insurance premiums and show which of the existing fairness metrics can be applied to assess the fairness of insurance premiums. We show that one of the often-discussed fairness criteria (separation) does not make sense for insurance premiums. However, we found that another fairness criterion (sufficiency) allows us to test for systematic biases in premiums towards some group with respect to the risk that they bring to the pool. Our results enable insurances to assess the fairness properties of their risk models, helping them avoid reputation damage because of possibly unfair and discriminatory premium systems.
Dr. Michael Karner
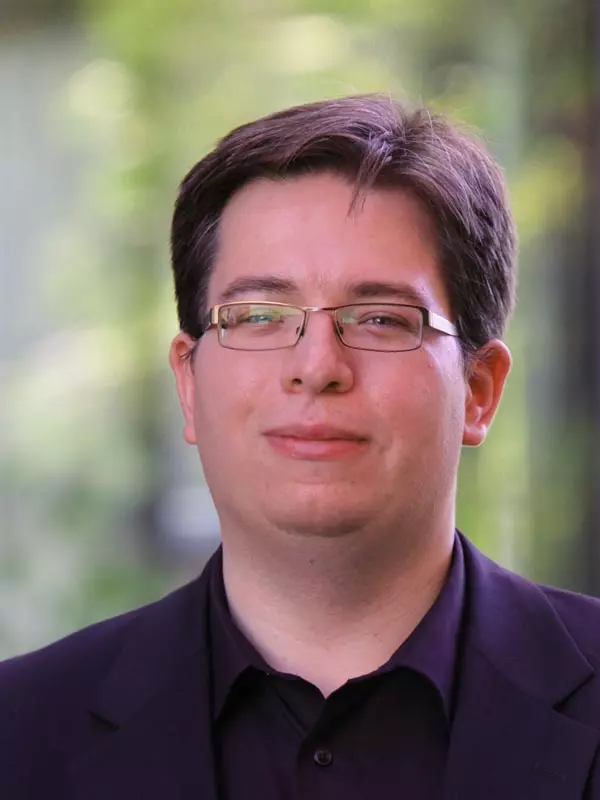
A Brief Biography
Dr. Michael Karner is lead researcher at VIRTUAL VEHICLE in Graz, Austria. He received a master’s (Information and Computer Engineering) and doctoral degree (Electrical Engineering) from Graz University of Technology. He was the coordinator for the recently finished ECSEL project SCOTT (focussing on cost-efficient solutions of wireless, end-to-end secure, trustworthy connectivity and interoperability in the Internet of Things), with a budget of 40M€ and involving nearly 60 partners from 11 European countries and Brazil). Currently, he is coordinator of the ECSEL InSecTT (Intelligent Secure Trustable Things) project dealing with bringing the Internet of Things and Artificial Intelligence together and making it trustworthy, with a budget of over 40M€ and involving more than 50 partners from 12 countries. He has more than ten years industrial and scientific experience in the field of intelligent and connected systems (including artificial intelligence, automated driving, trustworthiness…). Furthermore, he is active as a reviewer and technical program committee member in several conferences and journals.
Bringing Internet of Things and Artificial Intelligence together – but is it trustworthy?
Artificial Intelligence of Things (AIoT) is the natural evolution for both Artificial Intelligence (AI) and Internet of Things (IoT) because they are mutually beneficial. AI increases the value of the IoT through Machine Learning by transforming the data into useful information, while the IoT increases the value of AI through connectivity and data exchange. However, users are challenged to understand and trust their increasingly complex and smart devices, sometimes resulting in mistrust, usage hesitation and even rejection.
InSecTT – Intelligent Secure Trustable Things, a pan-European effort with 52 key partners from 12 countries (EU and Turkey), provides intelligent, secure and trustworthy systems for industrial applications. This results in comprehensive cost-efficient solutions of intelligent, end-to-end secure, trustworthy connectivity and interoperability to bring the Internet of Things and Artificial Intelligence together. InSecTT aims at creating end-user trust in AI-based intelligent systems and solutions as a major part of the AIoT. Trustworthy AI has three components: it should be lawful, it should be ethical, and it should be robust. In InSecTT, we focus on robustness and ethics, ensuring our developed systems are resilient, secure and reliable, while prioritising the principles of explainability and privacy.
