Deep neural networks are cheating
How do state-of-the-art models for automatic speaker recognition learn to recognize voices? A team of UZH and CAI researchers catches them "in flagranti": more comprehensive dynamic-prosodic features are not even learnt when forced.
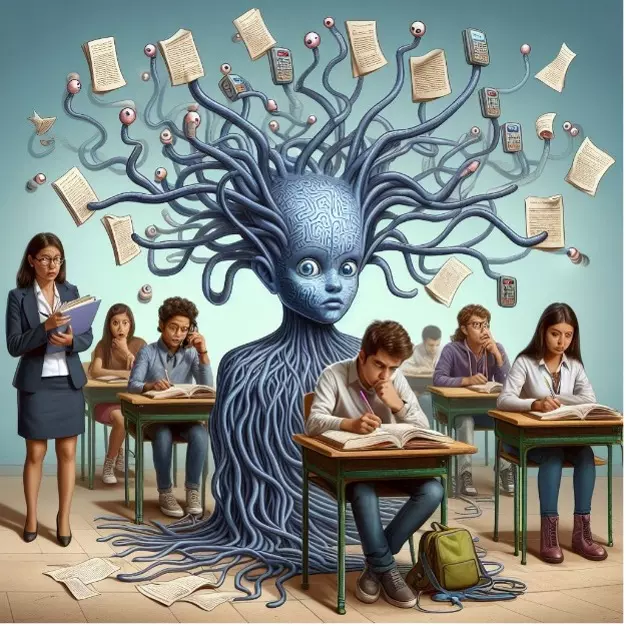
In a recent article in Patter Recognition Letters, our MSc alumn Daniel Neururer, University of Zurich Professor of Phonetics and Phonology Prof. Dr. Volker Dellwo, and our Prof. Dr. Thilo Stadelmann analyze how state-of-the-art models for automatic speaker recognition learn to recognize voices. The team finds that models cheat: they deliberately take the easiest route, completely avoiding to learn the more difficult patterns, even when forced.
This insight is significant: It shows a clear path for research by pointing out what specifically needs to be improved for better speaker recognition.
Here's the abstract:
While deep neural networks have shown impressive results in automatic speaker recognition and related tasks, it is dissatisfactory how little is understood about what exactly is responsible for these results. Part of the success has been attributed in prior work to their capability to model supra-segmental temporal information (SST), i.e., learn rhythmic-prosodic characteristics of speech in addition to spectral features. In this paper, we (i) present and apply a novel test to quantify to what extent the performance of state-of-the-art neural networks for speaker recognition can be explained by modeling SST; and (ii) present several means to force respective nets to focus more on SST and evaluate their merits. We find that a variety of CNN- and RNN-based neural network architectures for speaker recognition do not model SST to any sufficient degree, even when forced. The results provide a highly relevant basis for impactful future research into better exploitation of the full speech signal and give insights into the inner workings of such networks, enhancing explainability of deep learning for speech technologies.