Pilot study machine learning for injection molding processes
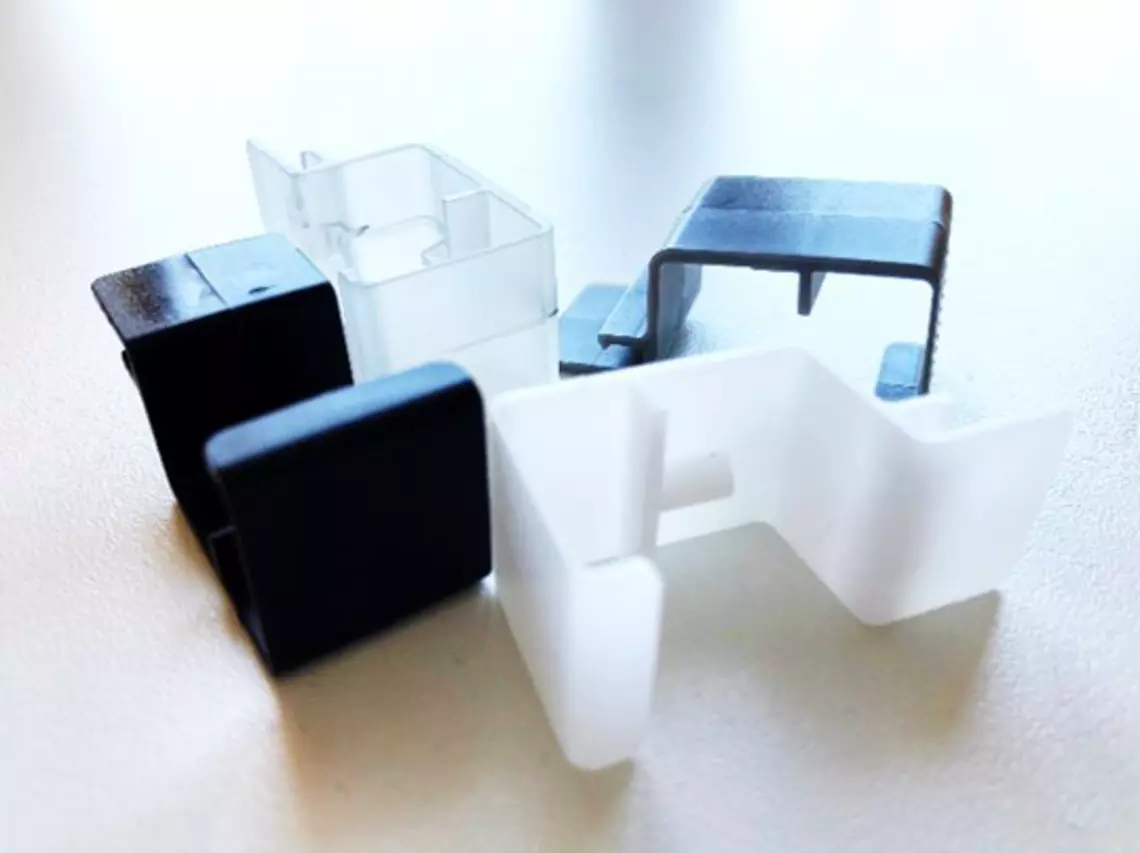
At a glance
- Project leader : Prof. Dr. Thilo Stadelmann
- Project team : Raphael Emberger, Prof. Dr. Matthias Rosenthal
- Project budget : CHF 11'000
- Project status : completed
- Funding partner : Innosuisse (NTN Innovation Booster), Third party (Kistler Instrumente AG)
- Project partner : Kistler Instrumente AG
- Contact person : Thilo Stadelmann
Description
Researchers from the CAI and InES conduct a technical deep dive together to explore the possibilities of capturing process knowledge on injection molding in deep neural networks and transfer the results to novel usage scenarios.The groups of Prof. Stadelmann (Computer Vision, Perception & Cognition, ZHAW CAI) and Prof. Rosenthal (Realtime Platforms, ZHAW InES)- recently joined forces with Kistler Innovation Lab to research risks and possibilities for improving automatic quality control and process supervision in plastic injection molding with the help of advanced machine learning. Specifically, transfer learning / domain adaptation and continual learning in neural networks for small data scenarios will be evaluated. The team secured funding for a technical deep dive from the NTN Databooster of the Data Innovation Alliance and Innosuisse for a three months study. Its results will influence the design and implementation of Kistler’s future products in a subsequent joint project.The NTN Databooster supports data-driven innovation by helping companies find matching research partners for their challenges, to brainstorm for fresh solutions, evaluate feasibility and risks in technical deep dives and ultimately apply for respective joint R&D project funding. CAI staff is active in the Databooster and its host organization, the Data Innovation Alliance in various roles since its foundation and has successfully concluded several R&D projects within its framework.