Dr. Gabriele Bolek-Fügl
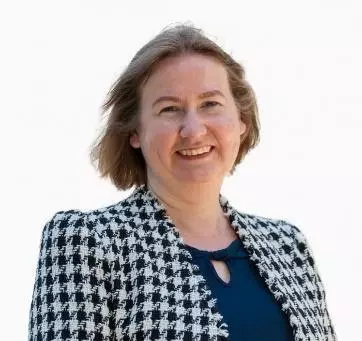
A Brief Biography
Gabriele Bolek-Fügl worked for more than 22 years in the area of IT compliance, primarily at international auditing networks with the areas of data protection, compliance frameworks and cyber security. The current focus of her activities is artificial intelligence and how companies can successfully use it for operations. In doing so, she connects the requirements of business with legal requirements and digital tools/algorithms.
In 2020, she founded the startup Compliance 2b and, together with her co-founders, provides a legally compliant channel for anonymous internal reporting in organizations. This system supports whistleblowers in submitting high quality reports as well as compliance officers in resolving them with AI functions.
Definition of Wrong
We believe we have a solid understanding of many things in our everyday working lives, such as wrongdoing in our own organization. But what does wrongdoing actually mean? While it is quite easy to define theft, it is often more difficult to do so in other areas. When and with which actions does, for example, bullying begin?
We use AI algorithms to reduce wrongdoing in organizations. In my presentation, I will talk about the challenges that need to be considered.
Dr. Branka Hadji Misheva
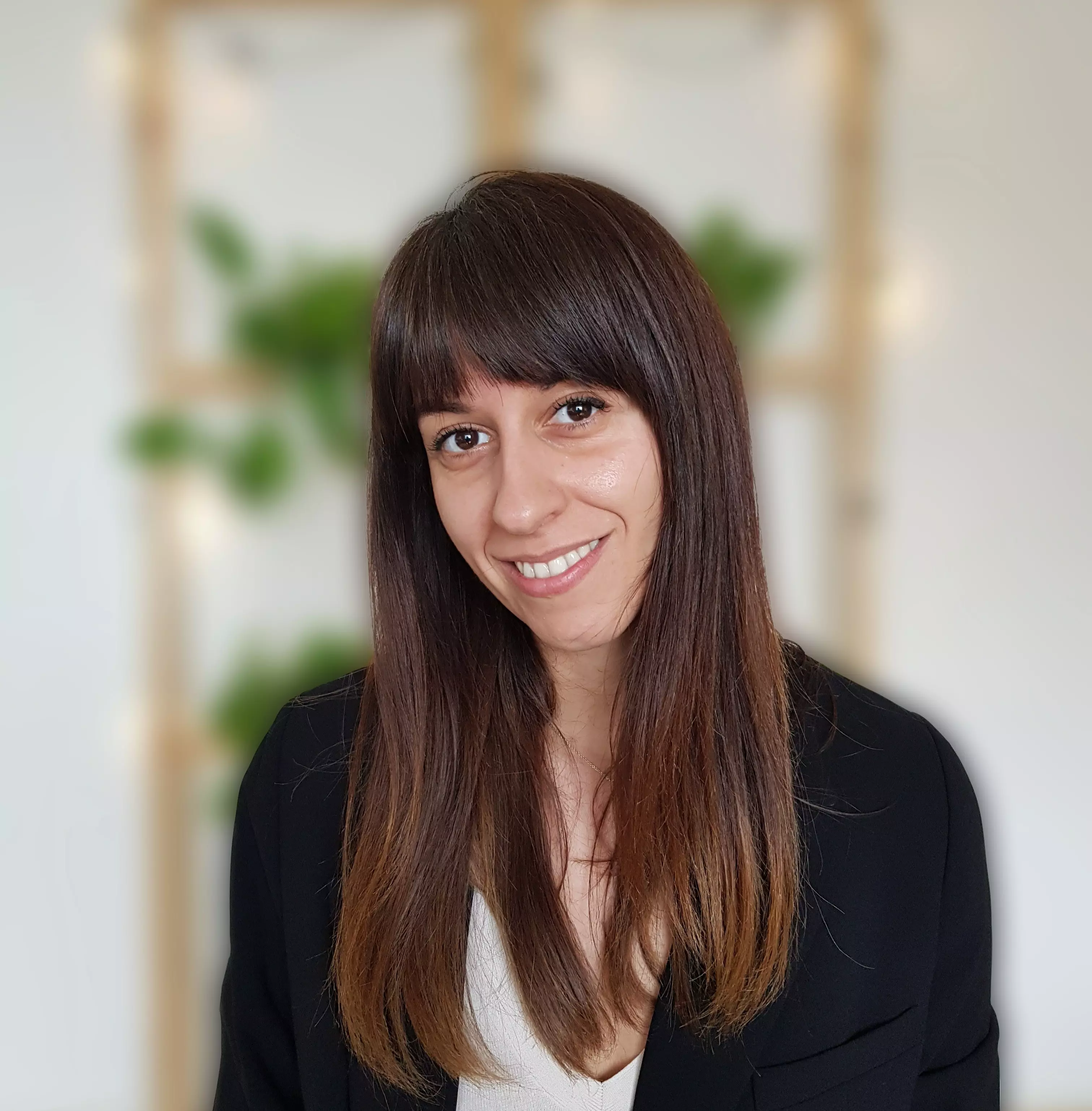
A Brief Biography
Branka Hadji Misheva is researcher at ZHAW Zurich University of Applied Sciences, working on AI applications in finance, XAI methods, network models and fintech risk management. She holds a PhD in Economics and Management of Technology with a specific focus on network models as they apply to the operation and performance of P2P lending platforms, from the University of Pavia, Italy. At her position at ZHAW, she leads several research and innovation projects on Artificial Intelligence and Machine Learning for Credit Risk Management. She is a research author of 10 papers in the field of credit risk modeling, graph theory, predictive performance of scoring models, lead behavior in crypto markets and explainable AI models for credit risk management.
