Research Centre for Cognitive Computing in Life Sciences
With cognitive computing, we develop new software technology, algorithms, and systems for signal processing, data analytics and process control in life sciences.
About us
The Research Centre for Cognitive Computing in Life Sciences develops and applies computational methods and models inspired by learning and self-organisation principles of biological neural systems to problems in life sciences, such as activity recognition, bio-signal processing, environment monitoring and assistive autonomous systems.
Methods we use
Combination of model-based approaches and data-driven machine learning:
Self-organizing and complex systems
- Dynamical systems, cellular automata, physics-constrained DL
Neuromorphic computing
- Event-based vision, neural motion planning and control, autonomous learning, power-efficient AI, real-time AI
Classical and deep learning based machine learning
- NLP, Generative AI, Multi-modal modelling, explainable AI
- Reinforcement learning, Bayesian modelling, predictive analytics, signal processing
Examples of application domains
- Environmental systems and sustainability
- Remote sensing
- Spectral analysis in food industry and material science
- Protein engineering
- Process automation
- Predictive maintenance
- Bio-signal analysis and sports analytics
- Smart farming
- Collaborative and assistive robotics
Advanced Signal Analytics
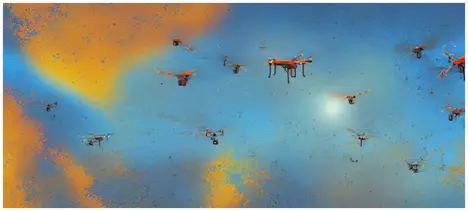
This research group works on the analysis, modelling and classification of signals as as well as statistical modelling and consulting. Our experts use physical and statistical models as well as deep learning applied to problems in the life sciences such as agricultural systems, chromatography or signals from drones. The group consists of data science and statistics experts with backgrounds in theoretical and applied physics and mathematics with a long experience of research and teaching in the life sciences.
Group leader: Dr. Matthias Nyfeler | Learn more about the research group Advanced Signal Analytics
Computational Environment
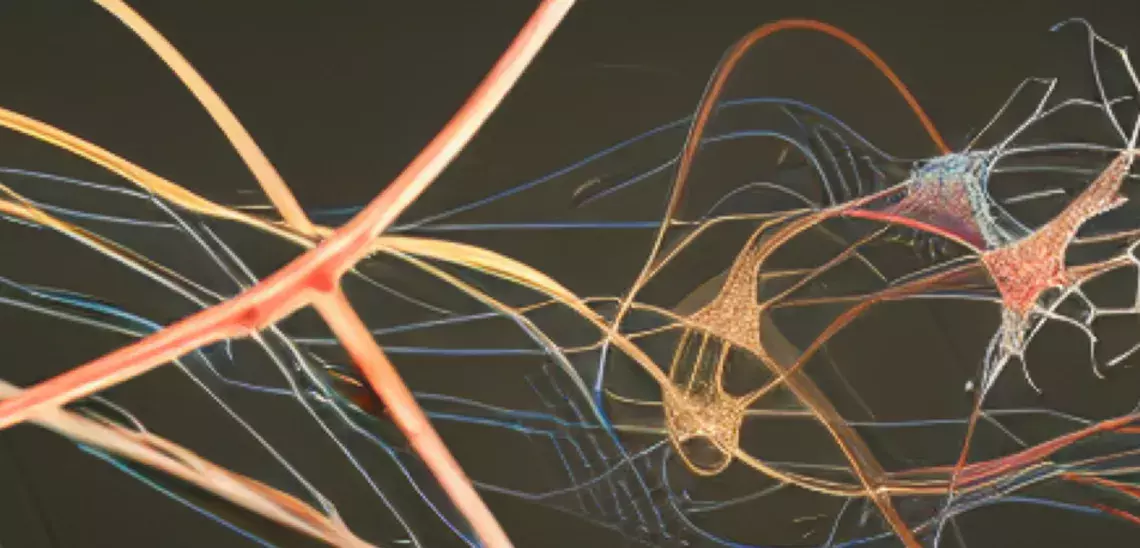
The research group focuses on the modeling of natural systems and their interaction with humans. This also includes sustainability topics in a more general context such as in view of social and economical questions. Our experts approach the challenges with data science, deep learning and modeling. A special methodological focus is on deep learning methods, multimodal learning with natural language processing and on modeling with discrete systems such as cellular automata.
