7th European COST Conference on Artificial Intelligence in Industry and Finance
We would like to welcome you to the «7th European COST Conference on Artificial Intelligence in Industry and Finance», hosted by the Institute of Applied Mathematics and Physics (IAMP), the Centre for Artificial Intelligence (CAI), the Institute of Data Analysis and Process Design (IDP) at the School of Engineering, and the Institute of Wealth & Asset Management (IWA) at the School of Management and Law of the Zurich University of Applied Sciences (ZHAW) in Winterthur, Switzerland.
Artificial Intelligence in Industry and Finance (7th European Conference on AI in Industry and Finance in Switzerland)
September 28, 2022, 9:45-17:15
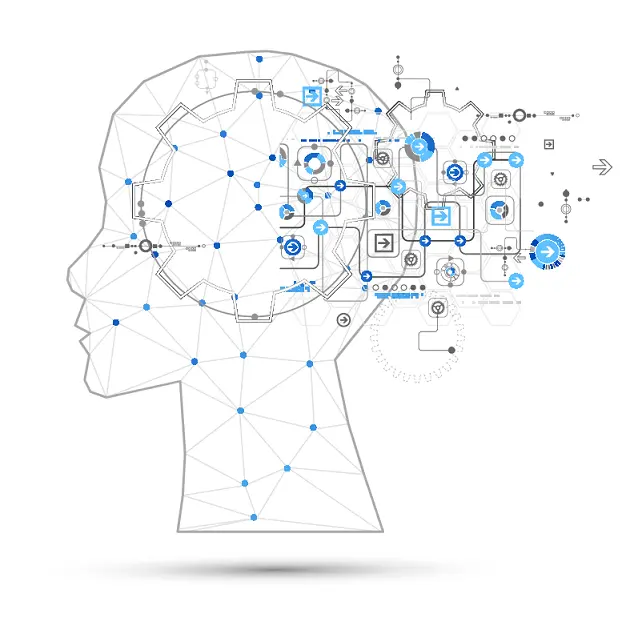
Information at a glance
- Conference Date: Wednesday, September 28, 2022
- Conference Location: ZHAW Winterthur
- Conference Topics: Artificial Intelligence in Industry and Finance
- Keynote Speaker: Dr. Peter Mattson, Google
- Conference Flyer(PDF 300,3 KB)
- Conference Program(PDF 279,1 KB)
- Program booklet(PDF 2,1 MB)
- Related Journal: "Frontiers in Artificial Intelligence"(PDF 266,5 KB)
- Funding: This conference is funded by the Innosuisse (Swiss Innovation Agency) Networking Event Series, and is an event in the Networking Event Series – Artificial Intelligence in Industry and Finance.
Aim of the conference
The aim of this conference is to bring together academics, young researchers, students and industrial practitioners to discuss the application of Artificial Intelligence in Industry and Finance.
The 1st COST Conference was held on September 15, 2016, the 2nd COST Conference was held on September 7, 2017, the 3rd COST Conference was held on September 6, 2018, the 4th AI Conference was held on September 5, 2019, the 5th AI Conference was held on September 3, 2020, and the 6th AI Conference was held on September 9, 2021.
Related Conferences
-
1st International Workshop on Automated Regulatory Financial Reporting (September 29, 2022, ZHAW Winterthur)
Thematic Sessions
- Artificial Intelligence in Finance: Artificial Intelligence and Fintech challenges for the banking and insurance industry
- Artificial Intelligence in Industry: Artificial Intelligence challenges for companies from the mechanical and electrical industry, but also life sciences.
- Ethical Questions in Artificial Intelligence: Issues arising in AI applications such as trust, explainability, neutrality, responsibility, moral consequences of algorithmic decisions.
- Academia-Industry Best Practices: General discussion of issues arising in academia-industry cooperations, and the role of Innosuisse in supporting these cooperations
Keynote Speaker
Peter Mattson, Google
Invited Speakers
Artificial Intelligence in Industry
- Martin Keller, Acodis
- Ekaterina Sirazitdinova, NVIDIA
- Armando Geller, Scensei
- Martina Perani, FFHS
- Giulia Fischetti, Univ. Venezia
- Sebastian Welter, IKEA
- Nikola Pascher / Thilo Stadelmann, Mettler Toledo/ZHAW
Artificial Intelligence in Finance
- Fabian Woebbeking, Axiomatec/Univ. Frankfurt
- Blanka Horvath, Oxford University
- Dominik Boos / Linus Grob, Avena Capital/ZHAW
- Thomas Krabichler, FH OST: "Deep Asset-Liability-Management"(PDF 1,7 MB)
- Matthias Niggli / Christian Rutzer, Univ. Basel: "What is it worth? Predicting the Importance of Patents Using Supervised Learning"(PDF 861,5 KB)
- Ken Cassar, Umnai: "Hybrid Intelligence – Machine Enhanced Decision Making beyond the current frontier"(PDF 1,1 MB)
- Marc Wildi / Branka Hadji Misheva, ZHAW/BFH: "A Time Series Approach to Explainability for Neural Nets with Applications to Risk-Management and Fraud Detection"(PDF 1,0 MB)
Ethical Questions in Artificial Intelligence
- Ricardo Chavarriaga, ZHAW
- Nicole Büttner, Merantix
- Eleonora Viganò, UZH
- Jérôme Koller, Mobiliar
- Andreas Blum, Unit8: "How to ensure "Trustworthy AI" from a governance perspective and get ready for the planned EU AI Act, also affecting Swiss entities"(PDF 3,9 MB)
Best practice / Use cases
- Patricia Deflorin, FHGR
- Hanspeter Bär, Innosuisse: "How to be successful with your Innosuisse Application for innovation project funding"(PDF 1,2 MB)
- Manuel Eicher, Geobrugg
- Amin Amini / Arman Iranfar, CertX
Closing panel: How to do interdisciplinary, data-driven research
- Peter Schwendner, ZHAW (Moderation)
- Jennifer Scurrell, ETH
- Pius von Däniken, ZHAW
Schedule
- Welcome: 09:45-10:00
- Keynote: 10:00-10:45
- Coffee Break: 10:45-11:15
- Thematic Sessions Part 1: 11:15-12:45. AI in Finance, AI in Industry, Ethical Questions, Best practice/use cases
- Lunch Break: 12:45-14:00
- Thematic Sessions Part 2: 14:00-15:00. AI in Finance, AI in Industry, Ethical Questions, Best practice/use cases
- Coffee Break: 15:00-15:30
- Thematic Sessions Part 3: 15:30-16:30. AI in Finance, AI in Industry, Ethical Questions, Best practice/use cases
- Closing panel: 16:45-17:15
- Apero: 17:15
Detailed Schedule
Keynote (TN E0.46-54) | |||
09:45-10:00 | D. Wilhelm: "Welcome and Introduction" | ||
10:00-10:45 | P. Mattson: "MLCommons: driving ML innovation with benchmarks" | ||
10:45-11:15 | Coffee (Foyer TN) | ||
AI in Industry 1 (TN E0.46) | AI in Finance 1 (TN E0.54) | Ethics in AI 1 (TN E0.58) | |
11:15-11:45 | M. Keller, Acodis: "Structuring Today’s Data to Save Tomorrow’s Lives" | F. Woebbeking, Axiomatec/Univ. Frankfurt: “Let me get back to you" - A machine learning approach to measuring non-answers" | R. Chavarriaga, ZHAW: "Operationalizing AI ethics: Challenges for organizational governance |
11:45-12:15 | E. Sirazitdinova, NVIDIA: "Synthetic Data and Simulation Workflows for AI Training in Robotics | B. Horvath, TU München: "Machine Learning - Market Simulation Generating Financial Markets with Signatures" | N. Büttner: "AI and Mafia" |
12:15-12:45 | A. Geller, Scensei: "Decision Support, AI and good practices" | D. Boos/L. Grob, Avena Capital/ZHAW: "Tracking Speculative Trading" | E. Viganò, UZH: "Ethics of algorithmic prediction: How algorithms threaten our right to be unpredictable" |
12:45-14:00 | Lunch (Foyer TN) | ||
AI in Industry 2 (TN E0.46) | AI in Finance 2 (TN E0.54) | Ethics in AI 2 (TN E0.58) | |
14:00-14:30 | M. Perani, FFHS: "Deep neural networks for predicting track geometry in additive manufacturing" | T. Krabichler, FH Ost: "Deep Asset-Liability-Management" | J. Koller, Mobiliar: "You have your ethical framework, so what?" |
14:30-15:00 | G. Fischetti, UniVe: "Automatic Classification of Multiplets in 1H NMR Spectra with Deep Learning" | M. Niggli/C. Rutzer, Univ. Basel: "What is it worth? Predicting the Importance of Patents Using Supervised Learning" | A. Blum, Unit8: "How to ensure "Trustworthy AI" from a governance perspective and get ready for the planned EU AI Act, also affecting Swiss entities" |
15:00-15:30 | Coffee (Foyer TN) | ||
AI in Industry 3 (TN E0.46) | AI in Finance 3 (TN E0.54) | Academia-Industry (TN E0.58) | |
15:30-16:00 | S. Welter, IKEA: "The life of an innovation manager in IKEA" | K. Cassar, Umnai: "Hybrid Intelligence – Machine Enhanced Decision Making beyond the current frontier" | P. Deflorin, FHGR: "Databooster – Open innovation activities to boost data based innovation"; H. Bär, Innosuisse: "How to be successful with your Innosuisse Application for innovation project funding" |
16:00-16:30 | N. Pascher/T. Stadelmann, Mettler Toledo/ZHAW: "Data-centric transfer learning as a solution to key-challenges in industrial data-based solutions" | M. Wildi/B. Hadji Misheva, ZHAW/BFH: "A Time Series Approach to Explainability for Neural Nets with Applications to Risk-Management and Fraud Detection" | M. Eicher, Geobrugg: "Smart protection systems - where do we go from here?"; A. Amini/A. Iranfar, CertX: "certAInty - A Certification Scheme for AI systems" |
16:45-17:15 | Closing panel (TN E0.46-54) | ||
17:15-18:00 | Apéro riche (Foyer TN) | ||
Registration
Registration is now open.