Explainable AI in Finance
Artificial Intelligence (AI) has created the single biggest technology revolution the world has ever seen. For the finance sector, it provides great opportunities to enhance customer experience, democratize financial services, ensure consumer protection and significantly improve risk management. While it is easier than ever to run state-of-the-art machine learning models, designing and implementing systems that support real-world finance applications have been challenging. In large part this is due to the lack of transparency and explainability which in turn represent important factors in establishing reliable technology. The research on this topic with a specific focus on applications in credit risk management has been limited. In this paper, we implement different advanced post-hoc model agnostic explainability techniques to machine learning (ML)-based credit scoring models applied to loan performance data. We present multiple comparison scenarios and we discuss in detail the practical challenges associated with the implementation of these state-of-art eXplainabale AI (XAI) methods.
Prof. Dr. Dirk Wilhelm
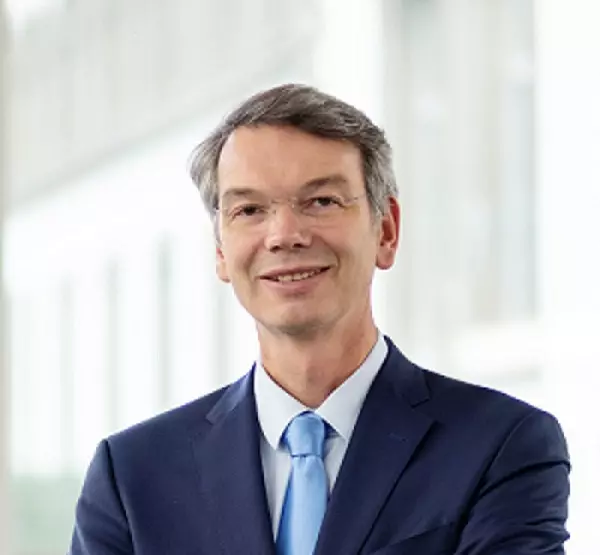
A Brief Biography
Dirk Wilhelm is Professor and Dean of the ZHAW School of Engineering, Zurich, Switzerland. He received his Master’s degree in Physics from University of Göttingen in Germany and his PhD in Mechanical Engineering from ETH Zurich in Switzerland. He worked as a development engineer for Alstom Power Systems in the gas turbine development department. Afterwards he joined the biomedical company Bruker BioSpin, where he headed a development department. In 2013 Dirk Wilhelm joint the School of Engineering at Zurich University of Applied Sciences (ZHAW) and became professor for Medical Physics. Since 2019 he is Dean of the School of Engineering and member of the University Board. He has 15 years of work experience in applied physics and mathematics. His research interests are in Medical Physics, Nuclear Magnetic Resonance Spectroscopy, Computational Fluid Dynamics, and Numerical Methods.
