ZHAW students win regional Siemens Excellence Award with work on AI model for improving thyroid cancer diagnosis
Computer science graduates Tenzin Samdrup Langdun and Martin Oswald win the regional Siemens Excellence Award, endowed with 4'000 Swiss Francs, for their bachelor's thesis titled "Deep-Learning-Based Cell Segmentation for the Detection of Thyroid Cancer in Single Cells." With this award, they are nominated for the national Siemens Excellence Award 2024.
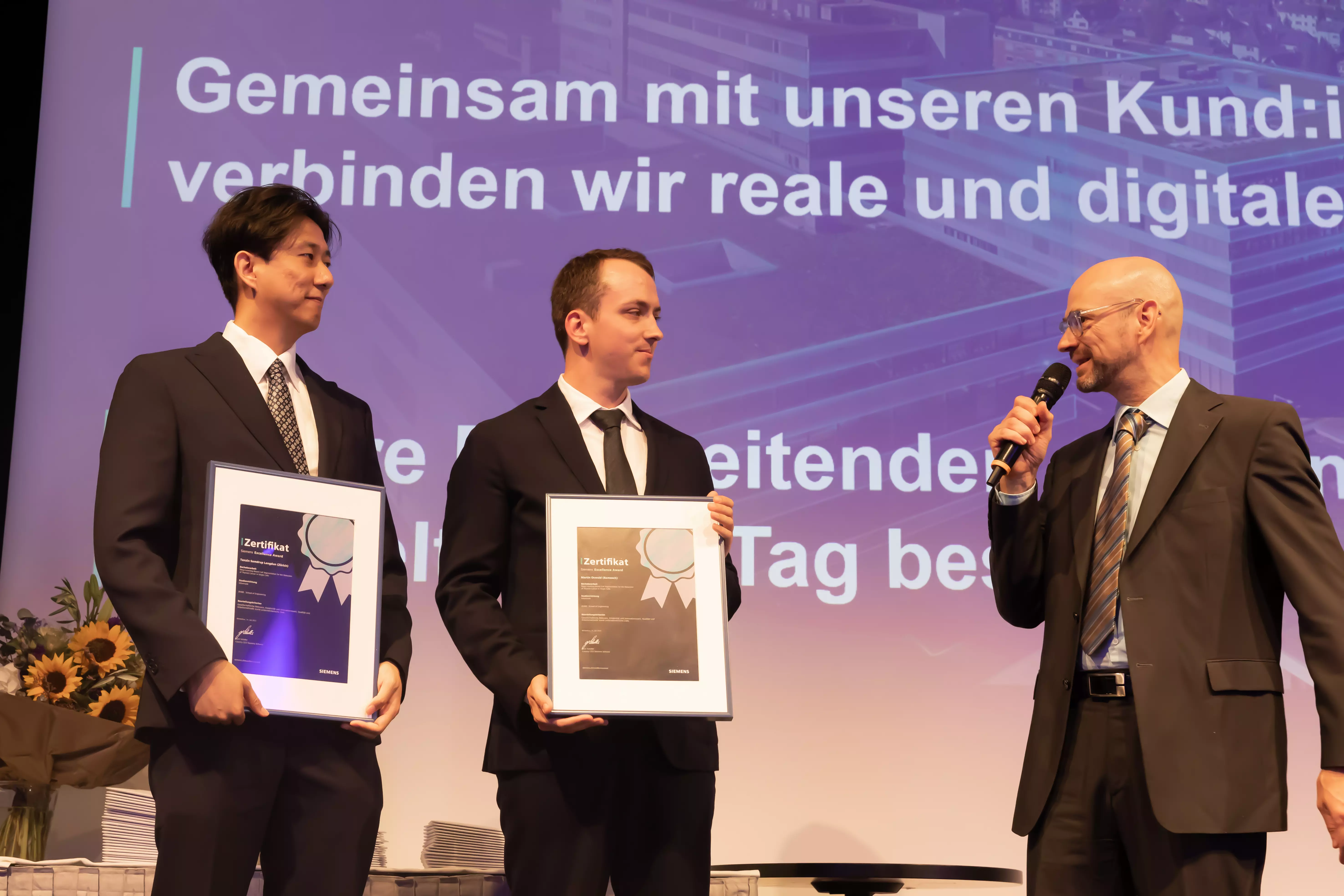
In the diagnosis of thyroid cancer, manual segmentation of fluorescence polarization images (Fpol) poses a challenge due to its considerable time requirements, hindering its widespread application. Hence, the two recent computer science graduates, Tenzin Samdrup Langdun and Martin Oswald, developed a deep learning model based on U-Net for automatic cell segmentation in their bachelor's thesis. Their model focuses on overcoming the limitations of manual image segmentation and improving the previously error-prone analysis of biological images by humans. By incorporating ambiguously labeled images, the model's performance is significantly enhanced.
Co-supervisor Prof. Dr. Thilo Stadelmann explains, "Humans learn very well from imprecise information: incomplete data, only sketchy descriptions instead of perfect information. To have made such data usable for a specific application of machine learning is the essential achievement of Martin and Tenzin, which was rightfully rewarded here with remarkable success."
Similarly, the two ZHAW graduates explored the use of Semi-Supervised Active Learning (SSAL), in which their AI model is trained using pseudo-labeled images. Initially, a deterioration in segmentation performance was observed. However, manual correction of pseudo-labeled images led to a slight improvement in image segmentation while simultaneously reducing the average time required for labeling image data by 65 percent. Overall, this automated approach reduced the time required for manual segmentation and calculation of Fpol values by over 99.7 percent. An impressive result, showcasing the enormous potential for development in the application of the SSAL framework in thyroid cancer diagnostics.