Dr. Peter Mattson
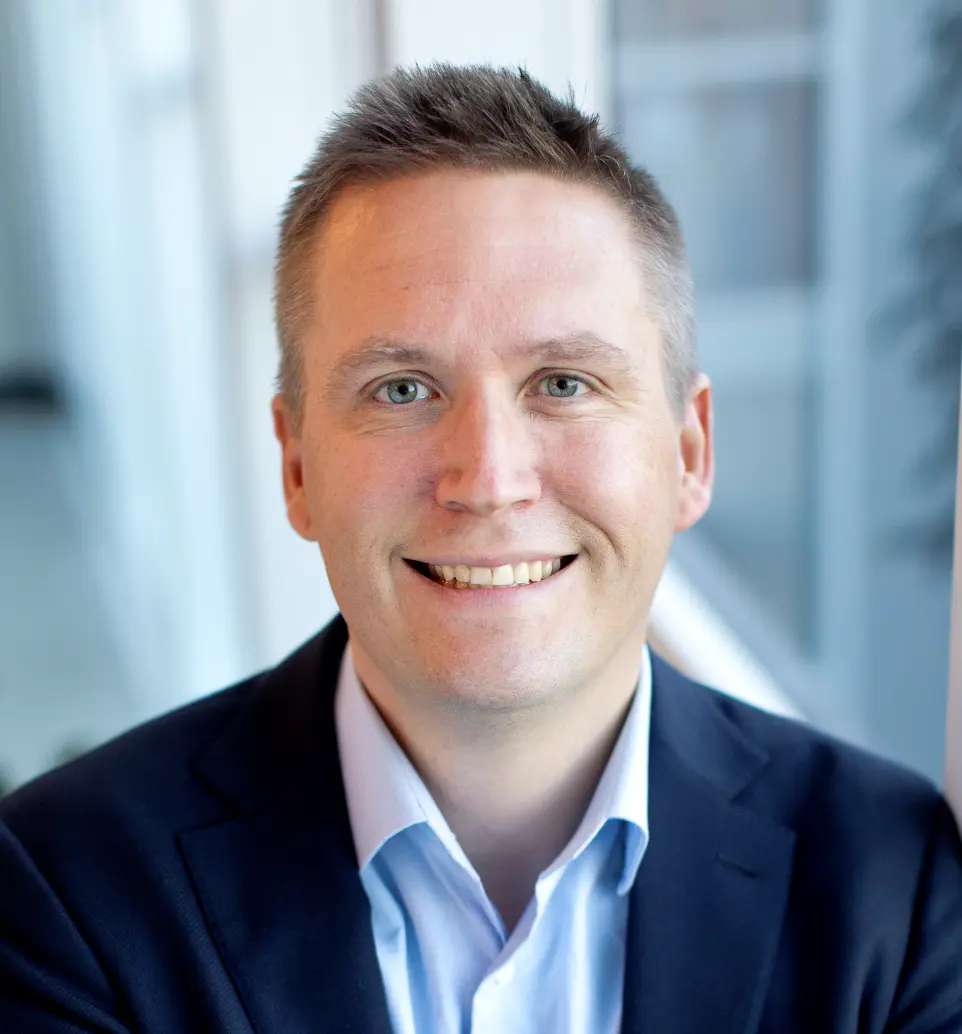
A Brief Biography
Peter Mattson is a Google senior staff engineer. He co-founded and is President of MLCommons, and co-founded and was General Chair of the MLPerf consortium that preceded it. Previously, he founded the Programming Systems and Applications Group at NVIDIA Research, was VP of software infrastructure for Stream Processors Inc (SPI), and was a managing engineer at Reservoir Labs. His research focuses on understanding machine learning models and data through quantitative metrics and analysis. Peter holds a PhD and MS from Stanford University and a BS from the University of Washington.
MLCommons: driving ML innovation with benchmarks
MLCommon.org is a non-profit with a mission to make ML better for everyone by building the ML ecosystem, principally benchmarks, datasets, and best practices. The talk will describe several benchmarks with applicability to finance, including the MLPerf speed benchmark, the DataPerf data quality benchmarks, and the MedPerf benchmark approach to federated evaluation. It will also speculate on the potential for benchmarks in the finance industry.
Martin Keller
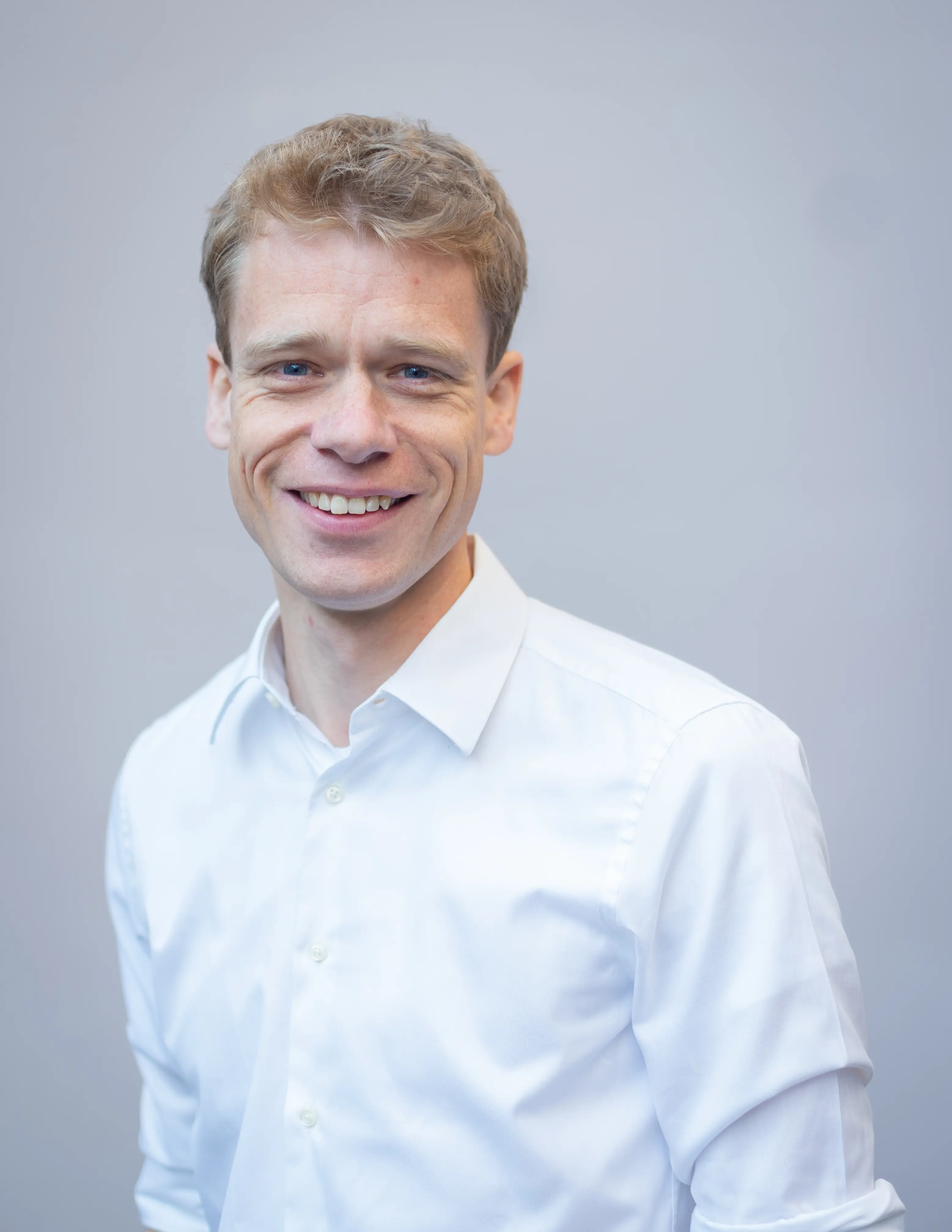
A Brief Biography
Martin Keller is co-founder and CEO of Acodis where he has built a tech startup that is pioneering the field of intelligent document processing. Prior to Acodis, Martin worked in finance and management consulting. He studied International Relations at The Graduate Institute in Geneva and completed his MBA at IESE Business School in Barcelona.
Structuring Today’s Data to Save Tomorrow’s Lives
80% of the world’s data that could be used to make critical decisions is unstructured data.
In health, this information is typically locked in documents like patient data, studies, leaflets, and health regulations. The issue is that these documents are hard to analyse and process in large amounts because they are neither machine-readable nor ready for downstream consumption.
Breakthroughs in AI are now available to extract and structure the correct information at scale. To provide NLP and data teams with the crucial context (including headers, tables, pictures, and handwriting) for accurate data analytics.
Join this talk to learn how to leverage your unstructured data, discover new insights, and save lives with AI.
Dr. Ekaterina Sirazitdinova
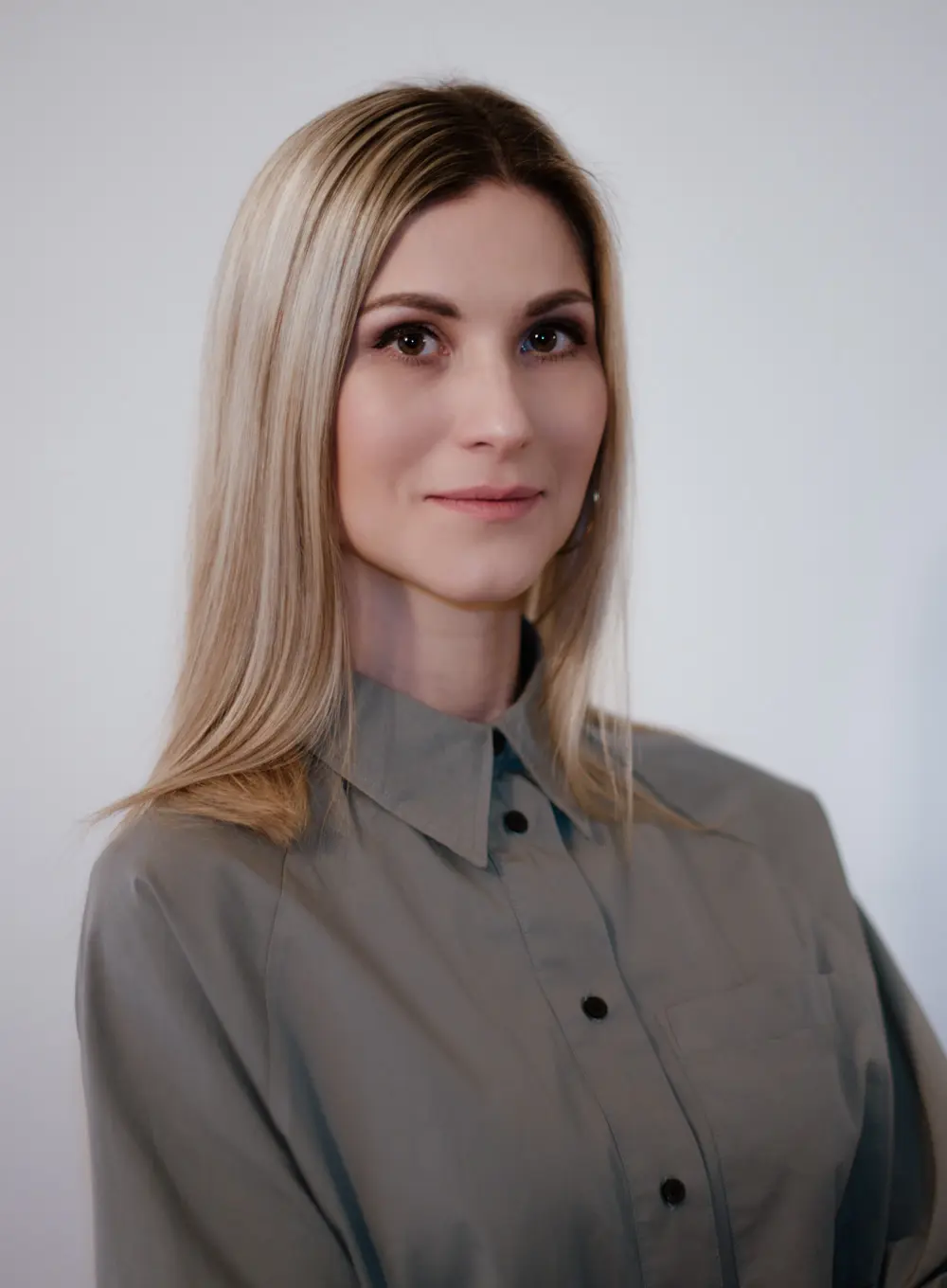
A Brief Biography
Ekaterina is a senior data scientist at NVIDIA specialized in solving computer vision and video analytics problems by means of AI. Her current focus also includes deep learning inference optimization on embedded devices. Previously, Ekaterina was a research engineer applying deep learning to medical image analysis. She has also authored several peer-reviewed journal and conference publications on various applications of image-based 3D reconstruction, localization and tracking. Ekaterina received her Ph.D. in Computer Science and M.Sc in Media Informatics, she also holds a Diploma in Business Informatics.
Synthetic Data and Simulation Workflows for AI Training in Robotics
Developing robots capable of perceiving complex environments in a scalable way is not a trivial task. Relying on AI, robots become more intelligent, flexible, and robust, but AI training requires lots of data. Data collection and annotation are time consuming and expensive. Furthermore, in order to create models which generalize well, data needs to be diverse and balanced. With advancement in simulation tools and generative models, more and more AI practitioners start utilizing synthetic data as a possible alternative to real data. In this session, we will focus on NVIDIA’s approach to synthetic data generation and robotics simulation and will showcase the underlying value of our tools for realistic and accurate data creation.