Group leader: Dr. Martin Schüle | Learn more about the research group Computational Environment Group
Neuromorphic Computing
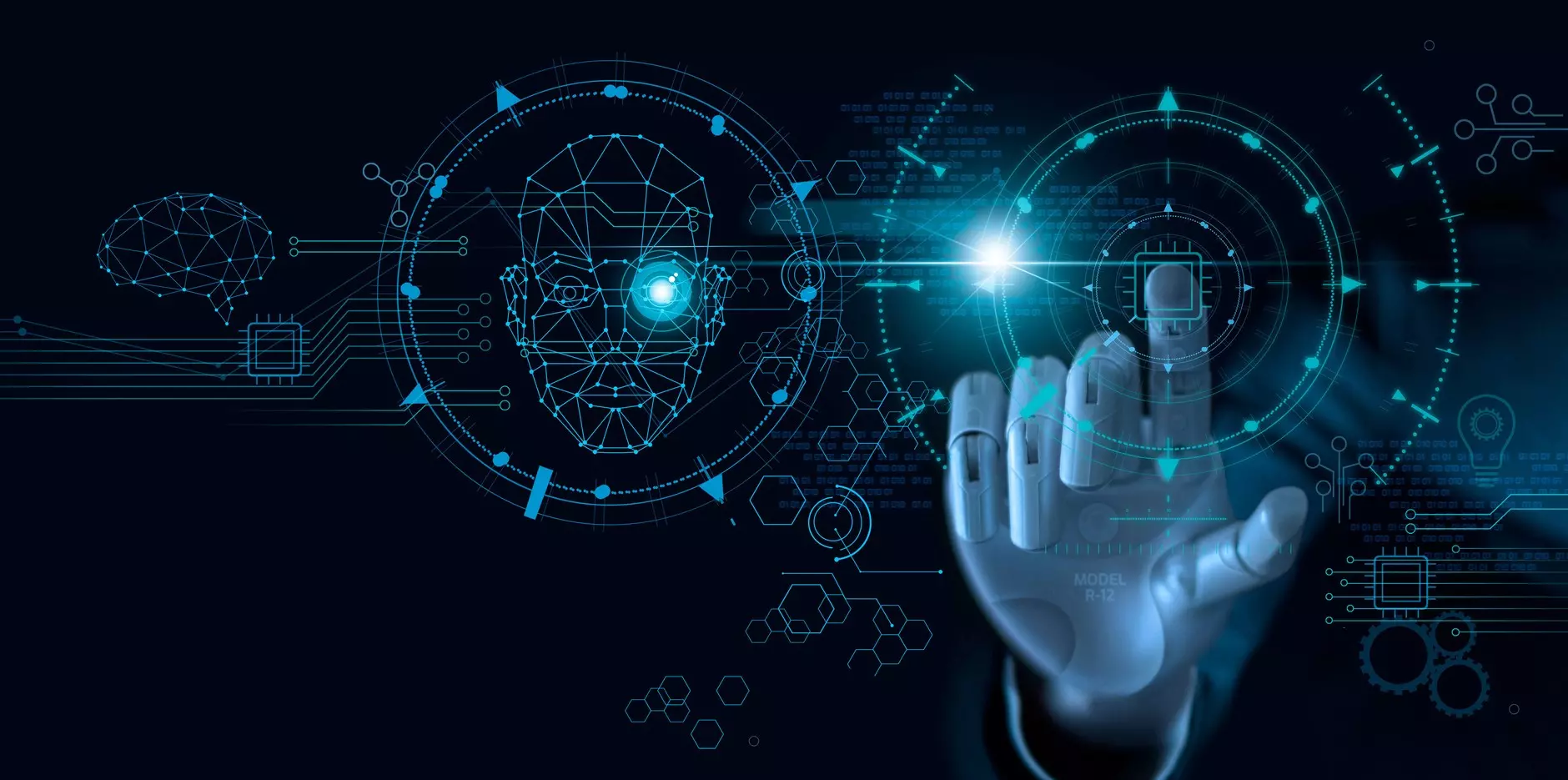
The research group develops advanced neural-network based algorithms, software libraries, and systems with the new generation of computing chips – brain-inspired neuromorphic sensing and computing hardware. Our experts focus on perception, motion planning, and control for robotic actuators with applications in there fields: healthcare, agriculture, food processing, and smart environments. Our research group follows a human-centered design approach to develop new generation of physical AI systems that are power-efficient, adaptive, and safe.
