Improving airspace surveillance with human-machine teams
Air Traffic Management (ATM) calls for pronounced situational awareness and a capacity for rapid reaction. The “AISA” Horizon 2020 SESAR 3 Joint Undertaking Project in which ZHAW is participating aims to determine how the deployment of machine situational awareness can meaningfully support air traffic controllers in their work and provide a basis for moving ahead with automation.
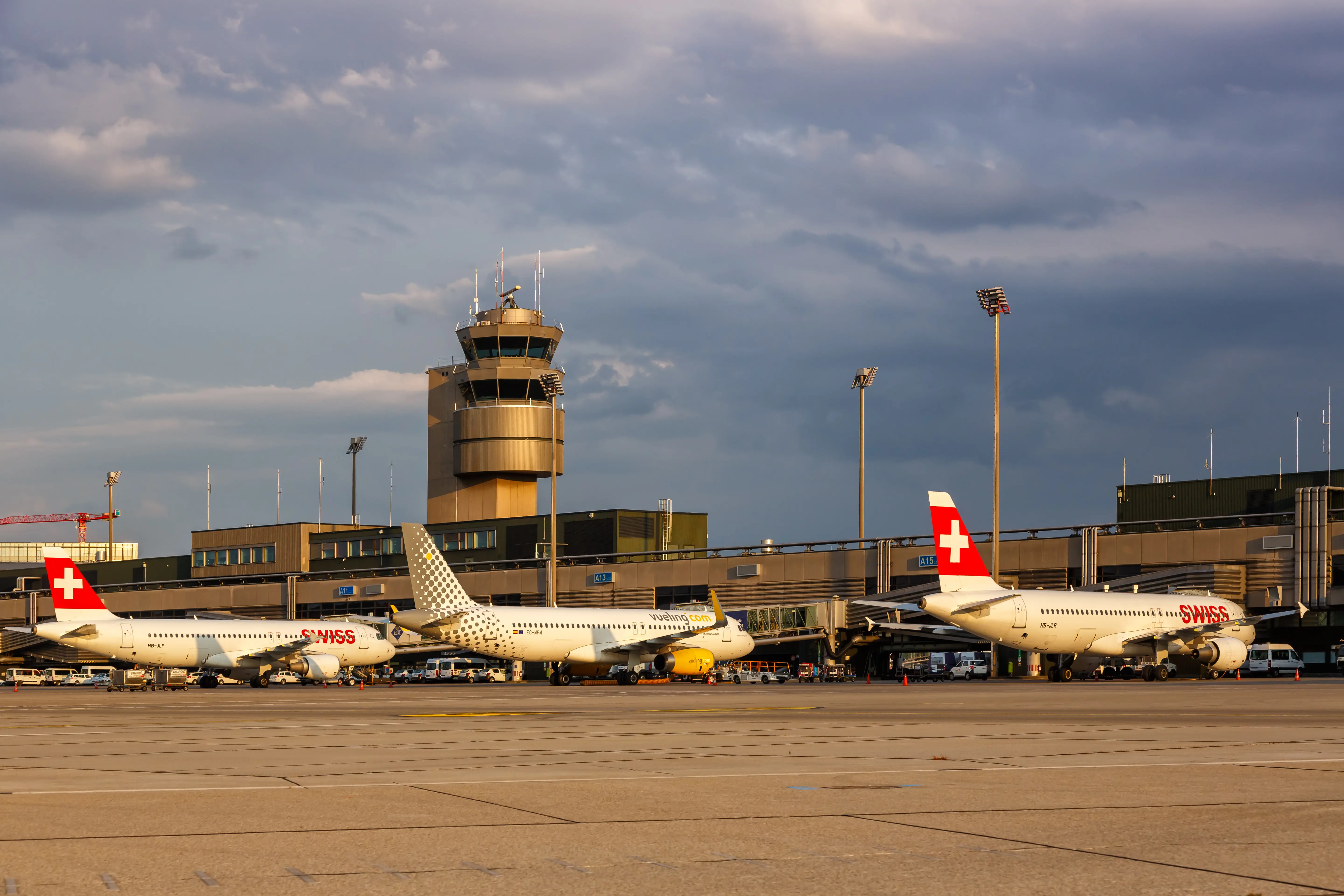
An air traffic controller’s job involves keeping a close eye on air traffic and making sure that flight altitudes and the distances between aircraft are observed. They have to concentrate on the radio and radar at all times. This complex task requires reliable, sustained attention and involves a high level of responsibility.