Dr. Armando Geller
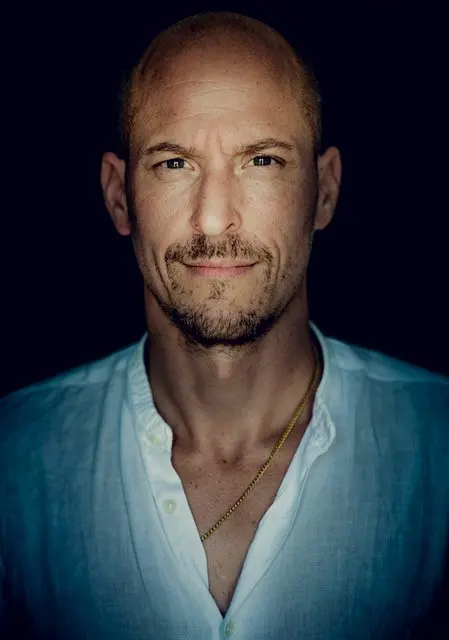
A Brief Biography
Armando Geller is Managing Partner at Scensei (Switzerland) GmbH, a boutique enterprise specializing in AI-driven constructive simulation. Armando holds a doctor of philosophy from the University of Zurich, did his postdoctoral degree at the Centre for Policy Modelling at Manchester Metropolitan University, and worked as a research assistant professor at the Department for Computational Social Science at George Mason University.
Decision Support, AI and good practices
Supporting decision makers in making the right decisions using computer simulations goes back decades and the literature on related challenges is abundant. As of today, when AI is thrown into the mix the good practices from the old times hold true by and large. Yet, we’ve been confronted with new challenges that arguably bear their own qualities when employing AI in decision support via computer simulation. Discussing these challenges and the best practices that address these challenges in context is important to establish practical relevance. What does, for example, “Can I explain the outcome of my simulation?” mean applied to a real world case? What is the actual implication of the answer to “Is the output of my simulation ethical?” During my talk I will invite you to play a short serious game to co-explore both these new challenges and the good practices to address them.
Dr. Martina Perani
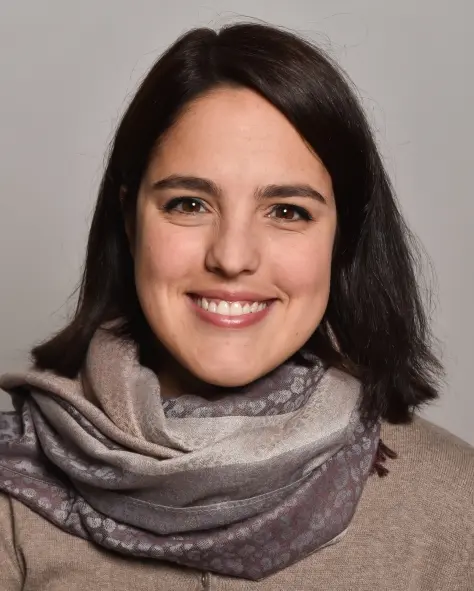
A Brief Biography
Dr. Martina Perani holds a PhD degree in Physics from the University of Bologna, where she focused on the optoelectronic and morphological characterization of semiconductors for photovoltaic applications. She then moved to the Fernfachhochschule Schweiz (FFHS), where she further developed her competences in the field of data science and artificial intelligence. Currently, she leads the research field “Data Science for Energy, Environment and Materials” at the FFHS. Her research interests lie in the application of artificial intelligence to address research questions in these technical disciplines, with projects in the areas of additive manufacturing, photovoltaics and warning systems.
Deep neural networks for predicting track geometry in additive manufacturing
Laser Metal Deposition (LMD) is an additive manufacturing technology that attracts great interest from the industry, thanks to its potential to realize parts with complex geometries in one piece, and to repair damaged ones, while maintaining good mechanical properties. The complexity of the LMD process has however limited its widespread adoption so far, as different part geometries, strategies and boundary conditions can yield very different results in terms of external shapes and inner flaws. Deep learning based architectures have been used to estimate the size of tracks deposited by LMD using powders of Inconel 718, a relevant material for the aerospace and automotive sectors. An architecture that combines the output of a convolutional neural network (that takes melt pool images as inputs) with scalar variables (process and trajectory data) is used to predict the track geometry. Moreover, preliminary results suggest that long-short memory networks are effective in modeling the temporal evolution of the deposition process using process and trajectory data.
Giulia Fischetti
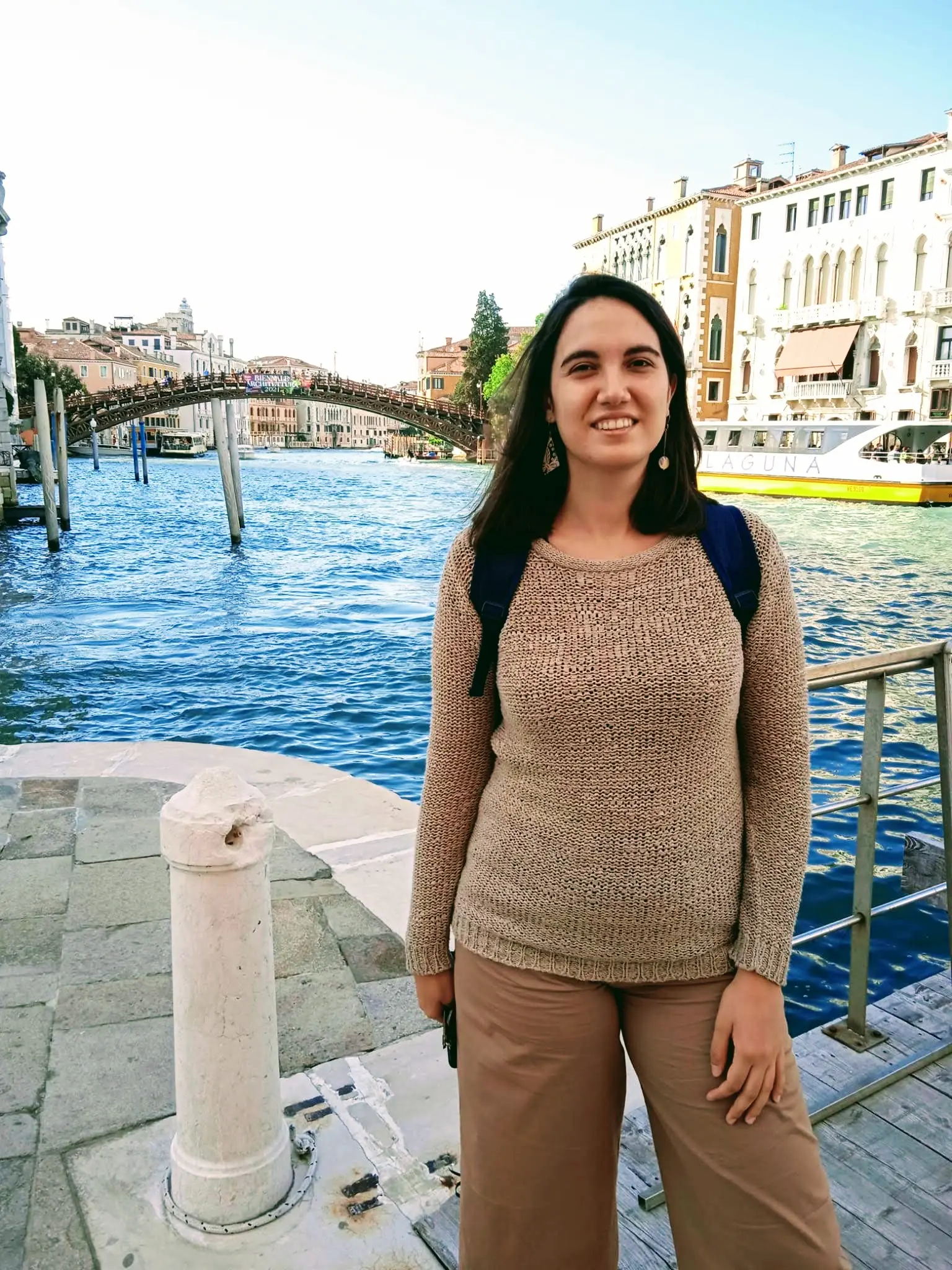
A Brief Biography
Giulia Fischetti obtained her B.Sc. (2017) and M.Sc. (2020) in Physics from the University La Sapienza of Rome. During her academic studies, she focused on the statistical physics of complex and disordered systems. For her Master’s thesis, she investigated the dynamics and storage properties of models for associative memory, such as the Hopfield model. She continued working on the statistical properties of neural networks thanks to a post-lauream fellowship (2021) at the University La Sapienza of Rome while cultivating her arising interest in artificial intelligence and deep learning algorithms.
Currently, she is pursuing a PhD in Science and Technology of Bio and Nanomaterials from the University Ca' Foscari of Venice. For her PhD project, she is collaborating with the ZHAW School of Engineering and the Bruker BioSpin Group to implement machine learning techniques to perform automatic analysis of Nuclear Magnetic Resonance (NMR) data.
Automatic Classification of Multiplets in 1H NMR Spectra with Deep Learning
The identification and classification of signal peaks (multiplets) in Nuclear Magnetic Resonance (NMR) spectra is a decisive stage in the characterization of complex chemical compounds. Unfortunately, the process of retrieving information from the spectra is often burdensome, time-consuming and susceptible to errors. Moreover, it requires the involvement of experienced users. Therefore, introducing automation into the NMR analysis workflow holds the promise of speeding up the process while increasing the robustness and reproducibility of the results.
We will present how a supervised deep learning algorithm can perform automatic detection and classification of multiplets in 1H NMR spectra, the most straightforward kind of NMR experiments. The training set is composed of a large number of synthetic spectra designed with complete control over the features represented in the samples. The output is a point-by-point prediction of a label value that corresponds to a given multiplet category.
Furthermore, we will discuss how implementing an ensemble learning framework can help distinguish overlapping multiplets from non-overlapping ones.
The precise location of signal regions can guide the integration of peaks’ profiles, while the correct classification gives information on the number of peaks within signal regions, facilitating peak picking and coupling constants extraction.
We will show that our model can discriminate signal regions effectively and minimize classification errors between different types of multiplets. Most importantly, we will demonstrate that the network generalizes remarkably well on real experimental 1H NMR spectra.
Sebastian Welter
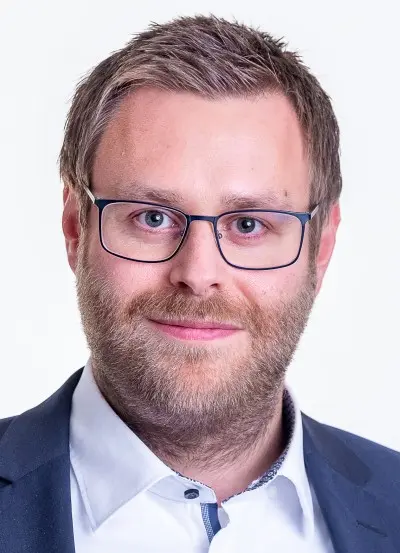
A Brief Biography
Sebastian Welter is leading AI innovation at IKEA. His background is classical IT, being an IT architect by profession. His first steps in AI were taken at IBM, when he was responsible as architect in Germany for the AI that started the hype in 2011 - IBM’s Watson. From there he went into consulting, spending some years in defining AI strategies and solutioning analytics/cloud/data/AI projects as a director at Accenture. Now his focus is technology driven innovation, whether its AI or any other fascinating digital technology.