Prof. Dr. Galena Pisoni
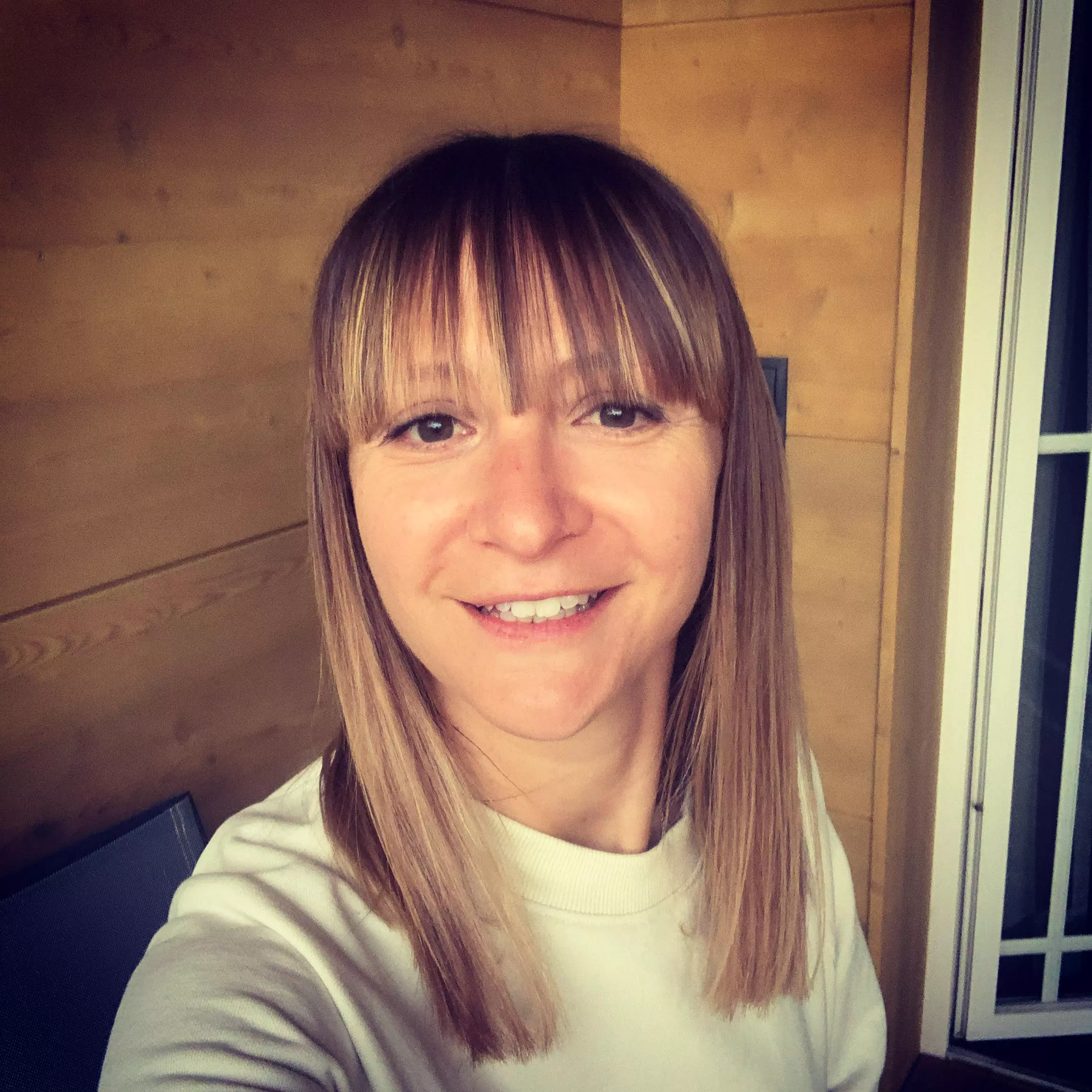
A Brief Biography
Galena Pisoni is a Lecturer and Innovation & Entrepreneurship (I&E) coordinator at University of Côte d’Azur, in this role she teaches I&E courses for computer science students and plan and coordinate local research projects and developments towards I&E
Innovative big data and AI-empowered solutions for financial companies
We live in an era of big data. Large volumes of complex and difficult to analyze data exist in a variety of industries, including the financial sector. In this talk, I'll discuss the role of innovative big data and AI-empowered solutions for financial services: first, I'll present an overview of data science tools and methods that financial companies use, second, I'll present de facto standard enterprise architectures of financial companies and show how data lakes, and data warehouses play a central role in a data-driven financial company, and last, I'll discuss a conceptual framework for AI-enabled financial sustainability, that is approaches for measuring adherence to sustainability goals for companies of different domains through financial data. Emerging technologies offer opportunities for finance companies to plan and develop additional services, and in my talk, I'll discuss implications from AI based practices and research industry agenda.
