New Swiss German Speech-to-Text Corpus Launched at ACL 2023
We present the STT4SG-350 (Speech-to-Text for Swiss German) corpus, which consists of 343 hours of audio with transcripts. The corresponding paper was accepted at ACL 2023.
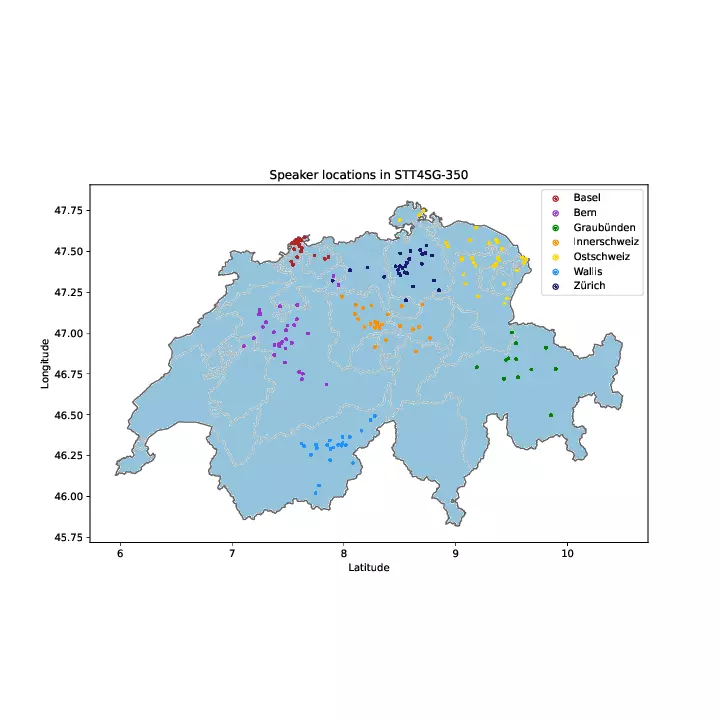
We are thrilled to announce the release of the STT4SG-350 (Speech-to-Text for Swiss German) corpus, an ambitious effort that contributes significantly to Swiss German linguistic research and technology. The corpus, published at the Association for Computational Linguistics (ACL) conference in 2023, features 343 hours of Swiss German speech data, annotated with Standard German text. This corpus is the result of the SNF Project: “End-to-End Low-Resource Speech Translation for Swiss German Dialects”, a collaboration between ZHAW, FHNW, and UZH.
The STT4SG-350 corpus, the largest annotated public Swiss German speech corpus to date, is the product of our dedicated efforts to expand the horizons of Swiss German linguistic technology. To gather the data, speakers were shown Standard German sentences in a web app, which they then translated into Swiss German and recorded. Spanning all Swiss German dialect regions, the corpus reflects a broad cross-section of Swiss society. It includes data from 316 speakers of all age groups, with an equal gender representation. Importantly, each dialect region is represented with approximately equal amounts of speech data, ensuring that the corpus is an excellent resource for comparative studies and dialect-specific technologies.
This extensive collection of Swiss German speech is a treasure trove for many areas of linguistic research and development. Its potential applications span automatic speech recognition (ASR), text-to-speech conversion, dialect identification, speaker recognition, and many more. Furthermore, we have carefully curated training, validation, and test splits of the data to support rigorous experimentation and evaluation. The test set, in particular, is composed of the same sentences spoken in each dialect region, facilitating a fair evaluation of technology across different dialects.
The strength of our corpus is not just in its size and diversity, but also in its quality. We trained an ASR model on the STT4SG-350 training set and achieved an impressive average BLEU score of 74.7 on the test set. Notably, this model outperformed the best published scores on two other Swiss German ASR test sets, underscoring the robustness of our corpus.
In the spirit of open research and collaboration, we are making the STT4SG-350 corpus available for research and commercial purposes.