Innovate a retailer with AI: The life of an innovation manager in IKEA
Everyone “does AI” nowadays, and the larger the company, the bigger the fails and maybe the achievements are. But how do you do it? Follow me on a typical day in innovation in IKEA on our journey to create a better everyday life for the many people. The talk will touch on how AI and innovation can change a company, what challenges to overcome and how to make it all work in grand scale.
Dr. Nikola Pascher, Dr. Thilo Stadelmann
A Brief Biography
Dr. Nikola Pascher is the global head of Mettler Toledo's R&D team in the strategic business unit standard industry. She is responsible for the product portfolio, consisting of weighing solutions for industrial applications, controllers and software, covering the entire technology-stack from the sensor to the cloud. She studied physics at the University of Augsburg in Germany. Her phd at ETH Zurich, Switzerland and a consecutive postdoctoral assignment at IBM Research, Zurich were focused on quantum science. After leaving academia, she worked in various positions in technology and innovation management with a focus on high-precision measurement instrumentation and data science for digital solutions and services. She extended her knowledge with an Executive MBA from the University of St. Gallen. Her main interest is to create value with breakthrough technologies.
Dr. Thilo Stadelmann is professor of artificial intelligence and machine learning at the ZHAW School of Engineering in Winterthur, director of the ZHAW Centre for Artificial Intelligence and head of its Computer Vision, Perception and Cognition Group. A computer scientist by training, he received his doctor of science degree from Marburg University in 2010, where he worked on multimedia analysis and voice recognition. Thilo worked in engineering and leadership roles in the automotive industry for 3 years prior to switching back to academia. His current research focuses on robust deep learning to solve diverse pattern recognition tasks such as biometrics, document analysis or computer vision for industrial and medical applications. He is fellow of the European Centre for Living Technology in Venice, Italy, co-founder and board member of Switzerland’s largest innovation network, the data innovation alliance, and of the ZHAW Datalab, one of Europe’s first dedicated research hubs for data science. His book "Applied Data Science - Lessons Learned for the Data-driven Business" was published 2019 by Springer, a pre-print on "A Theory of Natural Intelligence" that informs a future roadmap for machine learning research was released recently.
Data-centric transfer learning as a solution to key-challenges in industrial data-based solutions
To date, most of the applications of machine learning in industrial companies have an inward focus. Methods form data science are used to simplify company processes, place efficient marketing, automatic digitalization of documents and many more. The industrial internet of things instead, is focused outwards, towards the customers with the goal to create true value from their data, which is obtained, e.g., from running industrial applications. IIoT pictures a beautiful perspective, yet many companies struggle to offer data-based solutions, which are based on measured sensor-data from industrial processes. Digital innovators meet a variety of obstacles, which prevent them from efficiently developing solutions which turn data from the customer’s production sites into value. Taking Mettler Toledo’s industry division as an example, we picture the pathway of a very traditional industry company extending their technology stack to the top.
A general problem in industrial applications of machine learning is that (labeled) training data is rarely available. Either the creation of labeled ground-truth data sets is too expensive, or the data belongs to the customers, who might be worried to share their data with a solution provider. On top of that, machine learning models do not generalize efficiently. Model performance is poor, if the pre-trained model is applied to different data sets it was not trained for initially. This means a big pain to the solution provider and to the customer, as extensive model training would have to be included in the customer workflow.
We focus on a data-centric approach in combination with transfer learning as a possible solution scenario for the above-mentioned challenge. We show an example from medical imaging, where we improve the performance of automated image recognition of the spine with an unsupervised domain adaptation (UDA) approach for vertebrae detection and identification based on a novel Domain Sanity Loss (DSL) function.
This example can be abstracted to a variety of different processes and could be a valid solution to facilitate the use of machine learning in industrial processes.
Dr. Fabian Woebbeking
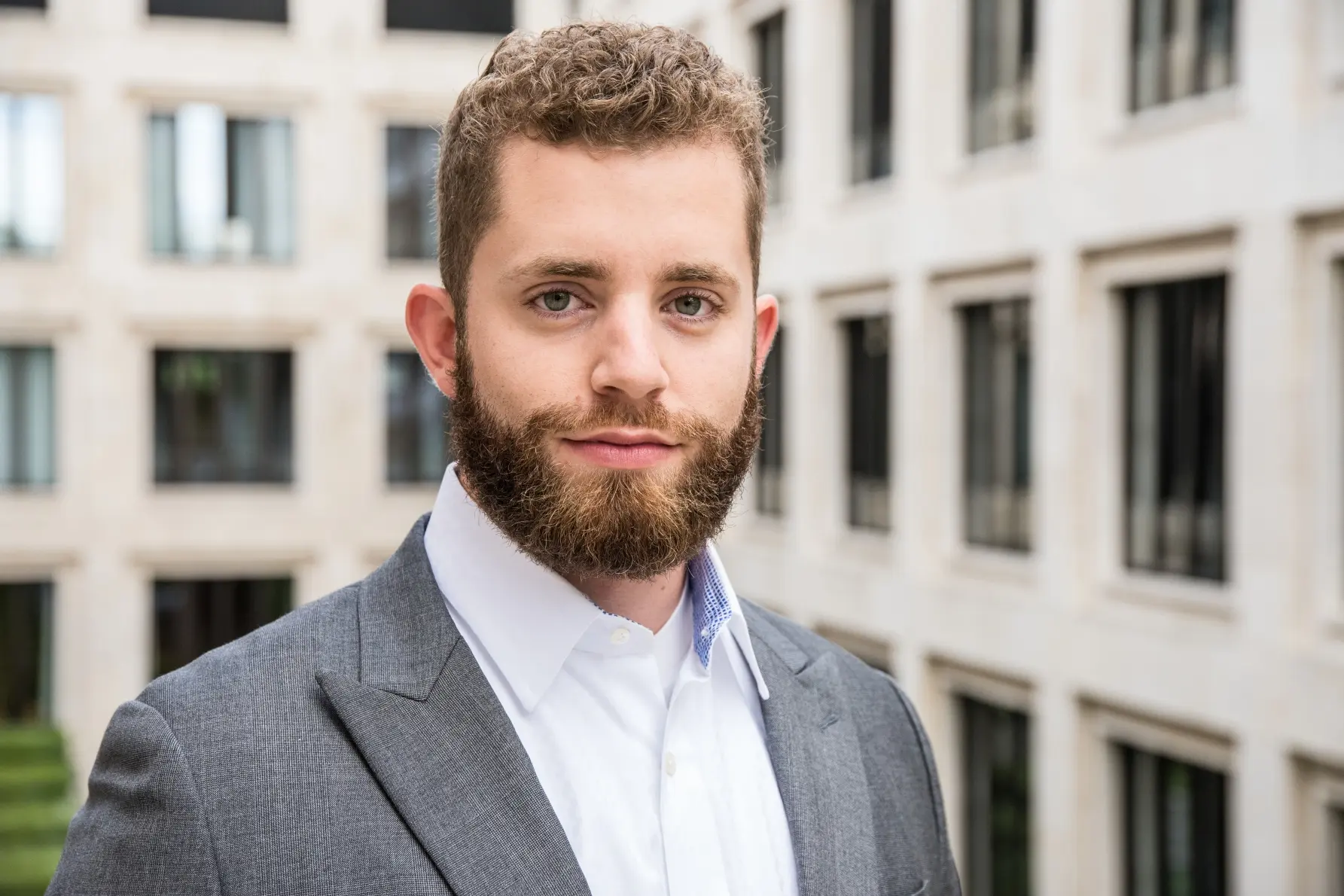
A Brief Biography
As a financial economist and data scientist I focus on computational finance, financial risk management, impact investing, natural language processing, extreme value theory, econlinguistics and systemic risk. As a lecturer at Frankfurt School of Finance & Management, I teach courses in financial economics and derivative pricing. I am a co-founder of two companies, namely Axiomatec AG, a fin-tech company that develops quantitative investment and risk management solutions for institutional clients. As well as Hiveanalytica UG that focuses on business analytics.
"Let me get back to you" - A machine learning approach to measuring non-answers
Using a supervised machine learning framework on a large training set of questions and answers, we identify 1,364 trigrams that signal non-answers in earnings call Q&A. We show that this glossary has economic relevance by applying it to contemporaneous stock market reactions after earnings calls. Our findings suggest that obstructing the flow of information leads to significantly lower cumulative abnormal stock returns and higher implied volatility. As both our method and glossary are free of financial context, we believe that the measure is applicable to other fields with a Q&A setup outside the contextual domain of financial earnings conference calls.
Prof. Blanka Horvath
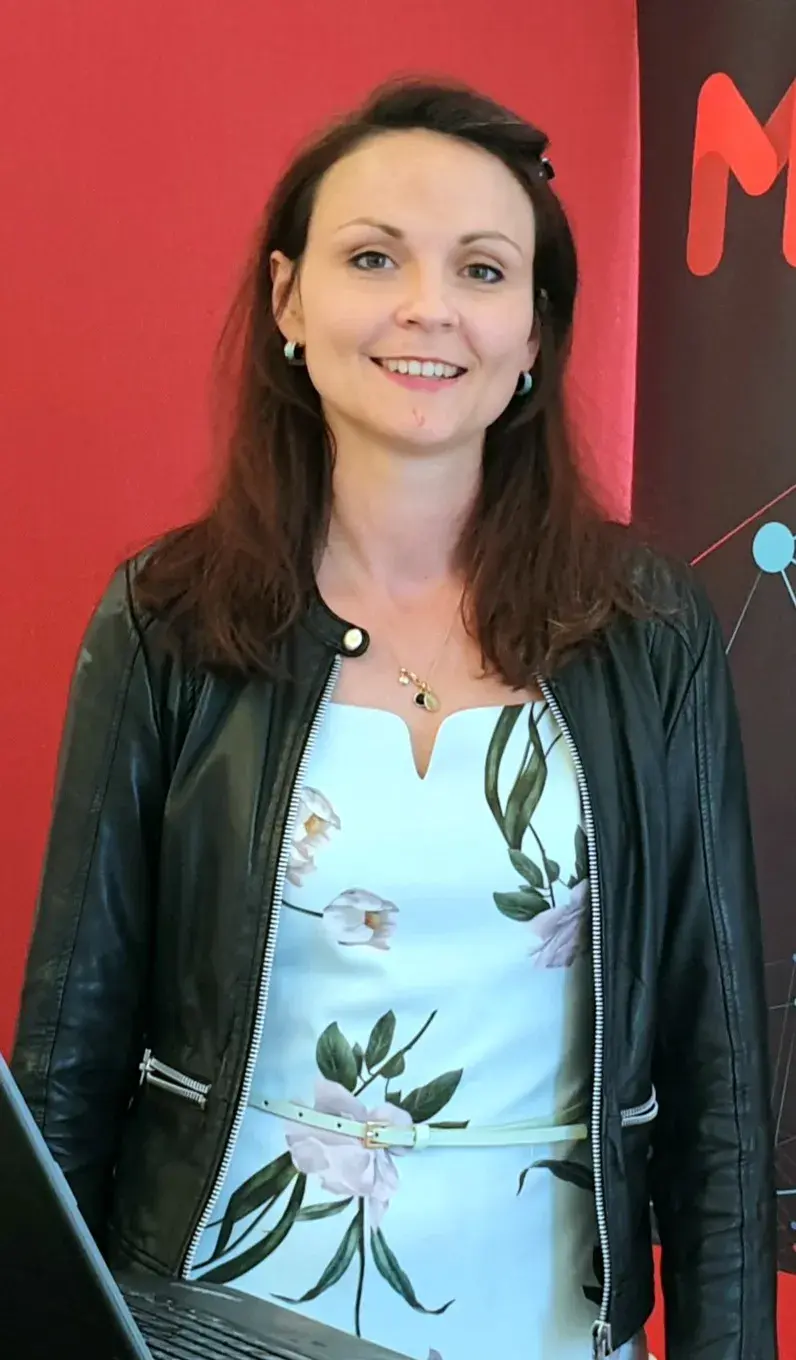
A Brief Biography
Professor Dr. Blanka Horvath's current research interests focus on asymptotic and numerical properties of stochastic volatility models, in particular models with rough paths (rough volatility), as well as using generative methods -across models- to generate synthetic financial market data. For her contributions to the calibration of (rough) stochastic volatility models with deep neural networks, she received the Rising Star Award from Risk Magazine in 2020. Blanka Horvath was appointed Professor at the Chair of Financial Mathematics at the Technical University of Munich in April 2021.
