Game Over? Experts panel at CAI discusses whether the current approach to AI is a dead end
Experts discussed whether we are at the verge of achieving artificial general intelligence in the second panel of the series "Pathways beyond present AI". They provided Insights on the differences between natural and machine intelligence, and research lines that can help us understand the former and improve the latter.
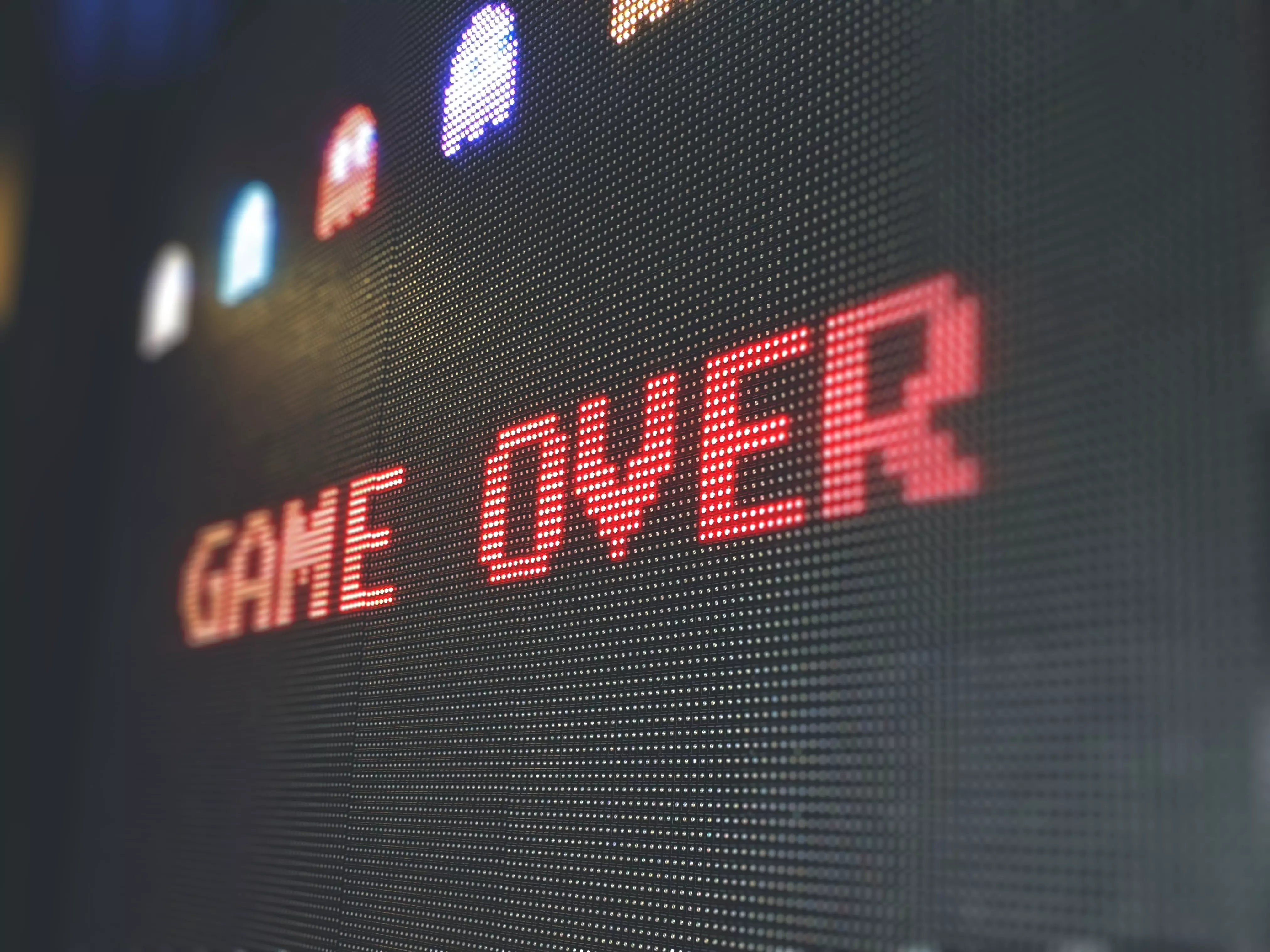
"The game is over" claimed triumphally a recent tweet announcing the release of "Gato", a generalist AI approach aimed at performing hundreds of different tasks. According to the announcement, artificial general intelligence (AGI) was now at reach and the key to achieve it laid on continuing to scale up current systems: i.e., using larger datasets and networks. Similar claims are now more and more common, based on sweeping success stories in the last years (Go Zero, BERT, GPT-3, DALL-E, LaMDA, Stable Diffusion). They purport the vision that access to a massive amounts of data and computing power is the direct way to achieve truly intelligent systems, with little role for further theoretical or formal research on the topic.
