Improving Enzyme Fitness with Machine Learning
A new publication from the Buller-Lab looks at how machine learning can be used to support enzyme engineering
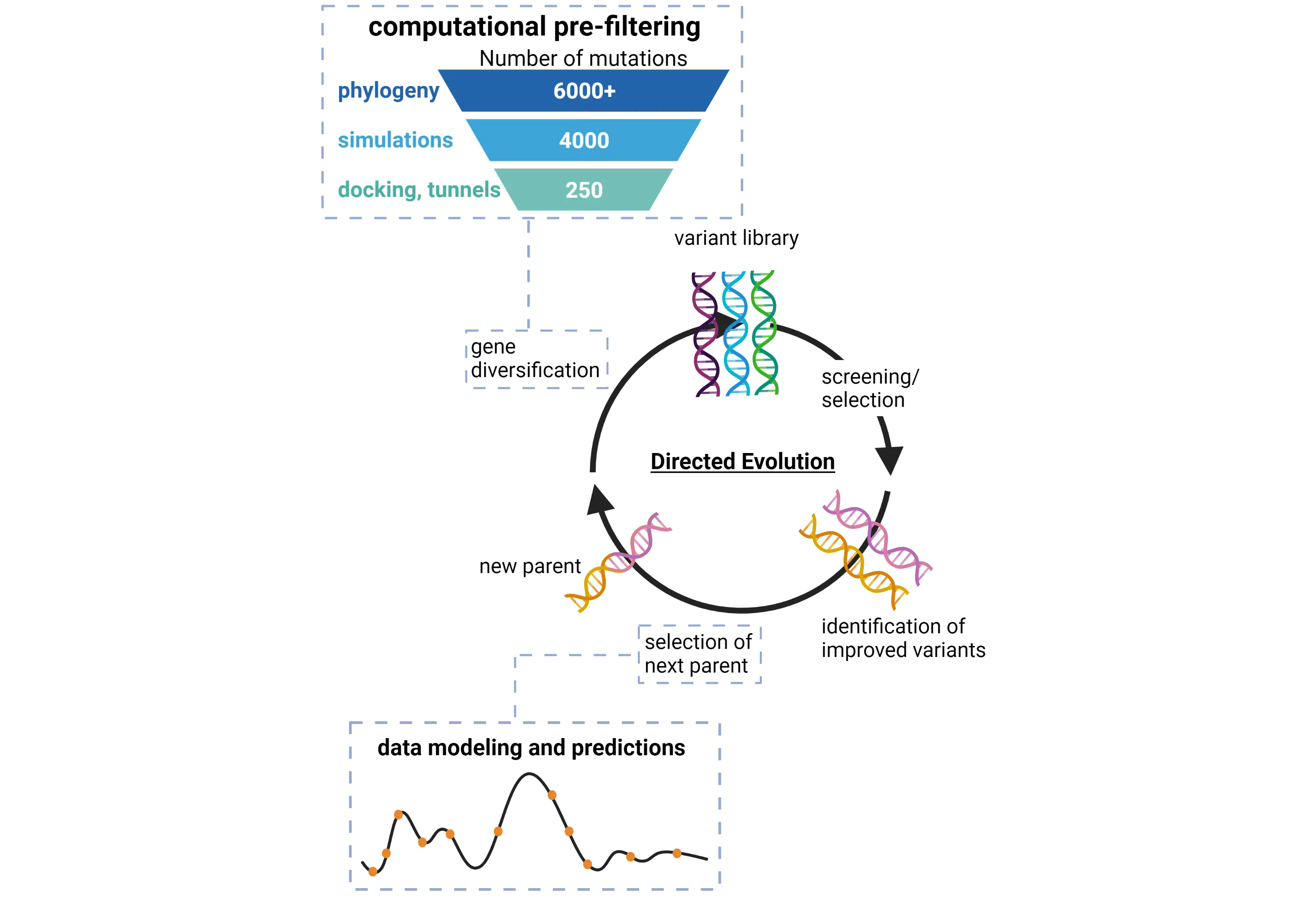
In nature, enzymes catalyze complex reactions with high specificity and selectivity. This potential is increasingly being exploited for the catalysis of reactions in organic chemistry. However, wild-type enzymes are often not stable and/or efficient enough under industrial conditions. Techniques developed in recent decades, such as directed evolution, are now routinely used to optimize enzyme properties. However, cost, time, and screening capacity limit the number of variants that can be tested with directed evolution to a relatively small number of possible combinations. Machine learning, on the other hand, can cover the sequence space more comprehensively. The article presents examples of how machine learning can be used to more efficiently improve enzyme properties such as stability, activity, and regio- and enantioselectivity by designing “smart” enzyme libraries.
Reference:
Improving Enzyme Fitness with Machine Learning D. Patsch & R. Buller. CHIMIA (2023) 77, 116-121;. https://doi.org/10.2533/chimia.2023.116