Machine Learning - Market Simulation Generating Financial Markets with Signatures
Market Simulation and Deep Hedging: Neural network based data-driven market simulation unveils a new and flexible way of modelling financial time series, which has recently inspired a surge of research activity in the quantitative finance community. While most generative models tend to rely on large amounts of training data, we present here a generative model that works reliably even in environments where the amount of available training data is small, irregularly paced or oscillatory. We show how a rough paths-based feature map encoded by the signature of the path outperforms returns-based market generation both numerically and from a theoretical point of view. Finally, we also propose a suitable performance evaluation metric for financial time series and discuss some connections of our signature-based Market Generator to deep hedging.
Linus Grob, Dr. Dominic Boos
A Brief Biography
Linus Grob is a research associate at the Institute for Wealth and Asset Management at the ZHAW School of Management and Law and a business analyst at the Bank Vontobel AG. He graduated in Banking and Finance at the ZHAW School of Management and Law and is now pursuing his Ph.D. in Banking and Finance at the University College Dublin. His research focuses on machine learning applications in capital markets and the application of alternative data in Finance.
Dominik Boos is a lecturer at the Institute for Wealth and Asset Management at the ZHAW School of Management and Law. His teaching focuses on active and quantitative investment strategies as well as on sustainable investing. Dominik has more than twenty years of industry expertise in strategy research and active investment management. He is also an investment advisor for Serafin Asset Management and founded Avena Capital in 2017. Dominik worked as Head of Global Macro Research at SSGA where he was a member of the global macro investment committee and directed the development and the implementation of the relative value book. Before, Dominik worked as a Senior Investment Strategist at the Swiss National Bank, responsible for the strategic asset allocation of the foreign reserves. Previously, he was Head of Quant Research at Zurich Cantonal Bank. Between 2004 and 2009, Dominik worked at Kraus Partner, a Zurich-based asset management company. As Partner and Head of Investment Management he was responsible for research, portfolio management and trading.
Dominik has earned a PhD in Finance and Economics from the University of St. Gallen and a Masters in Mathematics from the Swiss Federal Institute of Technology.
Tracking Speculative Trading
Managed futures funds are predominantly trend-followers. By analyzing positioning data, we provide novel evidence for this claim and estimate signals applied by these funds. We write trend-followers aggregate position as a weighted sum of past daily returns and use a generalized ridge regression for regularization and parameter estimation. This procedure prevents overfitting but remains flexible enough to capture various patterns. For the 23 commodities considered, trend-following can explain speculators' position changes with an average $R^2$ of more than 40 percent. Finally, we document that producers act as contrarians in a way that closely mirrors the behavior of momentum traders.
Dr. Thomas Krabichler
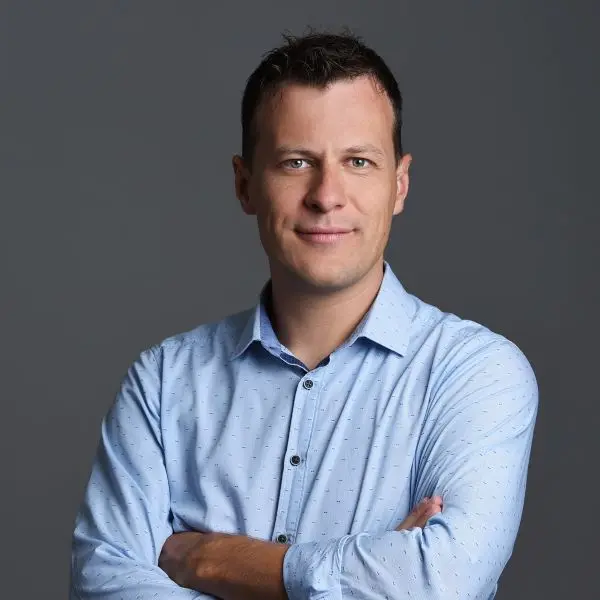
A Brief Biography
Thomas Krabichler is a lecturer and quant researcher at the OST – Eastern Switzerland University of Applied Sciences. He holds a doctoral degree from ETH Zürich in mathematics. His research focuses on applications of machine learning in finance. Previously, he worked as a quant specialist for the pricing and hedging of financial derivatives. In this role, he provided technical model validations of valuation models for major investment banks in London and Paris.
Deep Asset-Liability-Management
Recent advances inspired by reinforcement learning support well-balanced risk-taking and unprecedented risk-adjusted pricing. In ongoing initiatives with several colleagues and partners, we are utilising these techniques in order to tackle high-dimensional optimisation problems in the presence of constraints and frictions. The range of applications involves optimal decision making for treasurers, optimal procurement of commodities, or the optimisation of hydroelectric power plants.
Dr. Matthias Niggli, Dr. Christian Rutzer
A Brief Biography
Matthias Niggli is a research data scientist and project manager at the Center for International Economics and Business | CIEB of the University of Basel. He holds a PhD from the University of Basel, has a special interest in experimenting with all kinds of machine-learning methods and is enthusiastic about discovering novel approaches to visualize data.
Christian Rutzer is deputy director and project manager at the Center for International Economics and Business | CIEB of the University of Basel. He holds a PhD in economics from the University of Basel and likes to apply new methods from data science to analyze economic issues.
What is it worth? Predicting the Importance of Patents Using Supervised Learning
We demonstrate that a combination of deliberate feature engineering and deep learning methods can be used to predict the technological importance of recently filed patents. This provides a scalable framework for early assessments of companies’ current innovation pipelines.
Dr. Ken Cassar
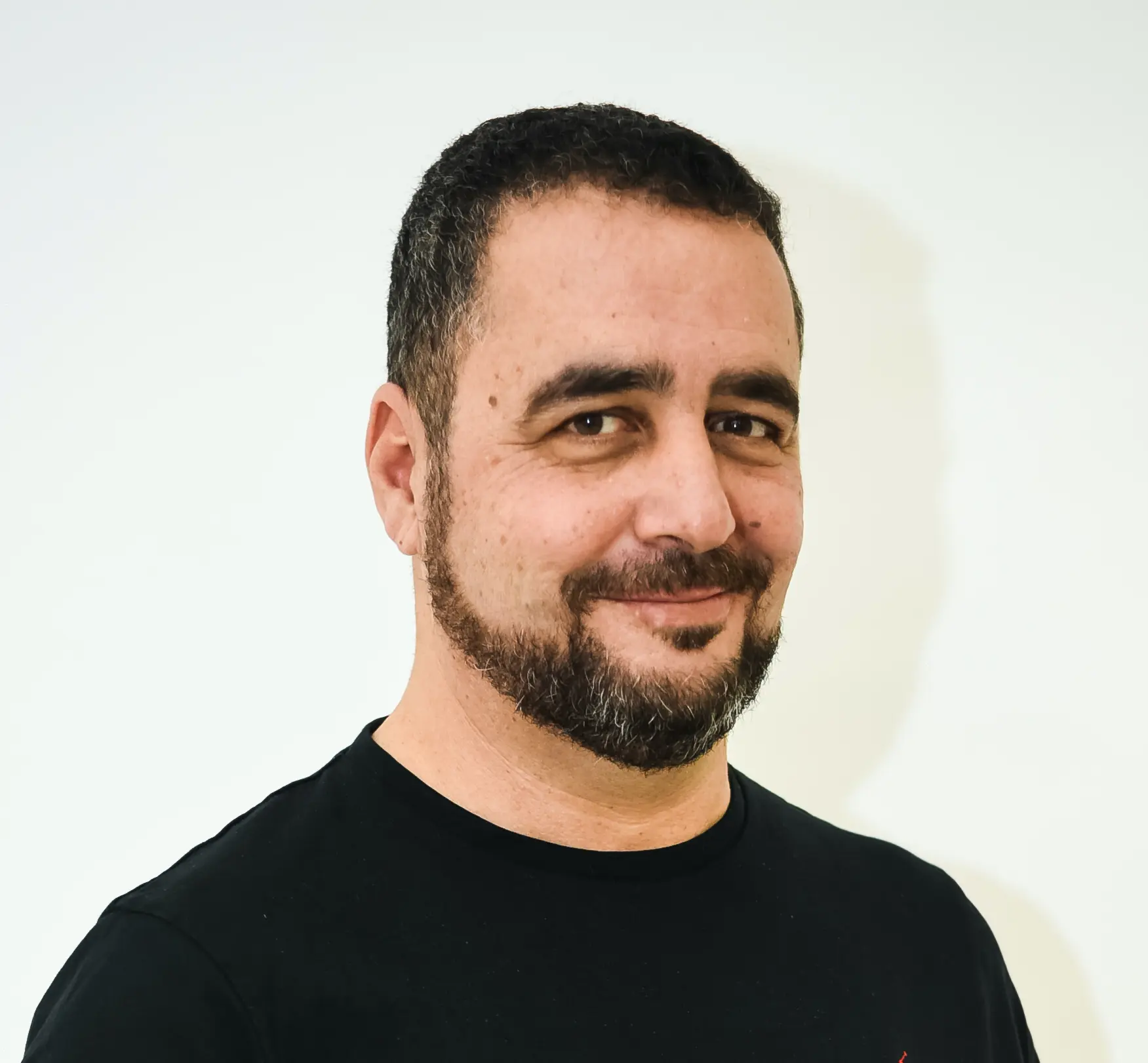
A Brief Biography
Ken is a co-founder and co-CEO of Umnai where he has been part of a team that has invented and productised a ground breaking technology since 2019. Prior to UMNAI Ken founded and ran two enterprises in the Fintech space, building a cutting edge physical/online POS infrastructure and later as a payment service provider in online card processing in Europe and North America.
Hybrid Intelligence – Machine Enhanced Decision Making beyond the current frontier
Recent advances in Neuro-Symbolic AI bring transformative changes to how we can conceive, design, build and consume data informed automated decision systems. This talk will introduce Hybrid intelligence and look at how it empowers organisations to resolve the problems of bias, risk, ethics, fairness and human oversight.
Prof. Marc Wildi / Prof. Branka Hadji Misheva
A Brief Biography
Prof. Dr. Marc Wildi is Professor of Econometrics at the Zurich University of Applied Sciences. His research interests are about forecasting, real-time signal extraction, business-cycle analysis, algorithmic trading and risk management. His recent work emphasizes hybrid approaches (mixing real-time filter designs and generic trading concepts) as well as explainability (XAI) of computationally intensive approaches (NN) in the context of longitudinal data (time series).
Prof. Dr. Branka Hadji Misheva is a Professor in Applied Data Science and Finance at BFH, working on AI applications in finance, XAI methods, network models and fintech risk management. She holds a PhD in Economics and Management of Technology with a specific focus on network models as they apply to the operation and performance of P2P lending platforms, from the University of Pavia, Italy. In the past, she has led several research and innovation projects on Artificial Intelligence and Machine Learning for Credit Risk Management (Innosuisse, EU Horizon 2020, COST Action). She is a research author of over 17 papers in the field of credit risk modeling, graph theory, predictive performance of scoring models, lead behavior in crypto markets and explainable AI models for credit risk management.