Group leader: Prof. Dr. Yulia Sandamirskaya | Learn more about the research group Neuromorphic Computing
Predictive Analytics
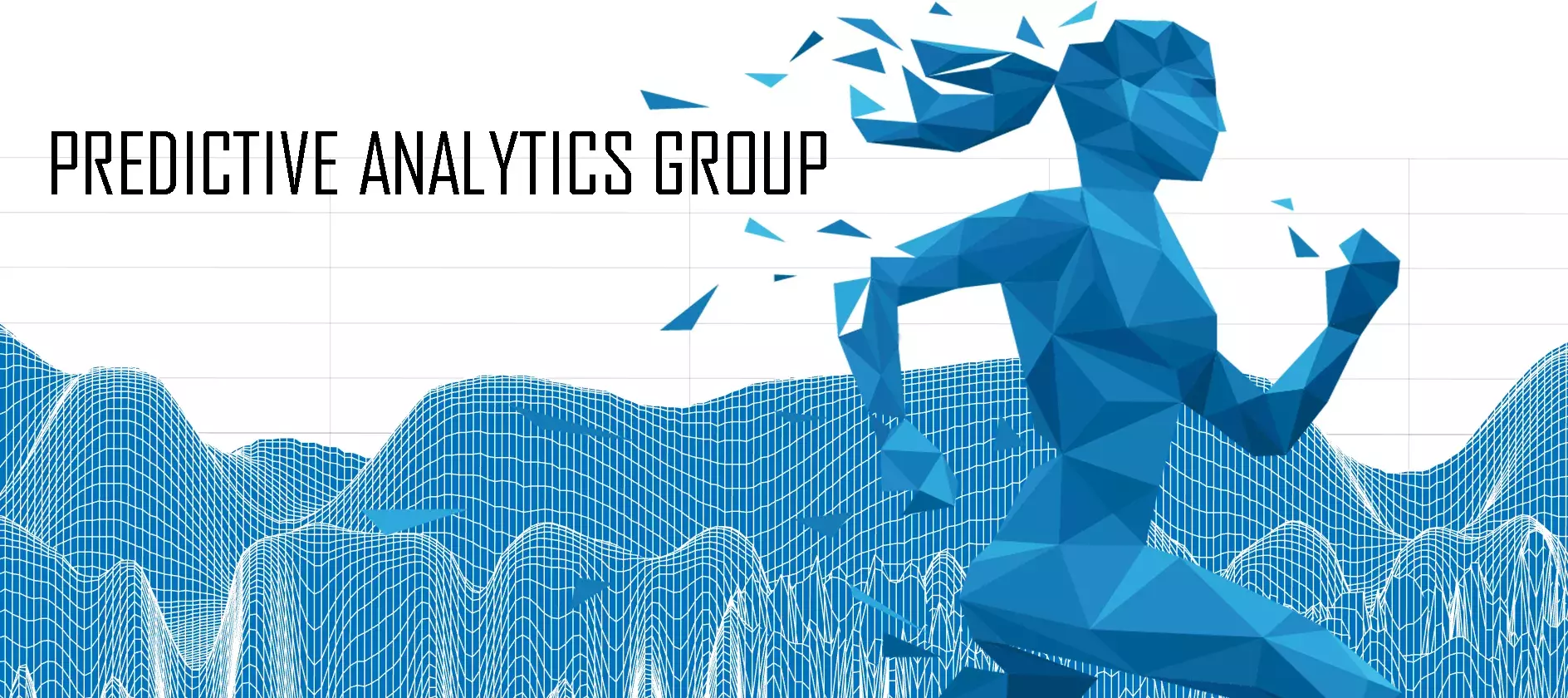
The research group's focus is on applied research in statistical modeling and machine learning for pattern discovery, as well as data mining, pattern recognition, and forecasting in life sciences. Our experts have a proven track record in the areas of med-tech, personalized health and sports analytics. The group's expertise lies in the fusion of heterogenous information sources and ensemble methods, in particular for time series and image/video analytics. In the context of Industry 4.0, the group conducts research in the area of predictive and prescriptive maintenance.