Dr. Gabriele Schwarz
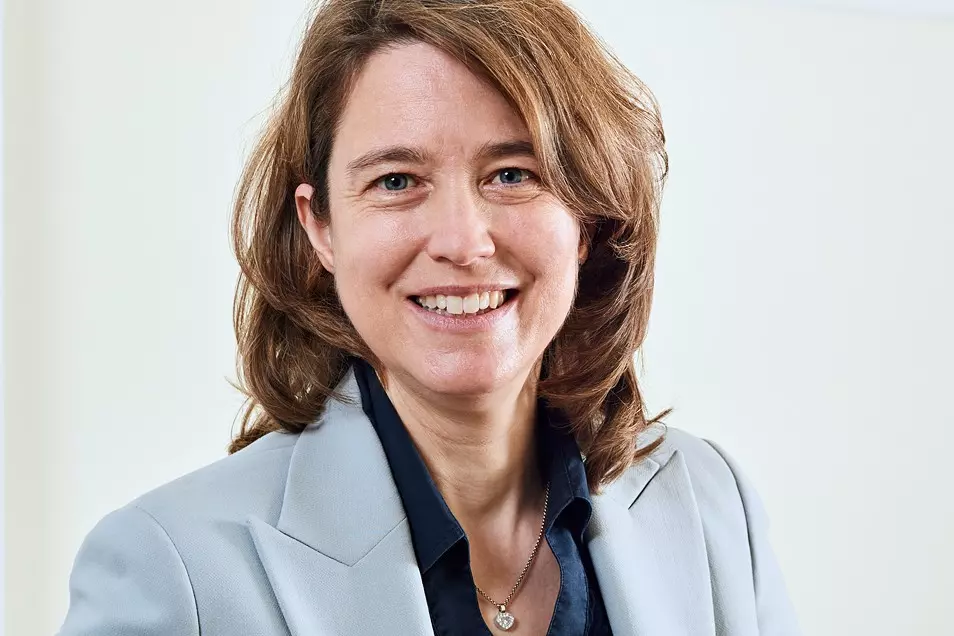
A Brief Biography
Gabriele Schwarz has been an accredited Innosuisse mentor for more than 8 years and has accompanied more than 250 projects since then. With a background and PhD in Information Management and several years of experience working in industry she founded her own company Innovista Management in 2012 which supports companies in innovation and technology.
Get support for your innovation project: Innosuisse mentoring
Innosuisse is the Swiss Innovation Agency. Its role is to promote science-based innovation in the interest of the economy and society in Switzerland. Innosuisse supports science-based innovation projects carried out by companies, private or public institutions in cooperation with research partners. The agency also funds preliminary studies with innovation cheques as well as innovation projects conducted by research institutes without implementation partners.
To stimulate the innovation activities in times of pandemic, Innosuisse launched the additional Impulse program Swiss Innovation Power. Its objective is to maintain the innovative strength and secure the long-term competitiveness of small to medium-sized companies in Switzerland in view of the current Covid-19 pandemic. Within the scope of this impulse program, the contributions of SMEs as the implementation partners can be reduced in comparison to the standard innovation projects.
The Innosuisse Guide (https://www.innosuisse.guide/#/en) helps all interested partners to find the right support offer in just a few steps. From individual advice through to national and international networking opportunities and financial support for projects.
The innovation mentors help organizations kick-start their innovation project and find the right partners. They know the Swiss innovation scene like the back of their hand. This service is free for SMEs with less than 250 full-time employees. Applications can be submitted to Innosuisse online.