To assist air traffic controllers in their demanding work, the AISA project (Artificial Situational Awareness Foundation for Advancing Automation) is developing a so-called knowledge graph system. This maps all the knowledge required for the job of air traffic controller and, with the aid of artificial intelligence, generates machine situation awareness. The system is then in a position to monitor air traffic and assist air traffic controllers in maintaining an overview and recognising safety-critical situations at an early stage so that they can be incorporated in the controllers’ decision making. The system will also permit the integration of existing automated tools, such as conflict detection systems. “Our task within the AISA project is to check whether the idea of a knowledge graph system with machine learning modules is feasible in the context of air traffic monitoring. What is of interest in our section of the research is how successfully a joint team situation awareness can be built up with humans and how the air traffic controllers react to this”, explains work package manager Ruth Häusler, who conducts research into human factors at the ZHAW Centre for Aviation (ZAV). The system is designed in such a way that it incorporates three machine learning modules – the conflict detection module, which makes sure that the correct distance is maintained between aircraft, the 4D flight trajectory prediction module and the traffic complexity module. In addition to Ruth Häusler, the project team includes research assistants Celina Vetter, Luca Tensfeldt and Manuel Roth.
Helpful assistant or useless distraction?
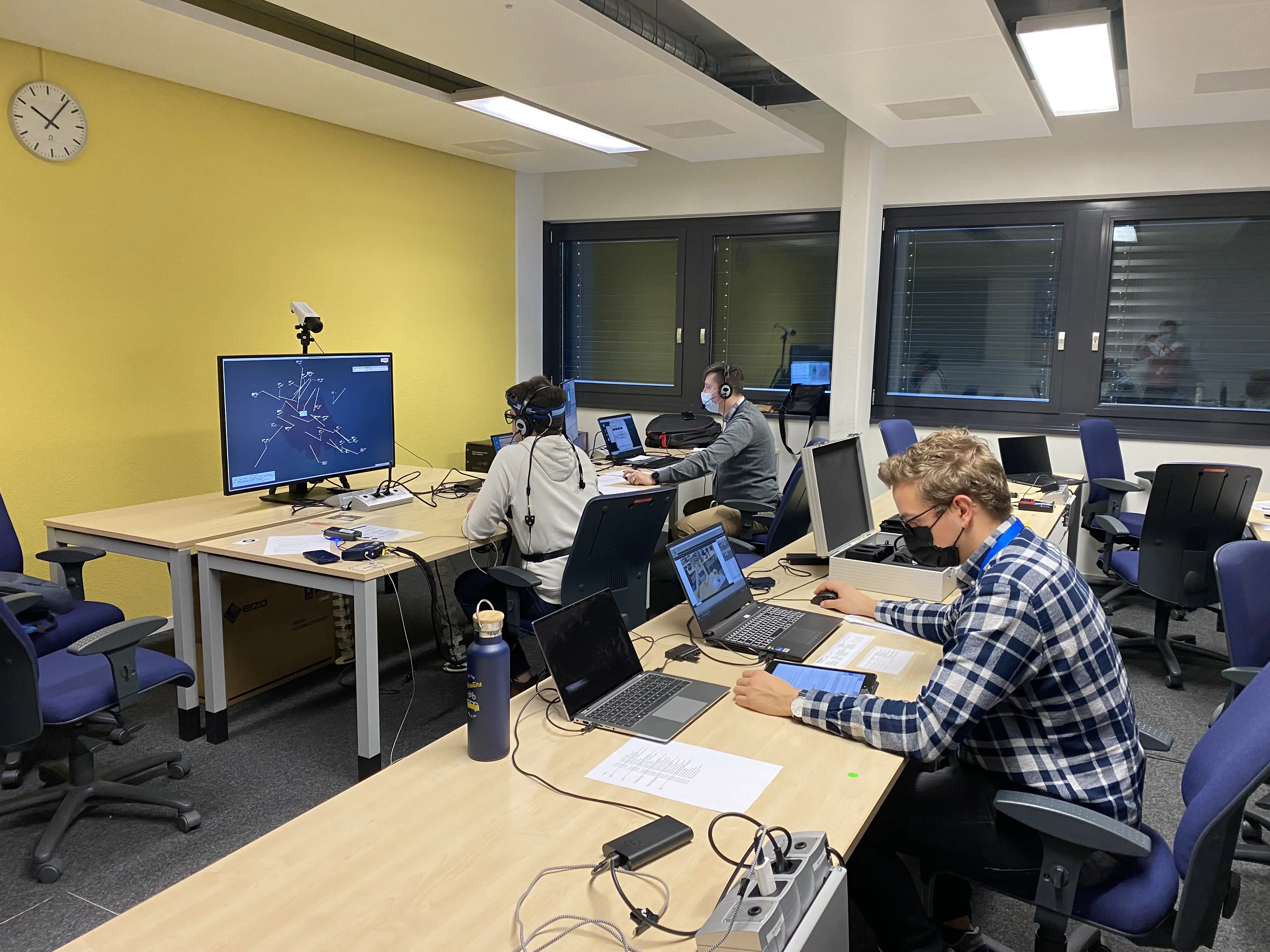
The ZHAW team performed two experiments to test the accuracy of machine situation awareness and to see how it compares with human situation awareness. “In the first step, it was a matter of understanding the way in which air traffic controllers carry out their work”, says Ruth Häusler. In the first experiment, we thus obtained data from the 20 air traffic controllers taking part, showing how they proceed in a number of given situations. A second experiment looked into how another group of air traffic controllers worked when they were assisted by machines. They were also asked about different aspects of a situation. Shortly afterwards, they received the responses generated by the machine situation awareness. “That provided us with a kind of comparison, and the participants received direct feedback on their answers at the same time”, says Ruth Häusler in explaining the effect of the experiment.
No polite restraint from machine colleagues