Group leader: Dr. Krzysztof Kryszczuk | Learn more about the research group Predictive Analytics
Teaching Activites
The centre includes teaching engagements at BSc and MSc level as well as in continuing education. At BSc level, basic subjects in mathematical and physical modelling, statistics and information processing are offered in all study programmes of the department. Furthermore, teaching includes specific subjects in the field of data science with a focus on "machine learning, neural networks, signal and image analysis" in the institute's own BSc ADLS and MSc ACLS programmes and in continuing education. Thirdly, specific subjects are offered, especially in the "Digital Environment" specialisation of the ADLS.
Projects
-
Stability of self-organizing net fragments as inductive bias for next-generation deep learning
We recently released "A Theory of Natural Intelligence", proposing a possible key to the emergence of intelligence in biological learners. Goal of this fellowship is to develop a technical implementation of the concept of self-organizing netfragments within contemporary deep artificial neural nets. ...
-
Investor and Stakeholder Tools for Tracking Companies’ Climate Commitments, Greenwashing and ESG Trends
In this project, we are developing science-based methods to systematically identify potential greenwashing in corporate communications as well as signals of green innovations and technologies. By applying advanced AI methods to thousands of stocks, we aim to develop novel, ESG-compliant financial products. ...
-
An experimental framework to allow evidence-based sustainability policymaking
This research project evaluates the feasibility of using a mathematical decision framework (based on the 2019 Nobel laureates in Economy who developed an experimental approach for improving policy in poverty through field experiments) in sustainability policy, and achieving a software-supported and data-driven ...
-
Drone Alarm
-
Reinforcement Learning Analysis Framework
The aim of this project is to implement a framework that facilitates the development of RL solutions for real-world applications. This is necessary since the academic literature usually focuses on specific algorithms and approaches differ widely for different regions in the highly complex RL problem space. ...
Publications
-
Epper, Pascale; Glüge, Stefan; Vidondo, Beatriz; Wróbel, Anna; Ott, Thomas; Sieme, Harald; Kaeser, Rebekka; Burger, Dominik,
2023.
Increase of body temperature immediately after ovulation in mares.
Journal of Equine Veterinary Science.
127(104565).
Available from: https://doi.org/10.1016/j.jevs.2023.104565
-
Glüge, Stefan; Balabanov, Stefan; Koelzer, Viktor Hendrik; Ott, Thomas,
2023.
Evaluation of deep learning training strategies for the classification of bone marrow cell images.
Computer Methods and Programs in Biomedicine.
(243), pp. 107924.
Available from: https://doi.org/10.1016/j.cmpb.2023.107924
-
Horn, Claus; Nyfeler, Matthias; Müller, Georg; Schüpbach, Christof,
2022.
Drone radio signal detection with multi-timescale deep neural networks [paper].
In:
Yurish, Sergey Y., ed.,
Proceedings of the 4th International Conference on Advances in Signal Processing and Artificial Intelligence.
4th International Conference on Advances in Signal Processing and Artificial Intelligence (ASPAI), Corfu, Greece, 19-21 October 2022.
IFSA Publishing.
pp. 140-143.
Available from: https://doi.org/10.21256/zhaw-27185
-
Müller, Antonia; Glüge, Stefan; Vidondo, Beatriz; Wróbel, Anna; Ott, Thomas; Sieme, Harald; Burger, Dominik,
2022.
Increase of skin temperature prior to parturition in mares.
Theriogenology.
190, pp. 46-51.
Available from: https://doi.org/10.1016/j.theriogenology.2022.07.007
-
Rerabek, Martin; Schiboni, Giovanni; Durrer, Lukas; Oliveras, Ruben; Eib, Philippe; Rouchat, Fabien; Probst, Anja; Schmidt, Markus; Kryszczuk, Krzysztof,
2022.
Circadian rhythm tracking using core body temperature estimates from wearable sensor data.
In:
7th International Conference on Human Interaction and Emerging Technologies (IHIET), Lausanne, Switzerland, 23-25 April 2022.