Jan-Egbert Sturm
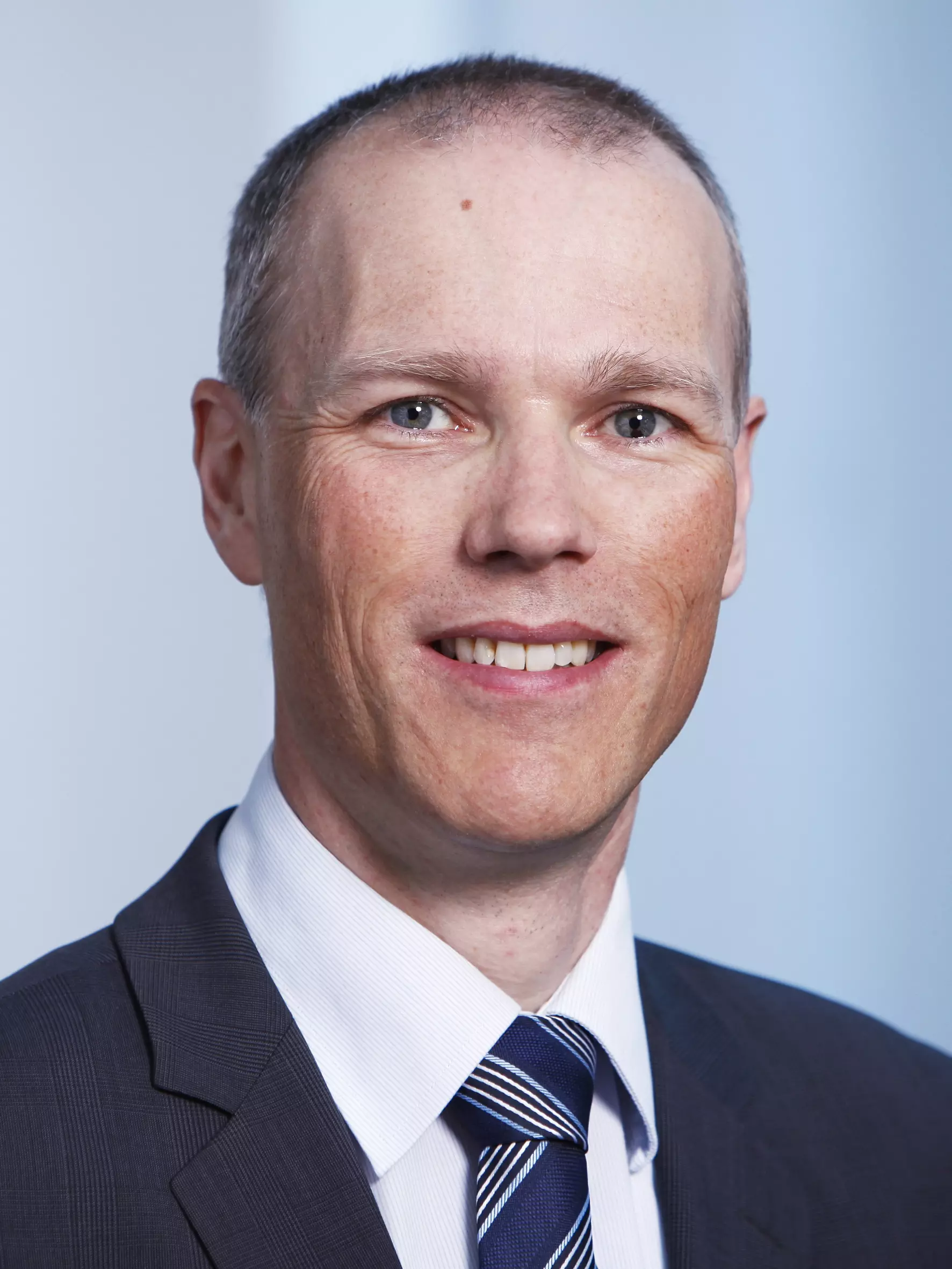
The Use of Ever Increasing Datasets in Macroeconomic Forecasting
Slides (PDF, 452 KB)(PDF 451,9 KB)
Abstract – KOF Swiss Economic Institute is probably best known for its forecasting activities. Besides by macroeconomic theory, these forecasts are largely driven by ever bigger datasets. Whereas traditionally microeconomic, i.e. firm- and household-specific, information is first aggregated to the macroeconomic, i.e. national, level data before macroeconomic relationships are estimated and used in producing such forecasts, there is a clear tendency to more and more analyse macroeconomic relationships using micro data more directly. This sometimes allows new insights that cannot be achieved by looking at solely macroeconomic data.
An example of this is given by the KOF Surprise Indicator. It is based on the semantic identification of survey answers of the KOF survey in the Swiss manufacturing industry. By using the firm-specific answers, it can estimate to what extent each firm has been surprised during the previous quarter. By subsequently aggregating this information, the KOF Surprise Indicator identifies the growth path of the Swiss economy and allows for accurate estimates of the current growth rate of the Gross Domestic Product (GDP).
Data limitations still make such examples in which microeconomic data are directly used in producing a macroeconomic indicator rare. Nevertheless, also the substantial increase in macroeconomic time series allows for the use of more sophisticated techniques to aggregate information. Since 1976, the KOF Swiss Economic Institute has been publishing a leading indicator, the KOF Economic Barometer, predicting how the Swiss economy will develop in the near future. Whereas in the previous versions of the KOF Barometer six to 25 variables were selected based on expert knowledge, the 2014 version contains over 200 variables selected out of a set of close to 4500. For that a relatively automated and robust selection procedure has been developed. This procedure is re-run every autumn allowing the Barometer to ‘learn’. Subsequently, the selected variables are subsequently transformed into one leading indicator. The increased number of variables allows a – compared to its previous versions – relatively stable and robust KOF Barometer that hence no longer has to rely on filtering techniques to reduce the noise in the final indicator.
Biography – Jan-Egbert Sturm is Professor of Applied Macroeconomics as well as Director of the KOF Swiss Economic Institute at the ETH Zurich. He was researcher at the University of Groningen, The Netherlands, until 2001, and held various positions as professor and director at universities und research centers worldwide. As a international authority in economics, he is best known by a broader audience for his regular appearances on television, radio and in newspapers.
In his research, Jan-Egbert Sturm relies heavily on empirical methods and statistics. He has a focus on monetary economics, macroeconomics as well as political economy and with a special interest in fields which are closely related to practical and current problems. His applied studies have focused on, for example, economic growth and central bank policy. He has published several books, contributed articles to various anthologies and internationally renowned journals.
Jan-Egbert Sturm headed the Ifo research team at the Joint Analysis of the Six Leading German Economic Research Institute, 2001-2003. Since 2001 he has been member of the CESifo Research Network and since 2003 Research Professor at the Ifo Institute. In 2005 he was appointed to the European Economic Advisory Group of the CESifo. In 2006 he was appointed member of the user advisory council of the Ifo Institute. At the beginning of 2007, he became President of the Centre for International Research on Economic Tendency Surveys (CIRET). Since 2013 he is editor of the European Journal of Political Economy. Currently he is member of several advisory commissions in Switzerland.