Not everybody shares this vision. Voices from academia and industry argue that further foundational research on artificial intelligence is needed (see here and here). The second installment of the ZHAW Center for Artificial Intelligence series of interdisciplinary panels on "Pathways beyond Present AI" focused on this topic. Panellists discussed whether the game was actually over and if not, what could be the missing elements and the roadmap towards AGI.
The panel took place on July 20th 2022, gathering the following panellists: Prof. Dr. Christoph von der Malsburg (Senior Fellow at the Frankfurt Institute for Advanced Studies, and guest professor at UZH/ETH and ZHAW), Prof. em. Dr. Rodney Douglas(UZH/ETHZ, Neuroinformatics), Prof Dr. Thilo Stadelmann (ZHAW CAI), Prof. Dr. Rico Sennrich (Natural Language Processing, UZH), Dr. Yulia Sandamirskaya (Neuromorphic Computing, Intel Labs, Munich, Germany), and Prof Dr. Benjamin Grewe (UZH/ETHZ). The panel was moderated by ZHAW’s Dr. Ricardo Chavarriaga, head of the CLAIRE office Switzerland.
Prof von der Malsburg pointed to the differences between machine and natural intelligence, noting that the learning environment of AI is composed by streams of human-provided samples while natural intelligence relies on self-driven exploration. Additionally, natural intelligence serves the pursuit of innate goals. This last point was supported by Prof. Grewe. He argued that a characteristic of intelligence is the ability of self-generating goals, a vision that was discussed in the first panel of this series.
Panellists also addressed ways towards AGI. Prof Stadelmann argued that achieving AGI may not intrinsically need to be related to understanding natural intelligence. As pointed out by Prof. Sennrich, we may not need self-awareness in systems devoted to solving some specific tasks. Nonetheless, panelists agreed that cross-fertilization of these fields can significantly benefit each other. Dr. Sandamirskaya reminded the importance of research and stated that the field may benefit from supporting not only large-scale "flagship" projects but numerous smaller projects allowing to diversify the objects of study and try multiple approaches. She proposed as an alternative to generalist models, the idea of starting from simple models, like insect brains, and evolve to be able to solve more complex tasks.
Regarding the differences between machine and natural intelligence, Prof Douglas challenged the common conception that the initial states of AI systems is "tabula rasa", clarifying that AI systems already encode some knowledge by the choice of architectures, and computational machinery. Interestingly, the role of the network architecture and learning biases is an important element of the "Theory of Natural Intelligence" advanced by Profs von der Malsburg, Stadelmann and Grewe. However, how does this theory maps into modern deep learning architectures is still an open question.
Besides the architectures, panellists also mentioned the importance of the learning rules. They stated that AI's reliance on backpropagation enforces consistency between the network output and the teacher's expected values. In contrast, bio-inspired self-organizing networks can potentially promote consistency in all levels, across connections and sensory inputs. Consistency over time and modalities was deemed of particular importance, highlighting the need for AI systems to interact with open environments, instead of treating their inputs as independent samples.
The depth and richness of the panel makes it clear that the game is far from being over and there exist yet multiple gaps that need further research to achieve AGI (or any considerable advance in AI systems towards “common sense”). The consensus was that only scaling up current systems is not likely to be the solution. The discussion led to several research directions that could provide insights into how to improve current artificially intelligent systems and get a better grasp on the principles that rule natural intelligence.
More specific aspects of these directions will continue to be discussed in future panels. Please be attentive to the CAI colloquium webpage and our news page for further announcements on the panel series: "Pathways beyond Present AI".