Intelligent operation of solar power plants: predictive maintenance using a hybrid AI model
As part of an Innosuisse project, researchers from the Institute of Data Analysis and Process Design (IDP) have developed a software module that combines existing knowledge with artificial intelligence (physics-informed AI). The performance analyses based upon it help to minimise energy losses of solar power plants and indicate when maintenance of the PV systems is worthwhile.
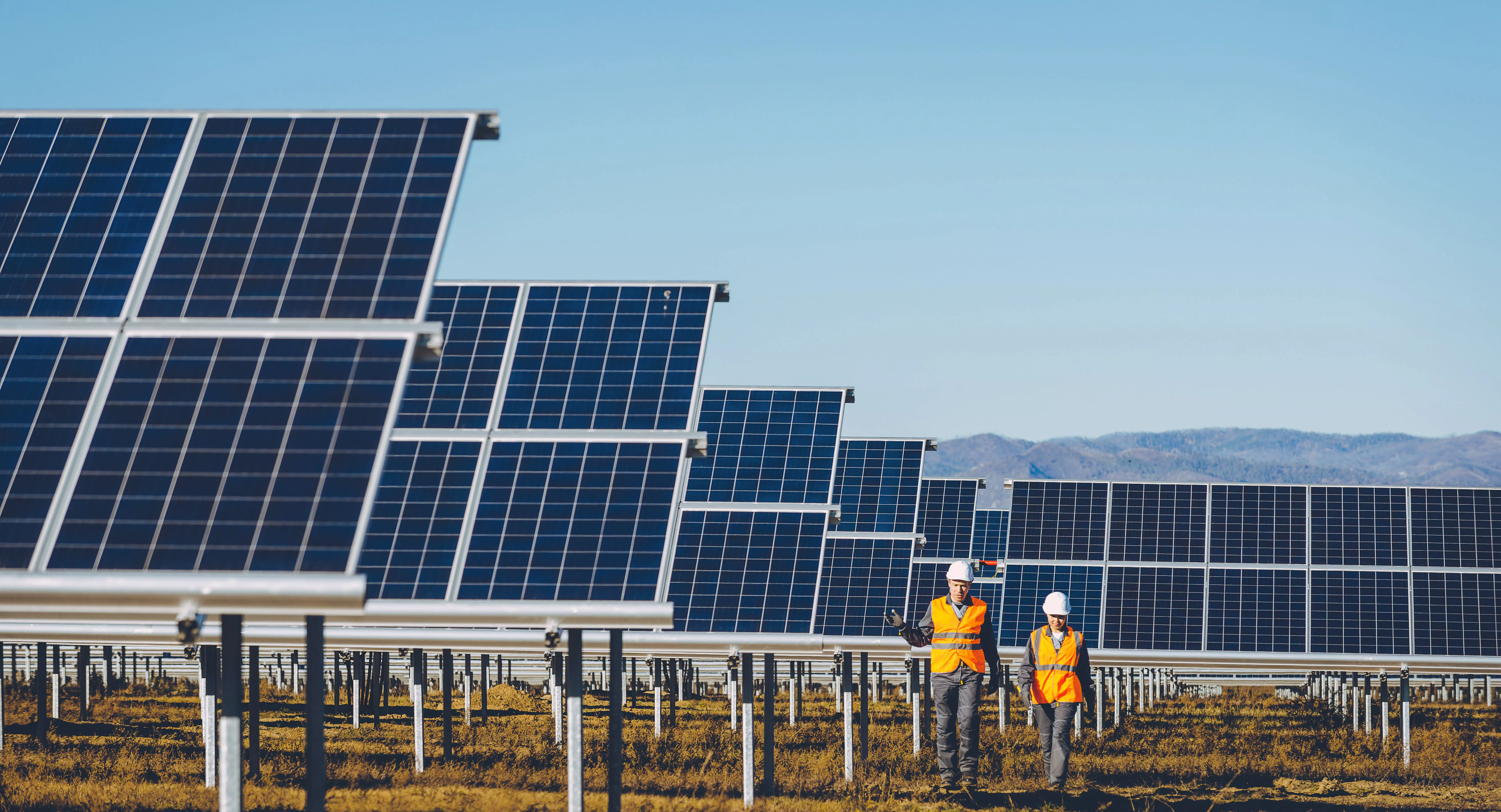
There can be a number of reasons for performance losses in solar power plants. Generally speaking, soiling on the modules, shading of individual areas, electronic failures, inverter faults or faulty tracking can give rise to significant energy losses or even a complete loss of production. Working together with Fluence Energy (formerly known as Nispera), a ZHAW team headed up by Lilach Goren Huber from the Smart Services and Maintenance Research Group at the ZHAW Institute of Data Analysis and Process Design (IDP) have developed a software module for optimising existing photovoltaic (PV) systems. Their program is able to diagnose energy losses suffered by a PV power plant as a result of electronic failures, tracker errors or soiled PV modules and can thus recognise at an early stage when an intervention in the system is required. Specifically, this means that, in an initial step, the program automatically identifies energy loss events owing to a number of causes. In a second step, the program can then decide when maintenance of the system in question will prove worthwhile, in other words when the costs relating to the energy loss are greater than the costs that will be incurred due to the repair itself. “Our challenge was to develop a software module that generates its estimates using data-driven AI, while also incorporating the broad specialist knowledge about the plant to make decisions that are also comprehensible to the engineers responsible for operating the plant,” explains project leader Lilach Goren Huber.
Cost savings thanks to a hybrid AI model