A Time Series Approach to Explainability for Neural Nets with Applications to Risk-Management and Fraud Detection
Artificial intelligence (AI) is creating one of the biggest revolution across technology-driven application fields. For the finance sector, it offers many opportunities for significant market innovation and yet broad adoption of AI systems heavily relies on our trust in their outputs. Trust in technology is enabled by understanding the rationale behind the predictions made. To this end, the concept of eXplainable AI (XAI) emerged introducing a suite of techniques attempting to explain to users how complex models arrived at a certain decision. For cross-sectional data classical XAI approaches can lead to valuable insights about the models' inner workings, but these techniques generally cannot cope well with longitudinal data (time series) in the presence of dependence structure or non-stationarity. In this talk I will present common work with Branka Hadji about a novel XAI-technique for deep learning methods (DL) which preserves and exploits the natural time ordering of the data. After a brief introduction to the main concepts, simple applications to financial data illustrate the potential of the approach in the context of risk-management and fraud-detection.
Dr. Ricardo Chavarriaga
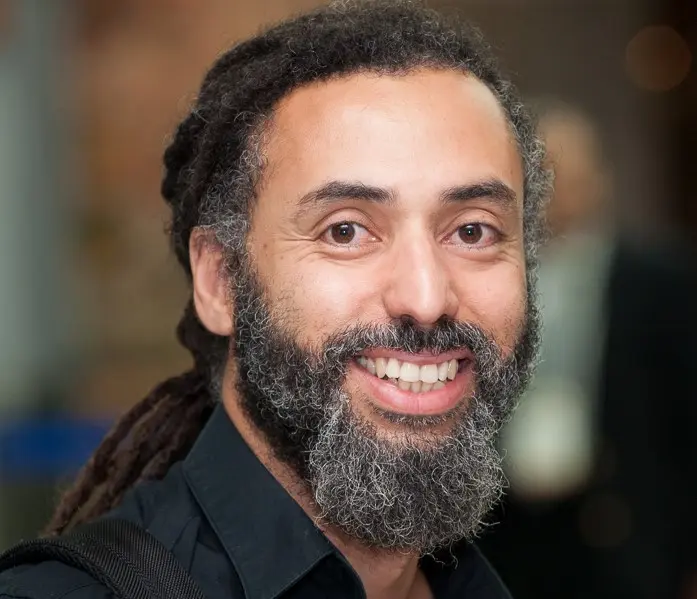
A Brief Biography
Ricardo Chavarriaga, PhD, is a neuroscientist passionate about responsible development and societal implications of technology. His work is focused on fostering research and innovation in emerging technologies towards the benefit of society.
Dr Chavarriaga is senior researcher at the Zürich University of Applied Sciences (ZHAW) and Polymath fellow at the Geneva Center for Security Policy (GCSP). He is the head of the Swiss office of the Confederation of Laboratories for AI Research in Europe (CLAIRE). CLAIRE is the largest European network on Artificial Intelligence (AI), comprising more than 400 research groups and 3’000 individual supporters. He chair the principles sub-group of IEEE P2863 Working group on Recommended Practices for Organisational Governance of AI.
Operationalizing AI ethics: Challenges for organizational governance
Upcoming regulatory frameworks for Artificial Intelligence (AI) systems will require organizations to certify that the systems they develop comply with requirements based on ethical principles such as transparency, fairness reliability, and safety. Making the bridge between these ethical principles and organizational processes is not an easy feat. This talk will discuss this challenge and present current examples of initiatives aimed at tackling it.
Nicole Büttner
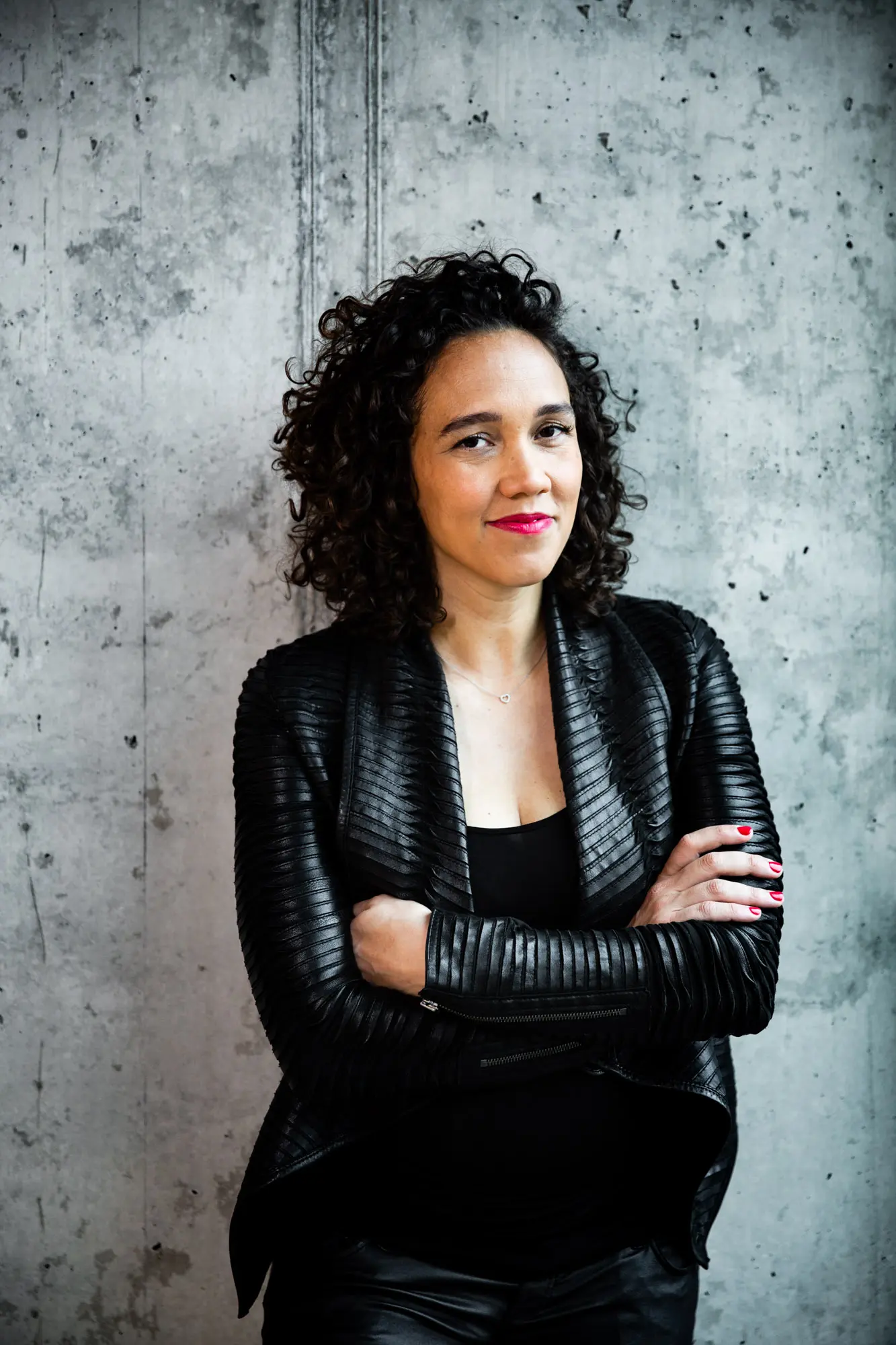
A Brief Biography
Nicole Büttner is a tech optimist at heart and is active as an entrepreneur, investor and board member. Her passion is to create real impact with new technologies useful to companies and people. Nicole is co-founder and CEO of MerantixMomentum, a leading AI solutions firm and member of the management board of Merantix, an AI venture studio based in Berlin.
Nicole is Digital Leader at the World Economic Forum and was nominated as a Young Leader by the Aspen Institute. She was selected twice as 40 under 40 by Capital Magazine and Rising Talent by the Women’s Forum.
Previously, she worked as a hedge fund portfolio manager at OFI AM in Paris and led the global business development for Autionomics, Nobel Prize winner Paul Milgrom’s tech and consulting firm. She serves on the Board of Alumni of the University of St. Gallen and on the board of the German Startup Association.
She regularly lectures at the University of St. Gallen and other academic institutions. Nicole trained as an economist and econometrician at the University of St.Gallen, Stockholm School of Economics and Stanford University and holds a MA in Quantitative Economics and Finance.
AI and Mafia
The challenges of our times are becoming more complex and innovating with AI is different than with classic software tools. What can we learn from the mafia for building successful AI solutions?
Dr. Eleonora Viganò
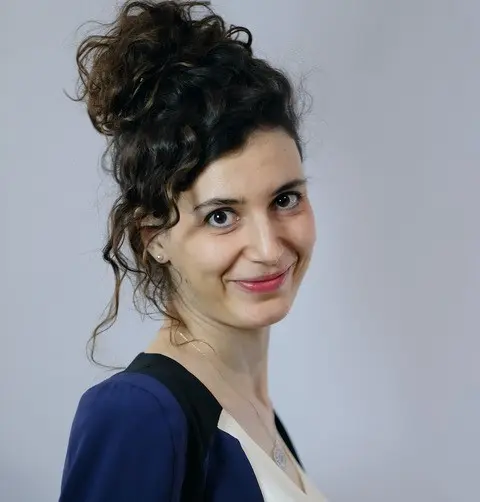
A Brief Biography
Eleonora Viganò is Postdoctoral Researcher at the Institute of Biomedical Ethics and History of Medicine, at the University of Zurich. She was recently the Executive Manager of the ELSI-Task-Force for the Swiss National Research Programme 75 “Big Data” and developed the moral aspects of the Swiss National Research Programme 77 project “Socially acceptable AI and fairness trade‐offs in predictive analytics”.
Her main research areas are Neuroscience of Ethics and Digital Ethics. She is currently working on the ethical issues of digital technologies aiming to improve people’s well-being.
Her latest publication is the book “Moral choices for our future selves” for the series Routledge Focus on Philosophy.
Ethics of algorithmic prediction: How algorithms threaten our right to be unpredictable
Predictive algorithms give an unprecedented support for decision-making in high-stakes domains such as the assignment of medical treatments, jobs, loans, and parole, as well as for everyday choices such as the suggestion of what news to read or movie to watch. Yet, such a support comes at ethical costs concerning privacy, lack of transparency, discrimination, profiling, and choice manipulation. In this talk, first, I will discuss an ethical issue of predictive algorithms involving the essence of being a person in the sense of an agent able to make choices autonomously. This ethical issue is due to an aspect of the design of predictive algorithms: a predictive algorithm treats people as mere members of a group it assigns them to, thus overlooking their uniqueness deriving from being autonomous agents and the possibility that their behavior deviates from the group they are assigned to. Then, I will defend the right to be unpredictable, which requires that we consider the possibility that the subject of an algorithmic decision can be an exception to the algorithm’s prediction, and analyze some decisional contexts in which this right is applicable.
