Unequal treatment through facial recognition procedures
ZHAW researchers are discovering that approaches aimed at reducing biases, i.e. the unequal treatment of different social groups, in facial recognition procedures do not lead to better results.
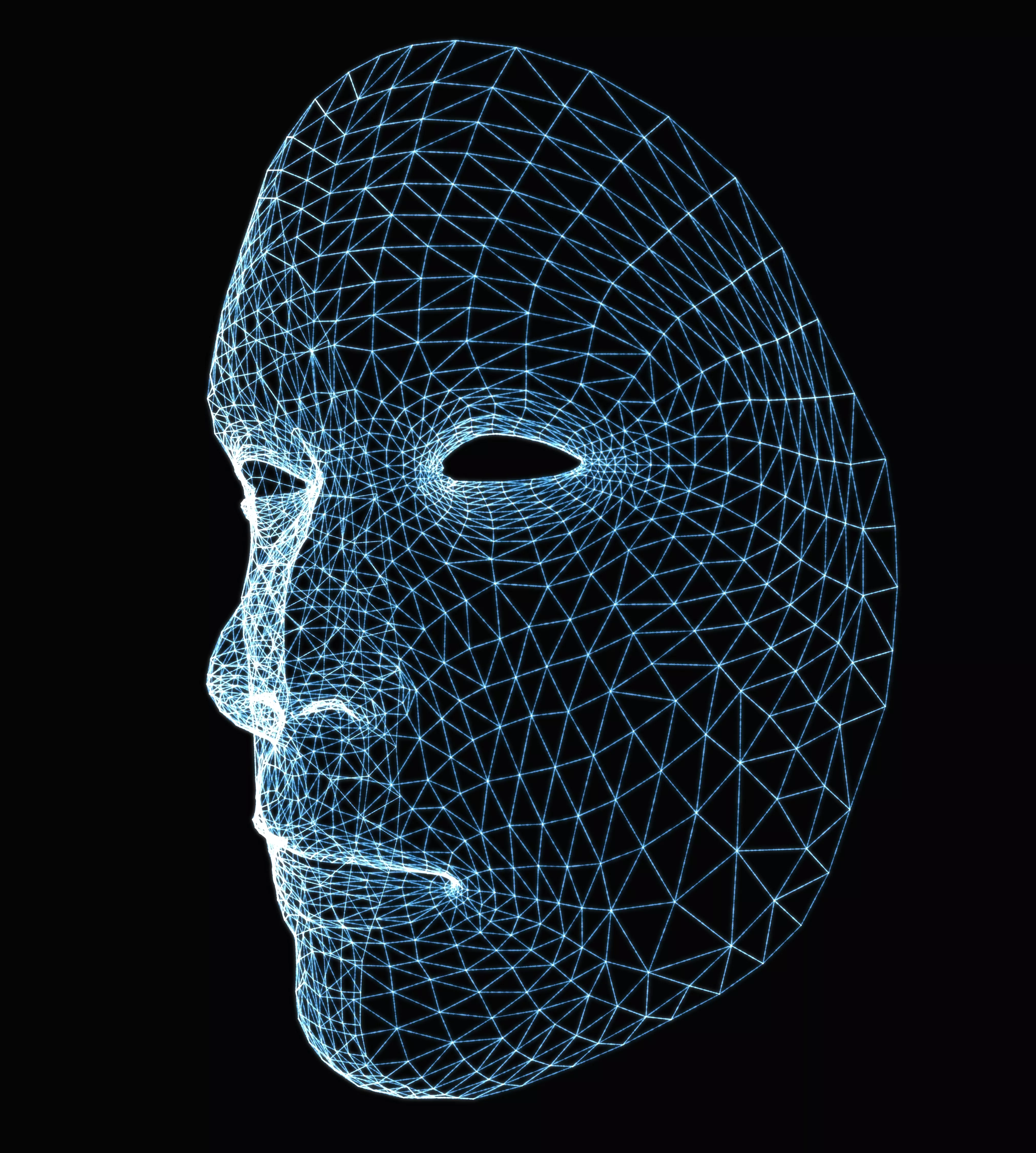
For many people, facial recognition is already part and parcel of everyday life, for example when unlocking their smartphone. This technology is also increasingly being used to combat crime, allowing suspected individuals to be identified with the help of surveillance cameras. The problem here is that, in addition to the increase in surveillance, modern facial recognition procedures are highly susceptible to biases. In other words, they do not work equally as well for different social groups. A team at the ZHAW has now discovered that approaches already being used to make these procedures less susceptible to biases do not work in practice.
Facial recognition procedures do not treat everyone equally
The aim of facial recognition is to “match” faces, i.e. to identify different images of the same person as being just that. This procedure is used to verify that the face in front of a smartphone’s camera actually belongs to the user when an attempt is made to unlock the device. In 2018, a study revealed that modern facial recognition procedures primarily work well for men and people with a light skin colour. In particular, men with light skin are almost always identified correctly. However, the level of accuracy was especially low for women with dark skin.
The ZHAW researchers also identified this bias in their experiments and noted that there are significant differences between the groups in terms of the errors made. This is particularly problematic when the technology is used for purposes such as the identification of suspects via facial recognition. There are two types of errors here: the first is that an individual is incorrectly identified as a suspect and the second is that a suspected individual is not recognised as such. During the experiments, errors of the second type predominated for white individuals, while for non-white people it was the first kind of error that occurred most frequently. As a result, it can be particularly common for non-white individuals to be arrested without justification. In order to test how these different levels of errors can be avoided, the researchers at the ZHAW have applied the method of blinding. In doing so, the aim was to make the facial recognition procedures less susceptible to certain characteristics, such as skin colour and gender. It was hoped that facial recognition technology would become less influenced by biases, meaning that it would work better for all groups. The fact that this approach did not work as assumed and therefore does not lead to bias-free facial recognition was reported by the ZHAW researchers in the study “Bias, awareness, and ignorance in deep-learning-based face recognition”. The study participants included Samuel Wehrli from the ZHAW School of Social Work, Corinna Hertweck from the ZHAW School of Engineering as well as Mohammadreza Amirian and Thilo Stadelmann from the ZHAW Centre of Artificial Intelligence and Stefan Glüge from ZHAW School of Life Sciences and Facility Management.
Susceptibility to biases despite blinding
The researchers discovered that despite the preset reduction in the ability to recognise sensitive characteristics such as ethnicity and gender, there was little change in the accuracy of the facial recognition. As was the case before, fewer errors were made for white people than was the case with other groups.
For Corinna Hertweck, the reason for this is obvious: “The individual facial recognition models are too poorly trained.” This means that the models have simply been supplied with too little data from different sociodemographic groups, making them susceptible to biases when used. While there have already been attempts to rectify these data gaps, many data collections still pay too little attention to ensuring the broad representation of all sociodemographic groups. When collecting training data for facial recognition procedures, a conscious effort must therefore be made to ensure greater diversity. Otherwise, the ZHAW researchers are sure that facial recognition technologies will in future remain susceptible to biases.
However, the ZHAW researchers also point out that politicians and society in general have to ask themselves in what areas and to what extent facial recognition should even be used in everyday life and how the misuse of this technology can be effectively prevented. The researchers believe that these questions need to be discussed and answered in order to ensure that facial recognition does not lead to discrimination against certain sociodemographic groups, but rather contributes to benefiting society as a whole.