Prof. Dr. Patricia Deflorin
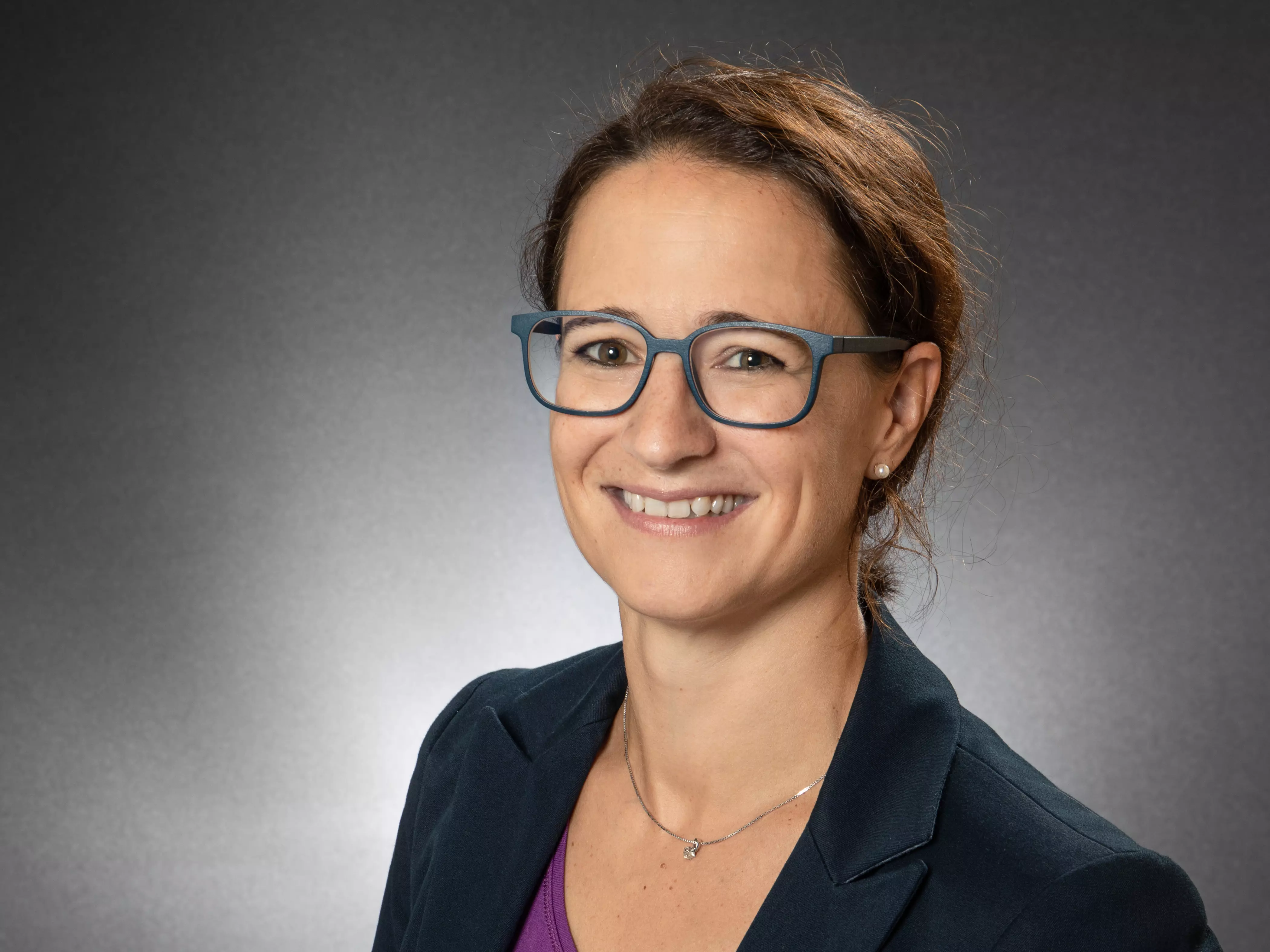
A Brief Biography
Prof. Dr. Patricia Deflorin is Research Director at the Swiss Institute for Entrepreneurship (SIFE) and lecturer at the University of Zurich. Her research focus on innovation, supply chain and digital transformation. Her area of experience includes the design and implementation of data-based services, the generation and analysis of IoT business models and the identification of necessary technologies for the successful implementation of digital transformation.