Jérôme Koller
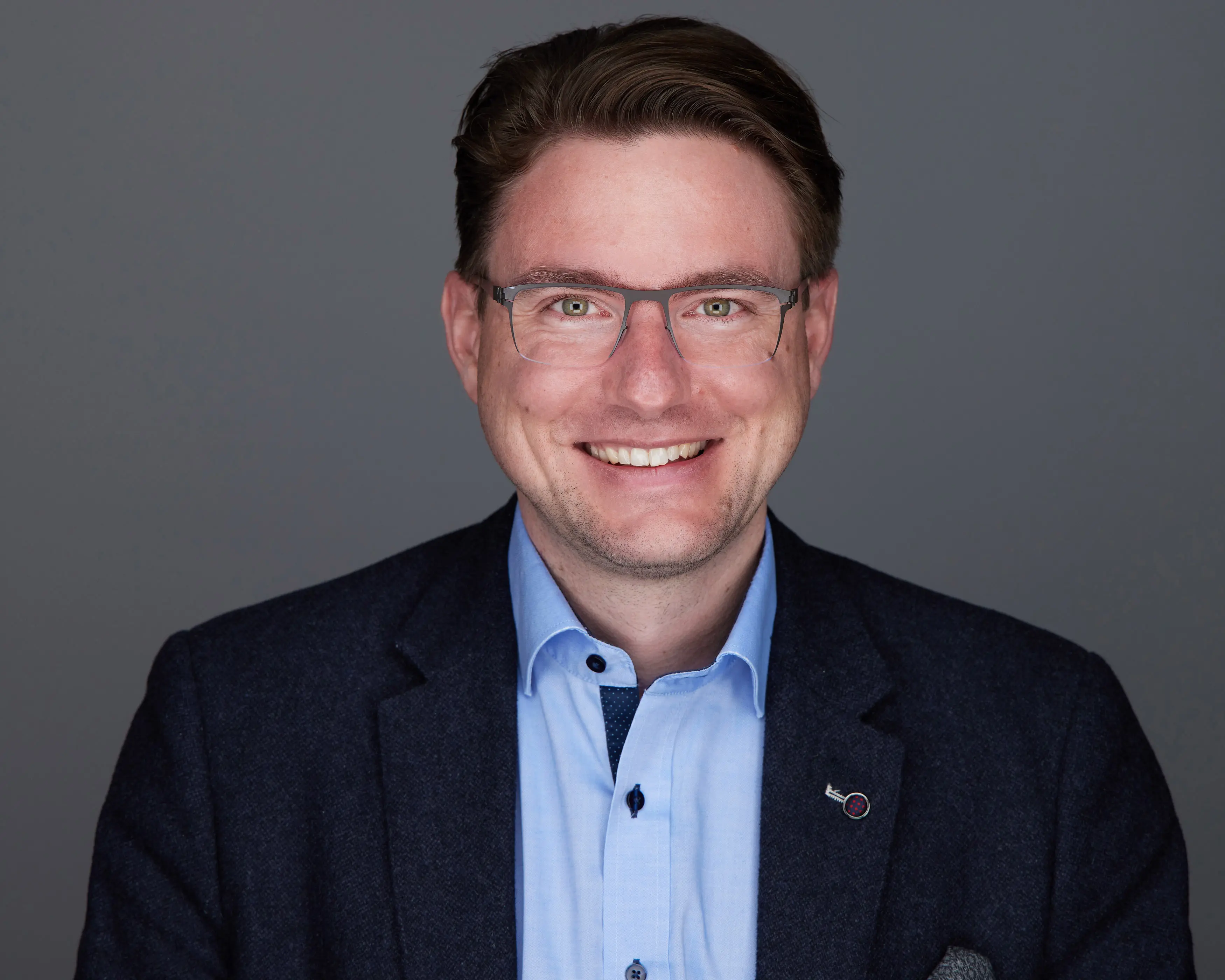
A Brief Biography
Jérôme Koller, Head of Arena Steuerung, La Mobilière
Jérôme is a passionate artificial intelligence professional committed to delivering value from data at scale in the organization. He has built and led a team of 25 scientists implementing projects across La Mobilière and aiming to a broad adoption of AI within the company. Jerome has previously held various positions in the field of data at La Mobilière, from risk management to data warehousing, business intelligence and customer analytics. He currently is in charge for the strategic steering of the agile organization of La Mobilière. He studied mathematics and informatics at the University of Fribourg and completed his EMBA degree at IMD Business School in Lausanne in 2022.
You have your ethical framework, so what?
Many companies are incorporating AI and data-driven solutions in their toolkit to create value for their customers. Using more data usually leads to ethical questions and can raise passionate internal debates about what to do or not. Is the ethical questioning coming from the more innovative side of the company, arguing that you must define such policies today to "stay tuned" with society, or is it a way for the more conservative employees to question the transformation?
At La Mobilière, we defined our ethical framework in 2019 and have been using it since then. I will give an overview of the ethical principles of La Mobilière, explain how we use them, and give my lessons learned from this journey. By focusing on which problems the framework can solve and which ones, in my opinion, are still open, I will highlight some underestimated values of clarifying ethical thoughts in Business.
Andreas Blum, MBA
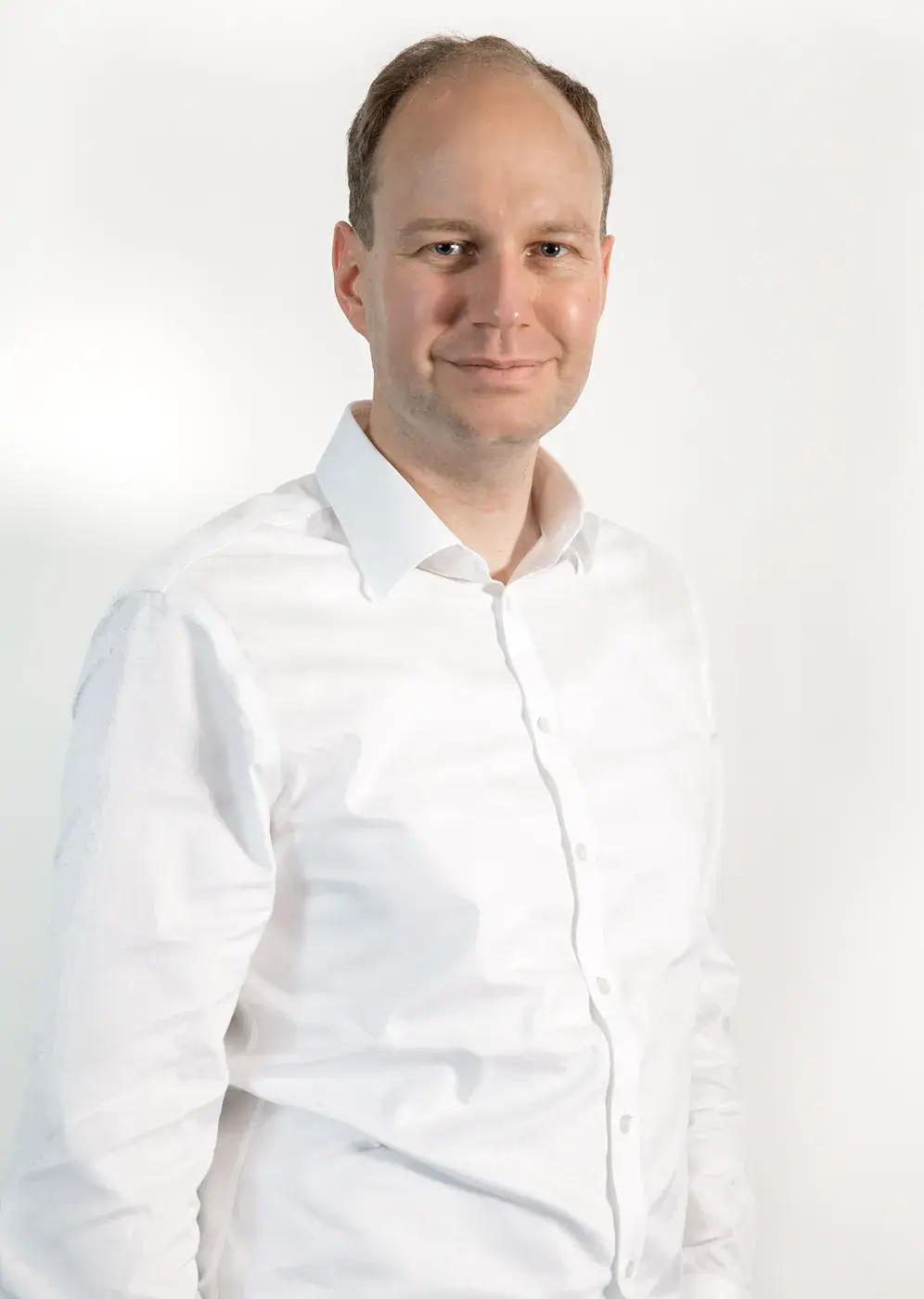
A Brief Biography
Andreas leads the Financial Services Practice and the Digital & AI Consulting Practice of Unit8, a Swiss data & analytics consultancy with focus on both implementation and strategic advisory.
He is a senior strategy consultant with more than 10 years of experience in both external and inhouse management consulting. During this time he conceptualised and implemented strategic initiatives across different industries and functions, in recent years with increasing focus on Digital Transformation and Data & Analytics.
He started his career with the strategy consultancy A.T. Kearney in Zürich and later worked for the strategy & transformation advisor goetzpartners in Germany. Before joining Unit8, he was a project manager within the Strategy & Business Development unit of UBS in Zürich.
He holds an MBA from INSEAD and an MPhil in Innovation & Strategy from Cambridge University.
In his spare time, Andreas likes to ski, explore European cities and run (half-)marathons.
Andreas is based in the Zurich office of Unit8.
How to ensure "Trustworthy AI" from a governance perspective and get ready for the planned EU AI Act, also affecting Swiss entities
Considering the far-reaching impact of AI including potential negative consequences and ethical questions of flawed AI systems, lawmakers across the globe are currently working on regulating its use.
Most noteworthy is the so-called “Artificial Intelligence Act”, a proposal by the European Commission to lay down harmonized rules on the use of AI.
This planned regulation by the European Commission could not only change the way how AI is developed in the EU but could also have profound implications for businesses in Switzerland. It is planned that non-compliance will lead to heavy fines, amounting to up to 6% of global company turnover or EUR 30m (which would be even heftier than fines imposed by the GDPR). It has not yet been decided when and in which exact shape this regulation will come into force, but companies are well advised to already now start ramping up their AI & advanced analytics governance effort to mitigate business, reputational and also compliance risks. This presentation will provide practical guidance on what to expect and how to prepare.