Drawing on historical data that is already available as a result of operating a solar power plant as well as specialist knowledge about the facility, the ZHAW researchers put together a neural network for intelligent fault diagnosis that can be integrated into the existing Nispera platform. In order for the neural network to perform reliably and intelligently, it needs a lot of data relating to faults, such as tracker errors. However, such events appear very rarely in the inventory data. With the help of the knowledge of the physical laws of the plant and the specialist knowledge of the engineers working on it, the team was able to corrupt the real data and thus create artificially “falsified data” containing realistic fault patterns with which the algorithms could be trained.
The researchers were thus able to develop a reliable software module without the need for elaborately generated training data by applying the approach fittingly known as physics-informed AI. This type of predictive maintenance also represents a novelty in terms of the maintenance of PV power plants. “By combining valuable specialist knowledge and a data-driven deep learning model, the module is able to identify faults in the system at an early stage and more accurately than was possible with previous approaches,” says Goren Huber in summarising the program’s advantages. “The ability to detect tracker errors has been improved by 70 percent relative to conventional AI models, meaning that the software module can contribute to reducing energy losses and ensuring the more cost-efficient maintenance of solar power plants,” adds the ZHAW researcher.
Publications relating to the Intelligent Diagnostics of Performance Degradation in Solar Power Plants project:
- Zgraggen, J., Guo, Y., Notaristefano, A. and Goren Huber, L., 2022, October. Physics informed deep learning for tracker fault detection in photovoltaic power plants. In 14th Annual Conference of the Prognostics and Health Management Society, Nashville, USA, 1-4 November 2022 (Vol. 14, No. 1). PHM Society.
- Zgraggen, J., Guo, Y., Notaristefano, A. and Goren Huber, L., 2023. Fully unsupervised fault detection in solar power plants using physics-informed deep learning. In 33rd European Safety and Reliability Conference (ESREL), Southampton, United Kingdom, 3-7 September 2023 (pp. 1737-1745). Research Publishing.
- Goren Huber, L., Palmé, T. and Chao, M.A., 2023, June. Physics-informed machine learning for predictive maintenance: applied use-cases. In 2023 10th IEEE Swiss Conference on Data Science (SDS) (pp. 66-72). IEEE.