Open Innovation for Industry 4.0
Industry 4.0 provides many opportunities for industry and academy. However, Industry 4.0 often involves the implementation of new technologies and processes. Therefore, the decision in which technologies to invest is often difficult. Open innovation supports the successful implementation of Industry 4.0 as it focus on the integration of external partners into the innovation process. The cooperation with technology or data specialists enables companies to derive innovative ideas as it fosters the understanding of Industry 4.0 and the related potential. Patricia Deflorin shows how the Databooster, a NTN Innovationbooster funded by Innosuisse supports the open innovation activities as the Databooster matches industrial needs with academic knowledge.
Prof. Dr. Jörg Osterrieder
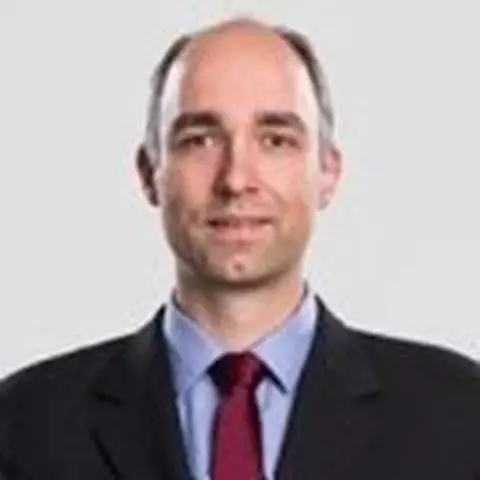
A Brief Biography
Joerg Osterrieder is Professor of Finance and Risk Modelling at the ZHAW School of Engineering (Switzerland). He has been working in the area of financial statistics, quantitative finance, algorithmic trading, and digitisation of the finance industry for more than 15 years.
Joerg is the Action Chair of the European COST Action 19130 Fintech and Artificial Intelligence in Finance, an interdisciplinary research network combining 200+ researchers and 38 European countries as well as five international partner countries. He is the director of studies for an executive education course on "Big Data Analytics, Blockchain and Distributed Ledger" and has been the main organizer of an annual research conference series on Artificial Intelligence in Industry and Finance since 2016. He is a founding associate editor of Digital Finance, an editor of Frontiers Artificial Intelligence in Finance and frequent reviewer for academic journals.
In addition, he serves as an expert reviewer for the European Commission on the "Executive Agency for Small & Medium-sized Enterprises" and the "European Innovation Council Accelerator Pilot" programmes.
Previously he worked as an executive director at Goldman Sachs and Merrill Lynch, as quantitative analyst at AHL as well as a member of the senior management at Credit Suisse Group. Joerg is now also active at the intersection of academia and industry, focusing on the transfer of research results to the financial services sector in order to implement practical solutions.
European Cooperation in Fintech and Artificial Intelligence in Finance
We will give an overview of the European COST Action 19130 Fintech and Artificial Intelligence in Finance, an interdisciplinary research network combining 200+ researchers and 38 European countries as well as five international partner countries.
The Action has three main goals: (i) transparency in FinTech, (ii) transparent versus black box decision-support models in the financial industry and (iii) transparency into investment product performance for clients. This network will bridge the gap between academia, industry, the public and governmental organizations by working in an interdisciplinary way across Europe and focusing on innovation.
Jürgen Büscher
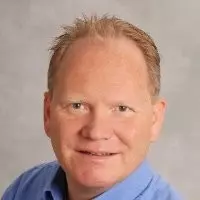
A Brief Biorgraphy
With more than 25 years of experience in the financial markets, the FX specialist Jürgen Büscher is contributing valuable experience and a broad professional network to QCAM.
As a pioneer in the development of the foreign exchange options market and the FX overlay business in Germany, he worked in leading positions at HSBC Trinkaus and Burkhardt Düsseldorf until 1995. This was followed by five years in Frankfurt as Head of Foreign Exchange at DG Bank and Head of Treasury Services & FX Overlay at ABN AMRO Bank.
Since 2001, the German has been living in Switzerland and for many years held global responsibility for the foreign exchange and commodity business at Deutsche Bank Private Wealth Management.
Registration
Please register here for the «6th European Conference on Artificial Intelligence in Industry and Finance».
Organizing Committee
- Prof. Dr. Wolfgang Breymann
- Prof. Dr. Patricia Deflorin
- Prof. Dr. Rudolf Füchslin
- Dr. Andreas Henrici
- Prof. Dr. Jörg Osterrieder
- Prof. Dr. Peter Schwendner
- Prof. Dr. Dirk Wilhelm