Prof. Patricia Deflorin
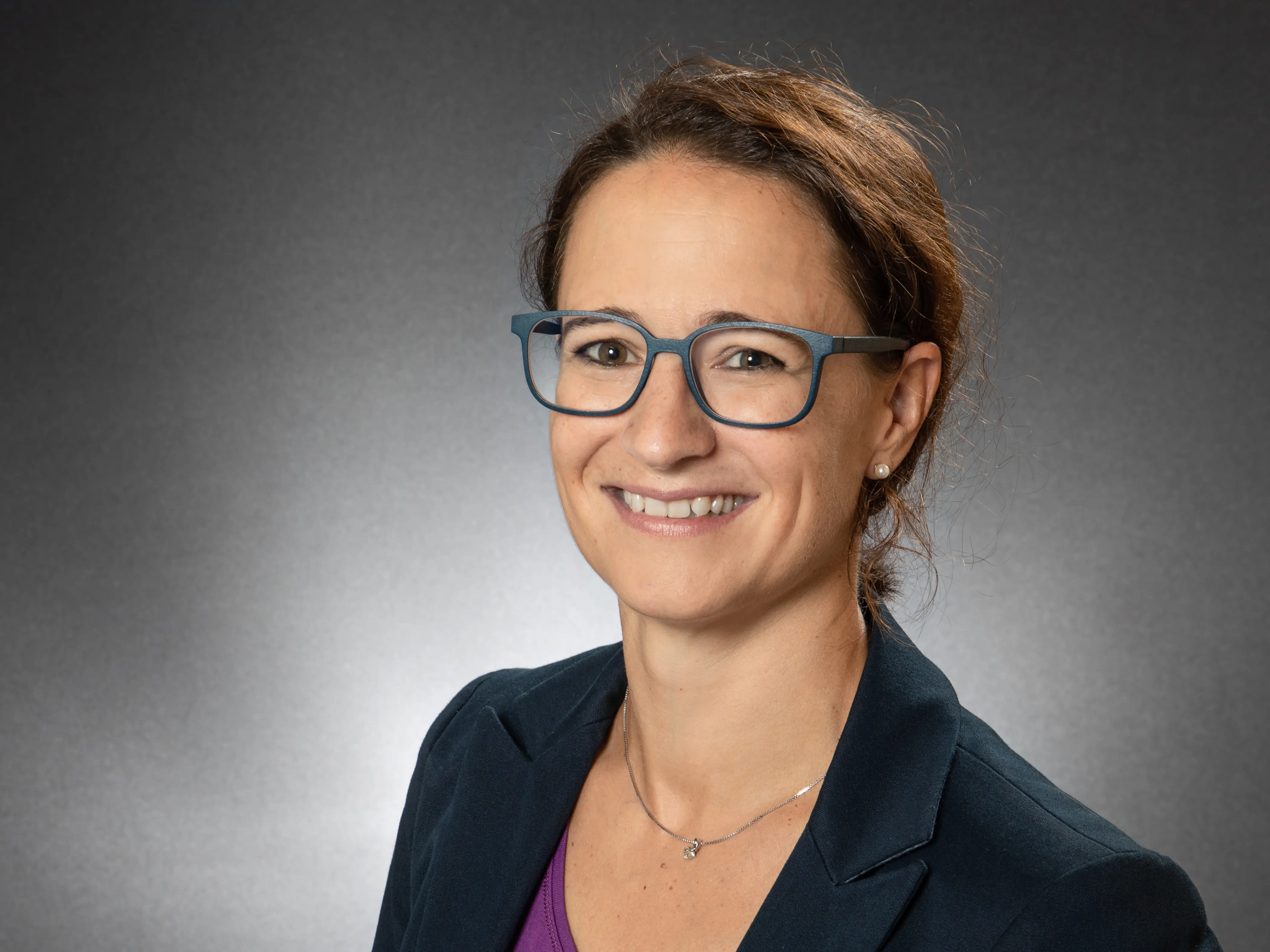
A Brief Biography
Prof. Dr. Patricia Deflorin is Research Director at the Swiss Institute for Entrepreneurship (SIFE), FH Graubünden, and lecturer at the University of Zurich. Within the NTN Innovation Booster Databooster, she is the co-leader of the focus topic Industry 4.0. Her research focus on innovation, supply chain and digital transformation. Her area of experience includes the design and implementation of data-based services, the generation and analysis of IoT business models and the identification of necessary technologies for the successful implementation of digital transformation.
Databooster – Open innovation activities to boost data based innovation
Technologies, data analysis, interfaces and standards. Companies pursuing Industry 4.0 and smart services are facing many decisions on how to pursue their activities. But which investments may lead to a radical innovation? The NTN Innovationbooster Databooster supports companies in their quest to develop and implement databased innovations. Patricia Deflorin shows which topics industrial companies seem as relevant to pursue and how these topics may differ between small and larger companies. In addition, she sheds light into the Databooster activities and how industrial companies can profit from the networks activities.
Dr. Hanspeter Bär
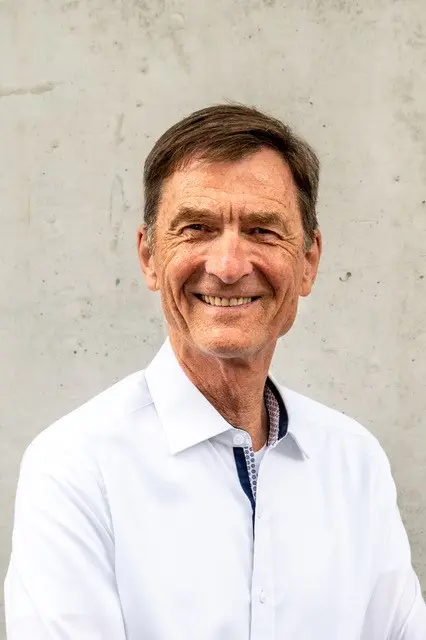
A Brief Biography
Hanspeter Bär's background is in Electrical Engineering (Dr. sc. techn./ETHZ in Communications Technologies) and Business Management. He spent his business life in 2 international Swiss companies (Zellweger Uster and Huber+Suhner), in various positions from Sales Engineer, R&D Manager to Division Manager and Member of the Group Management. Since his retirement, he is active as an Innovation Mentor for Innosuisse. In this role, he has coached more than 300 project teams, supporting them in writing a convincing application for Innosuisse funding of an innovation project.
How to be successful with your Innosuisse Application for innovation project funding
I will present the key instruments Innosuisse is offering for project funding. Furthermore, I will talk about the criteria for a successful application.
Manuel Eicher
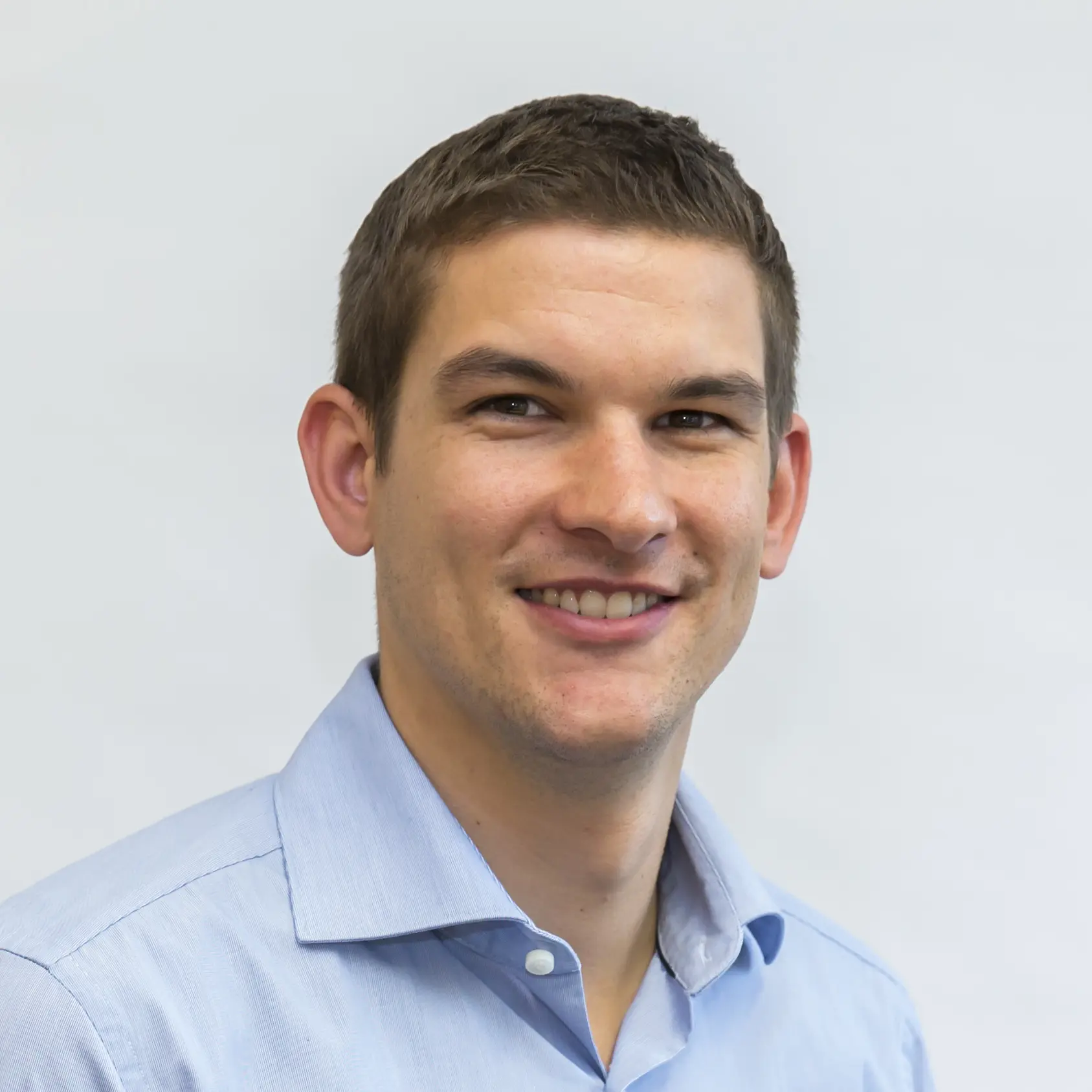
A Brief Biography
Manuel Eicher is in the role as General Manager Geohazard Solutions at Geobrugg, an international company that is a leader in the development and production of protection systems against natural hazards. Manuel learned his profession as a mechanic and further developed his knowledge in Business Processes HF, Sales and Marketing FH in the direction of business management EMBA. In his activities at Geobrugg, he was significantly involved in laying the foundation for a data-based business model in order to develop the company from a purely industrial company to a producing service provider.
Smart protection systems - where do we go from here?
Protection systems against natural hazards are mostly remote and difficult to monitor. In this setting, remote monitoring is a suitable new offering with great potential. In this presentation, it will be shown how Geobrugg identified the opportunity, developed the Geobrugg GUARD and started implementing the new hardware solution. But how does an industrial company deal when it suddenly has a monitoring solution that generates data and has to deal with other customers? The Geobrugg GUARD is used as an example to show how Geobrugg, together with the FH Graubünden, develops a data-based, international and scalable service model for protection against natural hazards in an Innosuisse project.
Dr. Arman Iranfar, Amin Amini
A Brief Biography
Arman Iranfar received his PhD in electrical engineering from EPFL in 2020 with a focus on applied machine learning in embedded systems. He has published over 20 peer-reviewed papers in top-notch conferences and journals and served as a reviewer in several top-ranked conferences and journals including IEEE TC, IEEE TSUSC, and IEEE TSC. He is currently a senior data scientist at CertX, building the first certification scheme for AI trustworthiness.
Amin Amini is leading the functional safety expert of CertX since September 2018. Based on his strong background in telecommunications and automotive industry, he has developed functional safety certification schemes for supporting customers to meet the State-of-the-Art, as well as to demonstrate their best practices to authorities across different market segments. Amin is an ISO 26262, OPST and IEC 61508 certified engineer and expert member of ISO 26262 2nd edition and ISO/PRF PAS 21448 (SOTIF) standardisation group.
certAInty - A Certification Scheme for AI systems
Certification of AI Systems by an accredited body increases trust, accelerates adoption and enables their use for safety-critical applications. Under the Innosuisse umbrella, CertX is developing a Certification Scheme comprising specific requirements, criteria, measures, and technical methods for assessing Machine Learning enabled systems.
Closing panel
Prof. Dr. Peter Schwendner leads the Institute of Wealth & Asset Management at Zurich University of Applied Sciences, School of Management and Law. He collected 15 years of work experience in the financial industry as a head of quantitative research at Sal. Oppenheim and as a partner at Fortinbras Asset Management after he completed a doctorate in physics. He has been developing analytics for primary and secondary markets and quantitative risk premia strategies with financial industry partners.
Jennifer Victoria Scurrell is a PhD candidate at the Center for Security Studies (CSS) at ETH Zurich. In her dissertation, she examines the influence of AI agents on political opinion formation in online social networks using experimental methods and ML. Jennifer is a political scientist by training and holds a MA and a BA from the University of Zurich.
Pius von Däniken is a research assistant at the ZHAW Centre for Artificial Intelligence. As a member of the Natural Language Processing group, he focuses on malicious behavior in Social Media and the dynamics of online radicalization. His other research interests include the evaluation of dialogue systems and the development of NLP tools for Swiss German. He holds a BSc in Computer Science from EPFL and is currently a MSE student at the ZHAW.
Organizing Committee
- Prof. Dr. Patricia Deflorin
- Prof. Dr. Rudolf Füchslin
- Prof. Dr. Branka Hadji Misheva
- Dr. Andreas Henrici
- Prof. Dr. Peter Schwendner
- Prof. Dr. Thilo Stadelmann
- Prof. Dr. Dirk Wilhelm