The results of the experiment showed that when air traffic controllers received feedback from machine situation awareness on specific aspects of a situation, they tended to rate their own situation awareness as somewhat lower. The experimental setup thus improved the air traffic controllers’ monitoring ability. “It’s important to understand that humans are minimalists when it comes to perception. They form a picture of a situation from just a few inputs. A machine, by contrast, does precisely the opposite, systematically and exhaustively sifting through mountains of data. The opportunity and challenge at one and the same time lies in coupling these different systems together to form a team”, Ruth Häusler explains. The system additionally has the advantage that it is not subject to any social norms and has no inhibitions when it comes to questioning or correcting colleagues.
“While we were carrying out the experiments, we were fascinated by how differently the air traffic controllers tackle their work in some cases”, says Ruth Häusler. “Some adopted a calmer approach to conflicts, gathering several items of information and weighting these before passing them on to the pilots in bundled form. Others, by contrast, passed on the information promptly in a number of different communication steps, adapting their approach more frequently. In the final instance, all the controllers achieved safe and efficient air traffic control – the ultimate mission of their job. It was an eye opener for us to see that there are many different methods by which this goal can be reliably achieved.” A combination of recordings of task performance with measurements from eye trackers and psychophysiological sensors could permit further interesting analyses of human performance and improve communication between humans and machines. A comprehensive data source of this kind holds great potential for further advancing machine learning and thus improving coordination between humans and machines in future.
The technology works but needs more data
In a subsequent step, researchers from Zagreb University assessed how reliably machine situational awareness operates. At all events, it has been shown that the technology deployed actually works. “The machine learning modules need to be further refined and trained with more data. They have to learn which information is important, at what times, for air traffic controllers and which information is not important”, Ruth Häusler explains. The system also needs to be optimised to ensure that it can communicate meaningfully with the air traffic controllers without constituting a needless distraction. In doing so, it will by no means replace the work of the air traffic controllers but aims to relieve the pressure on them in a meaningful manner so that, together, they can better manage large volumes of traffic.
There is still a long way to go before teamwork between air traffic controllers and machine situation awareness becomes a reality. The technical capabilities for this are already in place, however.